Improving intrusion detection in SCADA systems using stacking ensemble of tree-based models
Bulletin of Electrical Engineering and Informatics(2022)
Abstract
This paper introduces a stacking ensemble model, which combines three single models, to improve intrusion detection in supervisory control and data acquisition (SCADA) systems. The first layer of the proposed model is the combination of random forest, light boosting gradient machine, and eXtreme gradient boosting models. We use an multilayer perceptron (MLP) network as a meta-classifier of the model. The proposed model is optimized and tested on an international dataset (gas pipeline dataset). The tested results show an accuracy of 99.72% with the f1-score of 99.72% for binary classification tasks (attacked or non-attacked detection). For categorical tasks, the detection rates of almost all attack types are higher than 97.55% (except for denial of service (DoS)-95.17%), with an overall accuracy of 99.62%.
MoreTranslated text
Key words
scada systems,intrusion detection,ensemble,stacking,tree-based
AI Read Science
Must-Reading Tree
Example
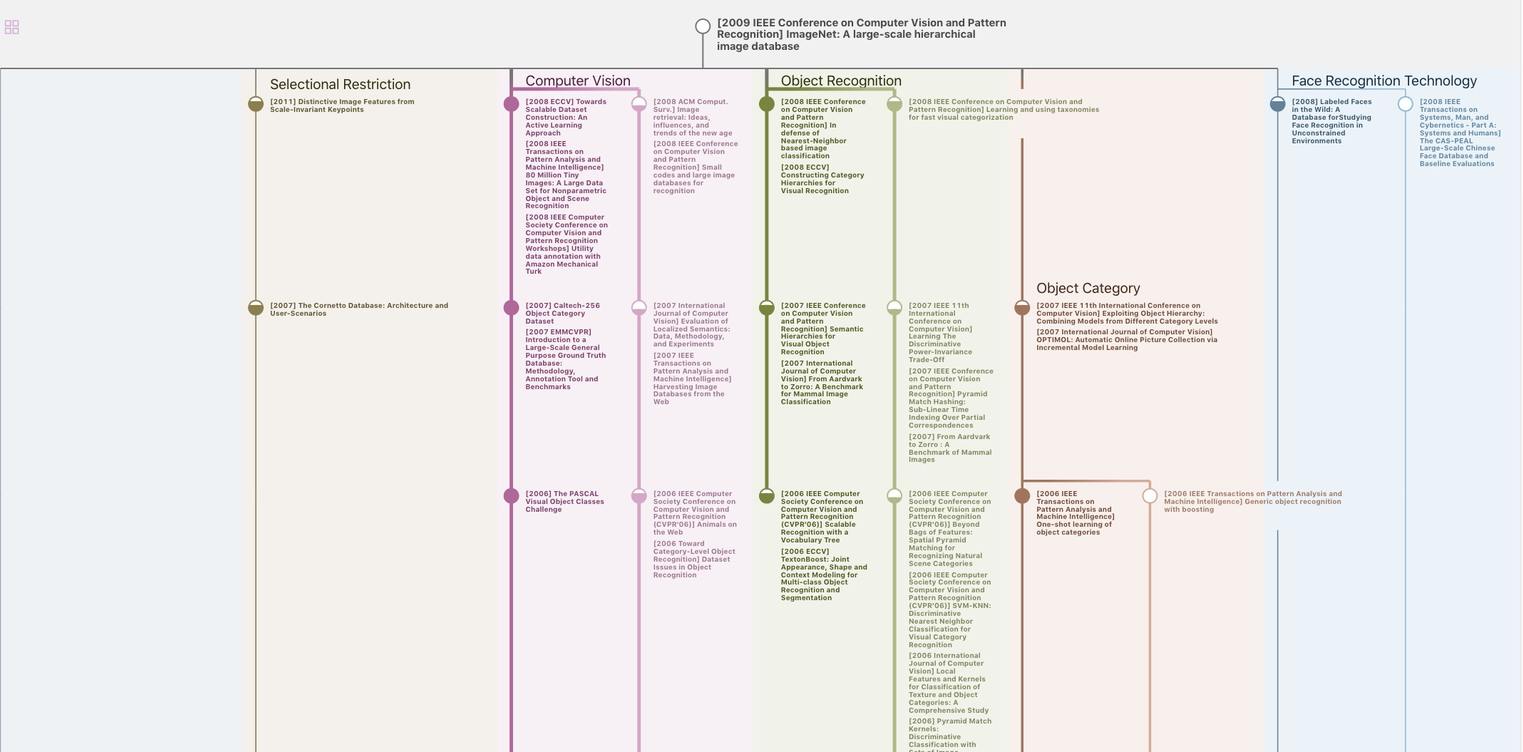
Generate MRT to find the research sequence of this paper
Chat Paper
Summary is being generated by the instructions you defined