Self-supervised Visual Feature Learning for Polyp Segmentation in Colonoscopy Images Using Image Reconstruction as Pretext Task
2021 8th NAFOSTED Conference on Information and Computer Science (NICS)(2021)
摘要
Automatic polyp detection and segmentation are desirable for colon screening because the polyps miss rate in clinical practice is relatively high. The deep learning-based approach for polyp segmentation has gained much attention in recent years due to the automatic feature extraction process to segment polyp regions with unprecedented precision. However, training these networks requires a large amount of manually annotated data, which is limited by the available resources of endoscopic doctors. We propose a self-supervised visual learning method for polyp segmentation to address this challenge. We adapted self-supervised visual feature learning with image reconstruction as a pretext task and polyp segmentation as a downstream task. UNet is used as the backbone architecture for both the pretext task and the downstream task. The unlabeled colonoscopy image dataset is used to train the pretext network. For polyp segmentation, we apply transfer learning on the pretext network. The polyp segmentation network is trained using a public benchmark dataset for polyp segmentation. Our experiments demonstrate that the proposed self-supervised learning method can achieve a better segmentation accuracy than an UNet trained from scratch. On the CVC-ColonDB polyp segmentation dataset with only annotated 300 images, the proposed method improves IoU metric from 76.87% to 81.99% and Dice metric from 86.61% to 89.33% for polyp segmentation, compared to the baseline UNet.
更多查看译文
关键词
Polyp segmentation,medical image analysis,transfer learning,deep learning,self-supervised learning
AI 理解论文
溯源树
样例
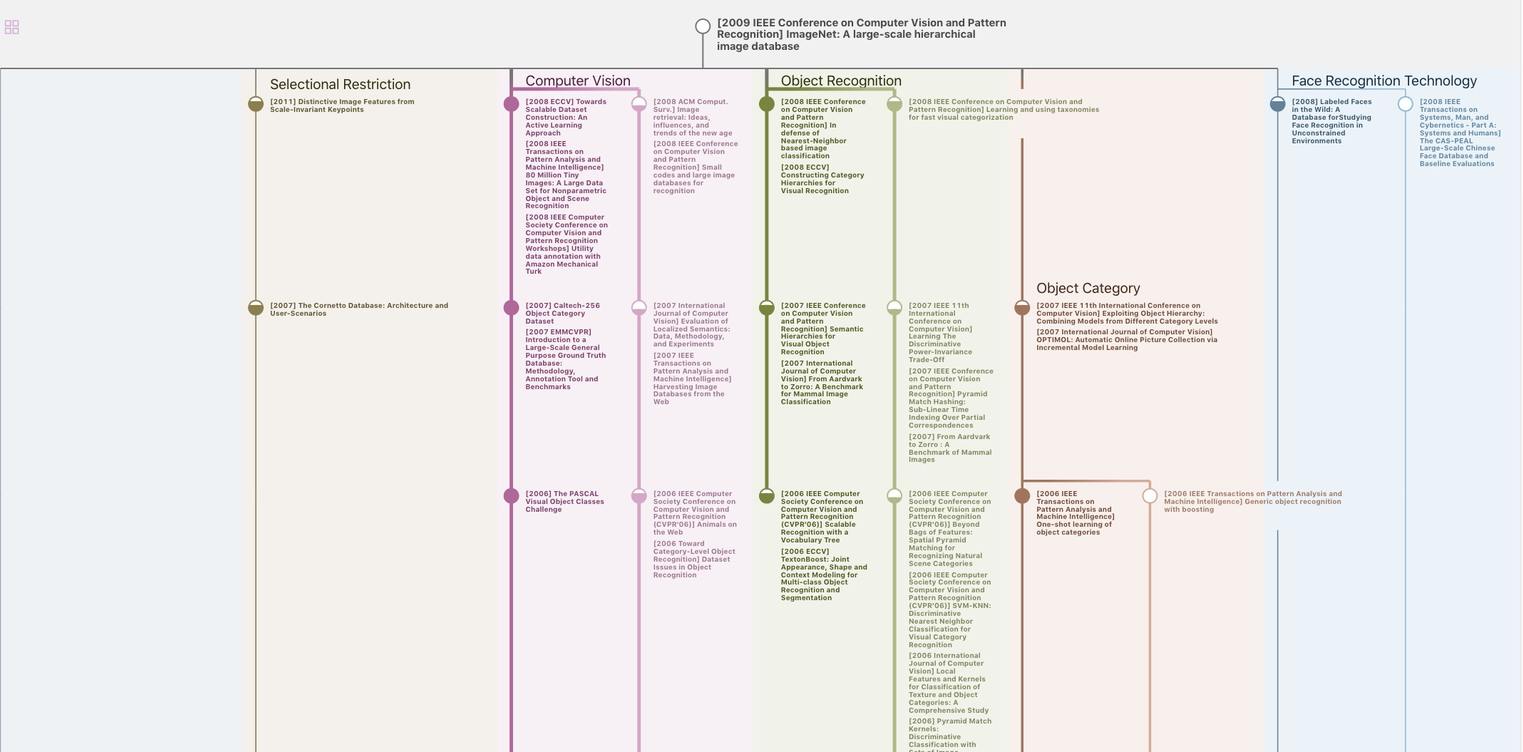
生成溯源树,研究论文发展脉络
Chat Paper
正在生成论文摘要