Dynamic Ridesharing With Minimal Regret: Towards an Enhanced Engagement Among Three Stakeholders
IEEE Transactions on Knowledge and Data Engineering(2023)
摘要
In dynamic ridesharing, the platform serves as the mediator by tailoring the assignment result between workers and riders with a focus on a certain objective. Existing studies generally focus on either one or two stakeholders when modelling the problem while the wellbeing of the other parties may be ignored or even undermined. For example, purely maximizing the total revenue of the ridesharing platform may cause the loss of riders and in turn lead to a low served rate, because those expensive orders will be processed in priority. In this paper, we for the first time study how to incorporate the willingness of all stakeholders (i.e., the platform, workers and riders). Given a set of workers and a set of rider requests, we aim to return the matchable worker-rider pairs in order to minimize the regret. Specifically, two types of regret are defined: (i) the served rate regret, which refers to the rate of unserved requests, catering for the reputation and profit of the platform and workers; (ii) the revenue regret, which considers the portion of revenue loss from unserved riders, catering for the focus of workers and riders in the trip schedule. We prove the NP-hardness of this problem. To tackle this problem, we first propose a dynamic programming insertion algorithm to improve the efficiency of inserting a rider request into a trip schedule of a worker. Furthermore, two kinds of heuristic algorithms are devised to match rider requests with workers effectively. Comprehensive experiments on two real-world datasets verify the effectiveness, efficiency and scalability of our solutions in dealing with different supply-demand relationships in practice.
更多查看译文
关键词
Stakeholders,Heuristic algorithms,Schedules,Costs,Loss measurement,Dynamic programming,Vehicle dynamics,Dynamic ridesharing,served rate regret,revenue regret
AI 理解论文
溯源树
样例
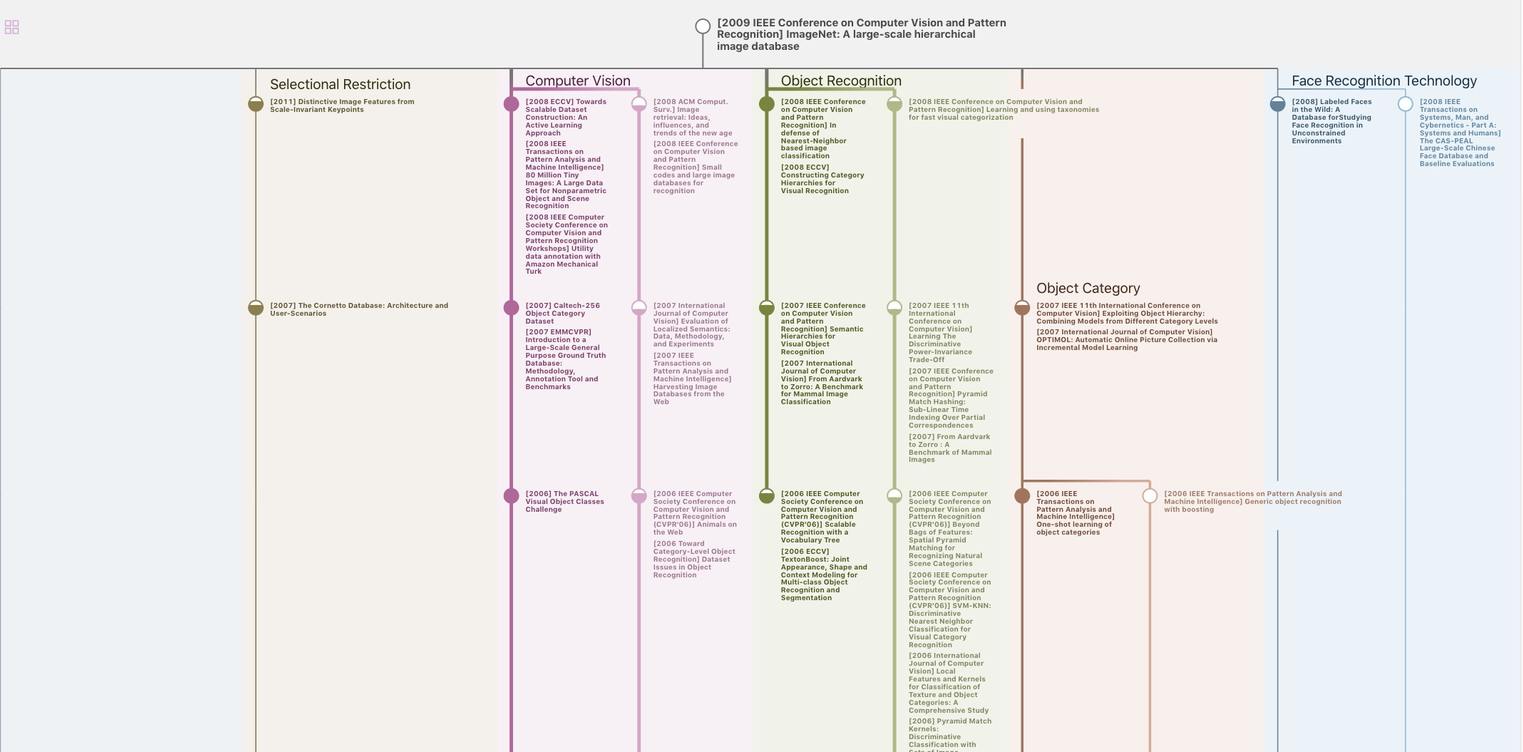
生成溯源树,研究论文发展脉络
Chat Paper
正在生成论文摘要