Structured graph based image regression for unsupervised multimodal change detection
ISPRS Journal of Photogrammetry and Remote Sensing(2022)
摘要
Change detection for multimodal remote sensing images is an important and challenging research topic with a wide range of applications in disaster assessment and environmental monitoring. To address the problem that heterogeneous images cannot be directly compared due to different imaging mechanisms, we propose an unsupervised image regression method based on the inherent structure consistency between heterogeneous images, which learns a structured graph and computes the regression image by graph projection. Firstly, the proposed method uses the self-expression property to preserve the global structure of image and uses the adaptive neighbor approach to capture the local structure of image in the graph learning process. Then, with the learned graph, two types of structure constraints are introduced into the regression model: one corresponds to the global self-expression constraint and the other corresponds to the local similarity constraint, which can be further implemented by using graph or hypergraph Laplacian based regularization. Finally, a Markov segmentation model is designed to calculate the binary change map, which combines the change information and spatial information to improve the detection accuracy. Experiments conducted on six real data sets show the effectiveness of the proposed method by comparing with five state-of-the-art algorithms, achieving 2.4%, 5.5% and 4.1% improvements in accuracy, Kappa coefficient, and F1 score respectively. Source code of the proposed method will be made available at https://github.com/yulisun/GIR-MRF.
更多查看译文
关键词
Unsupervised change detection,Structured graph,Hypergraph,Image regression,Multimodal,Markov random field
AI 理解论文
溯源树
样例
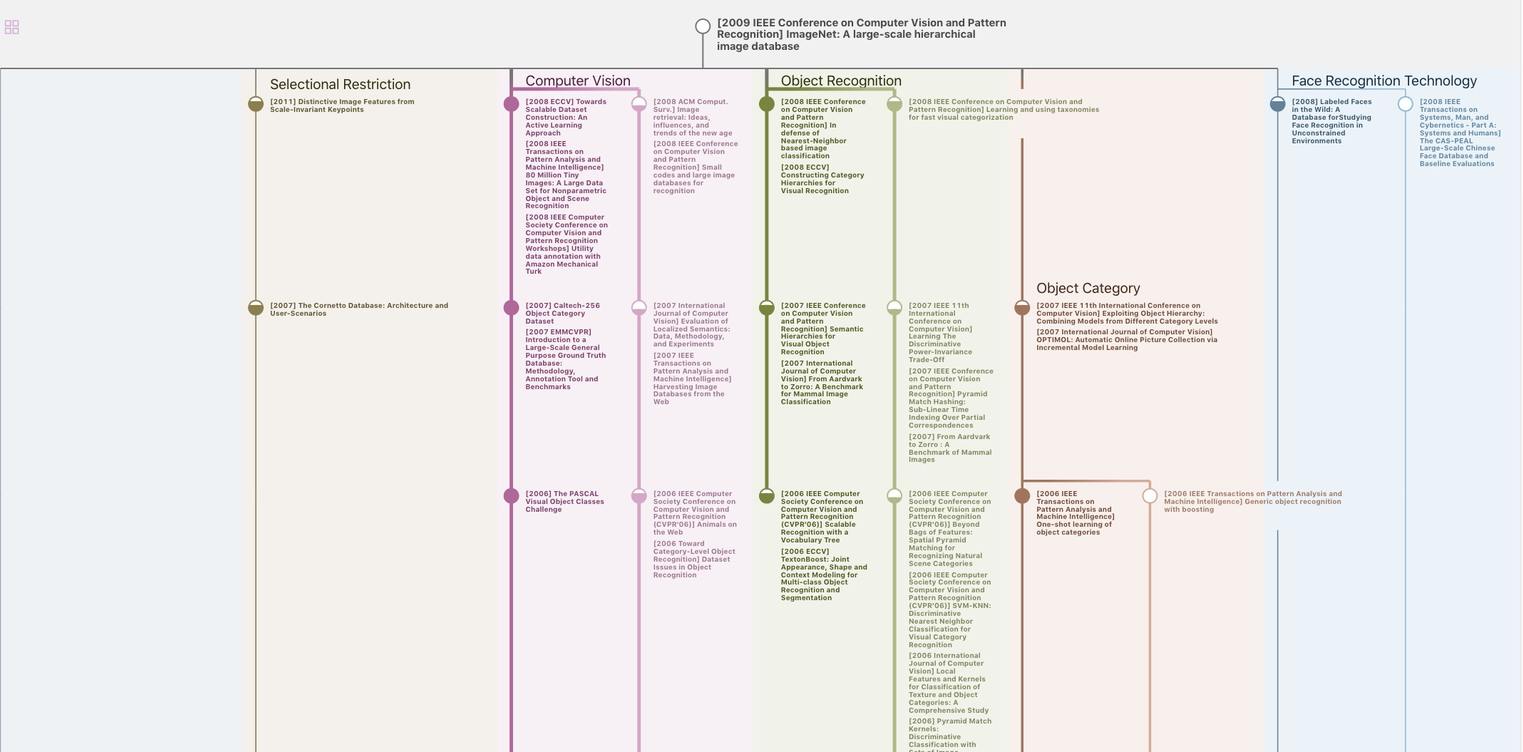
生成溯源树,研究论文发展脉络
Chat Paper
正在生成论文摘要