Phenotyping multiple maize ear traits from a single image: Kernels per ear, rows per ear, and kernels per row*
COMPUTERS AND ELECTRONICS IN AGRICULTURE(2022)
摘要
Certain traits of maize ears, such as kernels per ear, kernels per row, and rows per ear, are highly related with the grain yield and stress resistance. They can be used as quantitative indices for selecting superior varieties and analyzing experimental results in maize breeding. However, these traits are often manually obtained, which is laborious and prone to error. Image-based object counting methods can replace the manual counting procedure safely, which is fast and accurate. Although a constellation of methods have been proposed, problems remain: i) classic image processing-based methods are not robust to variations of illuminations and resolutions; ii) deep learning-based object counting methods can only predict the total kernels per ear. To address this, we propose a high-throughput maize ear phenotyping pipeline. From a single image, our pipeline estimates kernels per ear, rows per ear, and kernels per row in an interpretable way. The image is first input into a Maize Kernel Counting Network (MKNet), which outputs the estimated kernel density map. Then, receiving the density map as input, a Row Mask Generator (RMG) generates a series of masks; each covers a ear row. Naturally, rows per ear and kernels per row can be recovered from the masks. Extensive experiments show that our method can predict kernels per ear, rows per ear, and kernels per row precisely, with a mean absolute error of 7.48, 0.32 and 1.07, respectively. The results show that, compared with existing object counting methods, MKNet can not only reduce the counting error on kernels per ear, but also produce more accurate localization of maize kernels. The visualizations also demonstrate that RMG is robust to the irregular shapes of ear rows.
更多查看译文
关键词
Maize kernel counting,Convolutional neural network,Kernels per row,Rows per ear,Deep learning
AI 理解论文
溯源树
样例
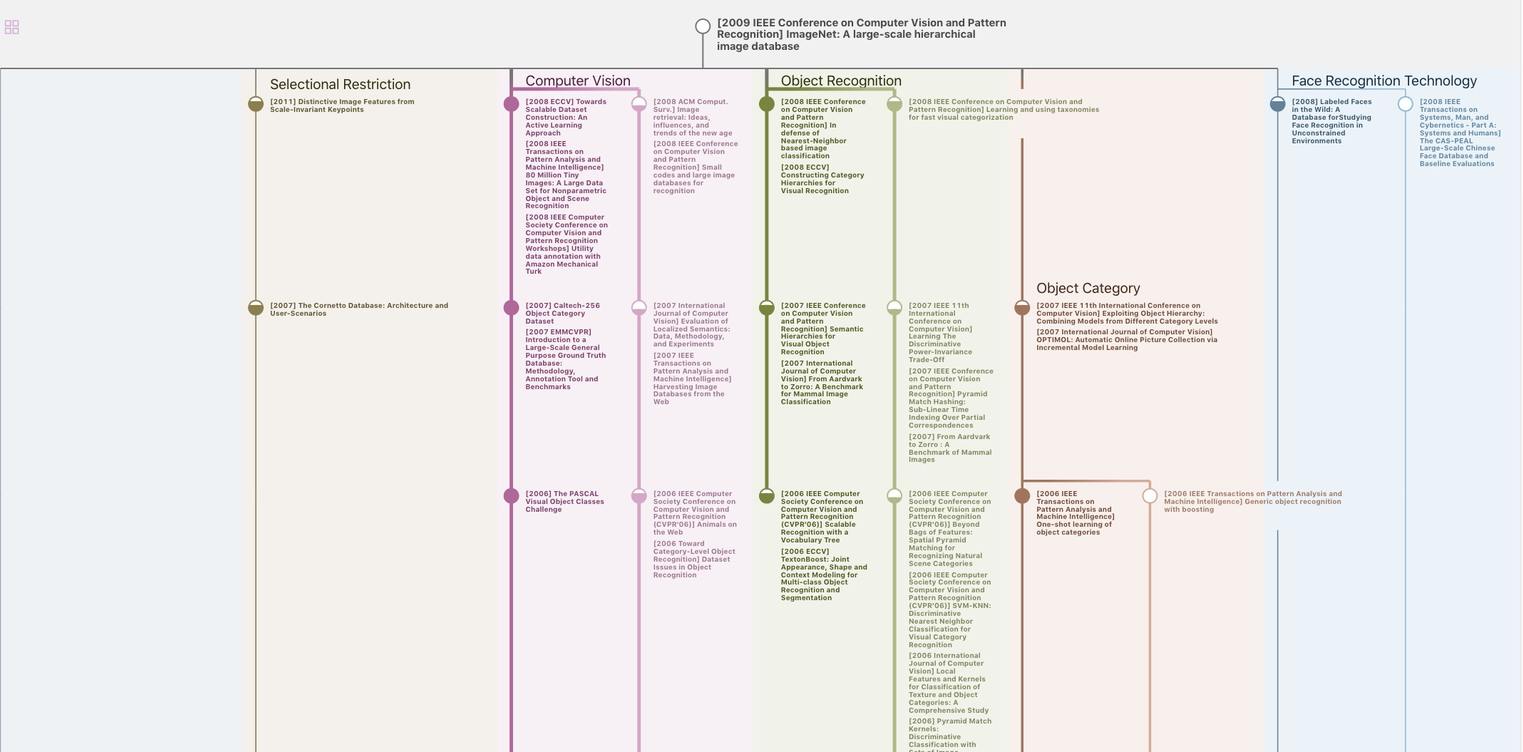
生成溯源树,研究论文发展脉络
Chat Paper
正在生成论文摘要