UAC: Offline Reinforcement Learning With Uncertain Action Constraint
IEEE TRANSACTIONS ON COGNITIVE AND DEVELOPMENTAL SYSTEMS(2024)
摘要
Offline reinforcement learning (RL) algorithms promise to learn policies directly from offline data sets without environmental interaction. This arrangement enables successful RL applications in the real world, particularly in robots and autonomous driving, where sampling is costly and dangerous. However, the existing offline RL algorithms suffer from insufficient performance attributed to extrapolation error caused by out-of-distribution (OOD) actions. In this work, we propose an offline RL algorithm with an uncertain action constraint (UAC). The design principle of UAC is to minimize the extrapolation error via eliminating unknown and uncertain actions. Concretely, we first theoretically analyze the effects of different types of actions on the extrapolation error. Based on this, we propose an action-constrained strategy that exploits the uncertainty of the environmental dynamics model to eliminate unknown and uncertain actions in the Q -value evaluation process. Furthermore, the convex combination of trajectory information and Gaussian noise is novelly leveraged to enhance the generation probability of the optimal actions. Finally, we carry out the comparison and ablation experiments on the standard D4RL data set. Experimental results indicate that UAC achieves competitive performance, especially in the field of robotic manipulation.
更多查看译文
关键词
Offline reinforcement learning (RL),out-of distribution (OOD) actions,uncertain actions
AI 理解论文
溯源树
样例
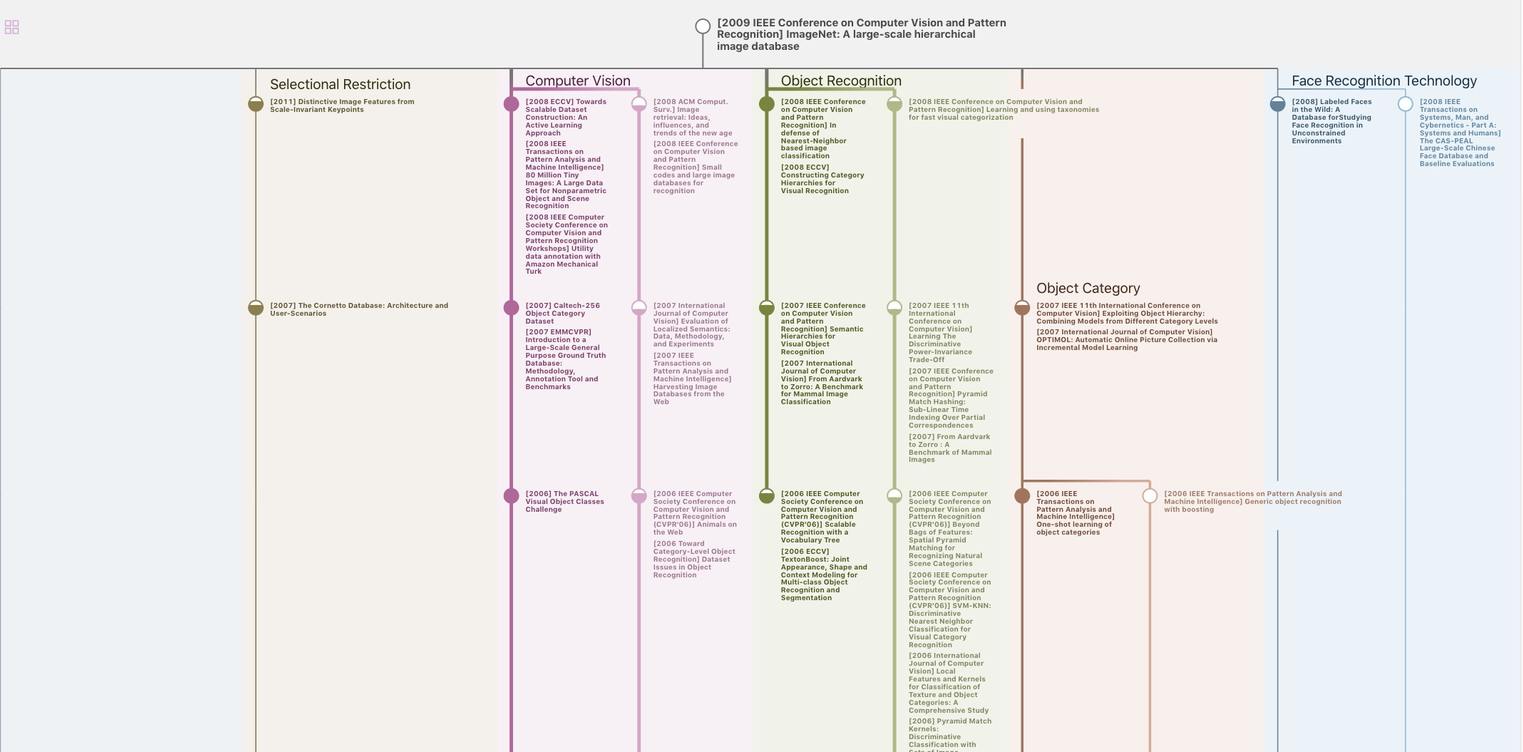
生成溯源树,研究论文发展脉络
Chat Paper
正在生成论文摘要