Radioisotope Identification with Scintillation Detector Based on Artificial Neural Networks Using Simulated Training Data
2020 IEEE Nuclear Science Symposium and Medical Imaging Conference (NSS/MIC)(2020)
摘要
Artificial neural networks (ANN) based on learning the features of the entire measured gamma energy spectrum has been used for radioisotope identification and proved promising especially for gamma-ray spectroscopy with low energy resolution. The implementation of ANN method, however, requires tedious experimental measurement process in generation of training data for various radioisotopes. In this work, we propose an ANN-based radioisotope identification method with simulated training data. Gamma energy spectra of 27 different radioisotopes were generated with Monte Carlo simulation. A detector energy response model was proposed to match the energy spectra generated from simulation and measured from experiment, thus “pseudo” measured energy spectra of various radioisotopes transformed from simulation can be used for ANN training, which eliminates the tedious experimental measurement process for training data generation. To reduce the complexity of the training process, the principal component analysis (PCA) method was used for dimension reduction of the input energy spectra in ANN and the channel number of the energy spectra was reduced from 2000 to 50. The trained ANN was further used to identify experimentally measured gamma energy spectra of various radioisotopes including
60
Co.,
137
CS.,
18
F,
131
I,
226
Ra and
232
Th at 10
3
, 10
4
and 10
5
count levels. In single isotope identification test, with increased count level, higher correct identification rate is achieved and at 10
5
count level, all the isotopes are correctly identified for all the samples. In mixed isotope identification test, at 10
5
count level, all the radioisotope combinations can be identified with a correct identification rate larger than 98%, which demonstrates the feasibility and accuracy of the ANN method. To conclude, the proposed ANN method with simulated training data features good radioisotope identification capability with greatly simplified training data generation process and is feasible for gamma spectroscopy with relatively poor energy resolution.
更多查看译文
关键词
ANN-based radioisotope identification method,27 different radioisotopes,Monte Carlo simulation,detector energy response model,measured energy spectra,ANN training,tedious experimental measurement process,training process,principal component analysis method,input energy spectra,trained ANN,experimentally measured gamma energy spectra,5 count level,single isotope identification test,increased count level,higher correct identification rate,mixed isotope identification test,radioisotope combinations,ANN method,simulated training data features good radioisotope identification capability,greatly simplified training data generation process,gamma spectroscopy,relatively poor energy resolution,scintillation detector,artificial neural networks,entire measured gamma energy spectrum,gamma-ray spectroscopy,low energy resolution
AI 理解论文
溯源树
样例
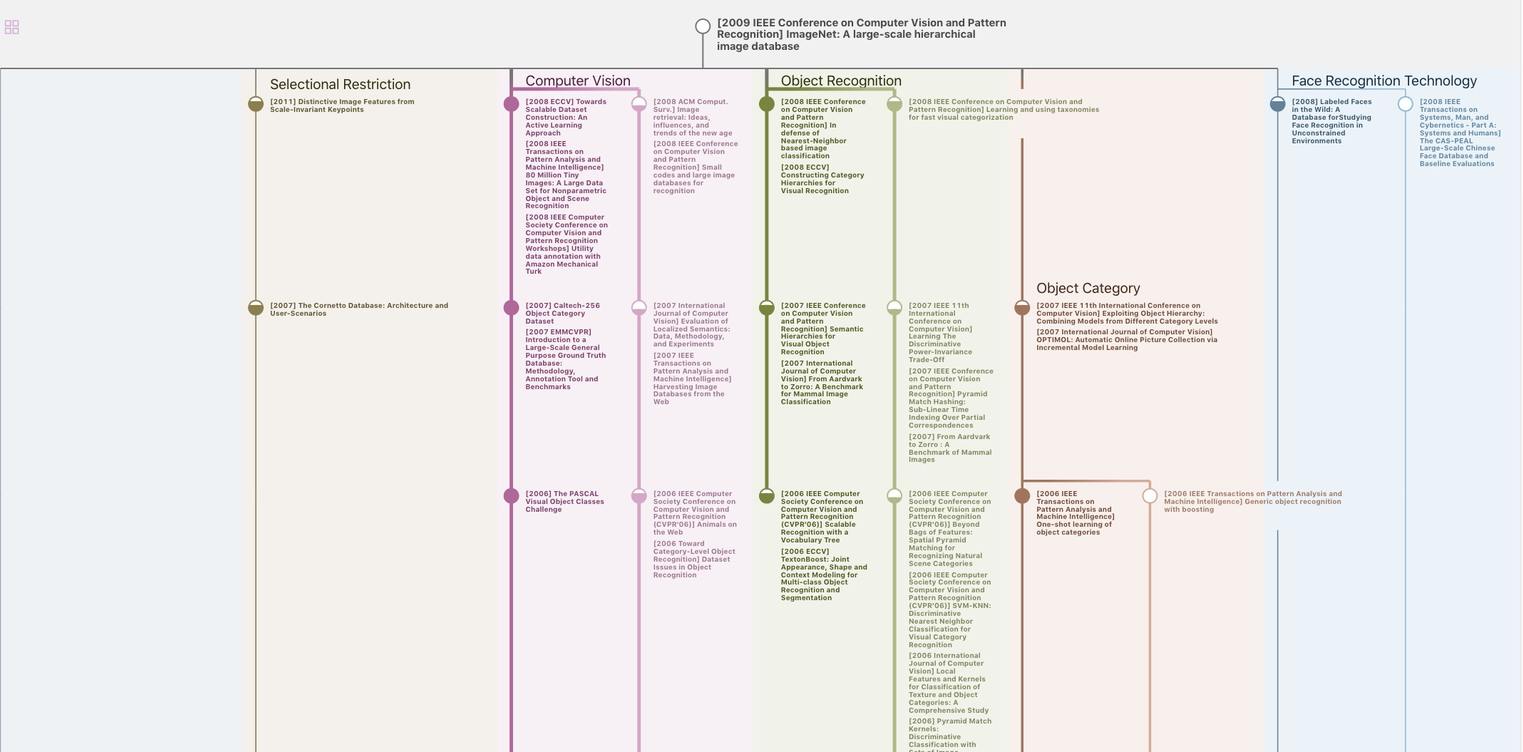
生成溯源树,研究论文发展脉络
Chat Paper
正在生成论文摘要