Abstract 1485: Biomarker development for lung cancer diagnosis using integrative microRNA and gene expression networks
Cancer Research(2014)
Abstract
Proceedings: AACR Annual Meeting 2014; April 5-9, 2014; San Diego, CA
Introduction: We have previously shown that smoking creates a “molecular field of injury” throughout the epithelial cells that line the respiratory tract and that gene-expression alterations in the cytologically-normal mainstem bronchus epithelium can serve as an early detection biomarker for lung cancer. We hypothesize that microRNAs (miRNAs) regulate these airway gene expression changes and that miRNA expression differences in this tissue can be used as biomarkers for lung cancer diagnosis.
Methods: We profiled miRNA expression via small RNA sequencing in bronchial epithelial brushes collected from the mainstem bronchus of 230 subjects undergoing bronchoscopy for suspect lung cancer and gene expression (mRNA) via microarray for 201 matched samples.
Bilal et al. (2013) have shown that incorporating biological knowledge into model building improves prediction. Therefore, we sought to test the hypothesis that including information about the expression levels of the predicted mRNA targets of miRNA may improve miRNA feature selection and aid in interpretation of signatures. We used mirConnX which combines miRNA with mRNA data to create disease-specific, genome-wide regulatory networks.
Results: First, we show that there is a miRNA expression signal for cancer: across many combinations of feature selection methods, predictive models and different parameters within 100 bootstraps to predict cancer phenotype, we find the AUC values obtained are consistently higher compared to the random control procedure where we randomly shuffle the class labels (p < 0.001).
Second, using a training set of 106 samples, we built networks separately for cancer and non-cancer samples, using 10-fold cross validation in order to determine robust cancer and non-cancer specific features. The disease-state specific networks are then aggregated by taking the overlapping features across the ten folds. Next, we select the non-overlapping features (miRNAs and genes) between cancer and non-cancer as those that capture the difference between the two phenotypes. The selected genes are enriched for relevant cancer related pathways, including KEGG pathways in cancer (p = 0.0003), cell cycle (p = 0.008), WNT signaling (p = 0.0001), basal cell carcinoma (p = 0.023), MAPK signaling pathway (p = 0.024), TGF-beta signaling pathway (p = 0.00014), p53 signaling pathway (p = 0.087) etc. Most importantly, the miRNA features from these disease specific networks are found to have higher predictive power (highest AUC 0.72) compared to all miRNA features (highest AUC 0.57), on a second set of 68 samples in cross-validation.
Conclusion: Using novel integrative analysis, we improved miRNA biomarker prediction. This is the first report of cancer-associated miRNA expression differences in cytologically normal bronchial epithelium, for lung cancer diagnosis, and it extends our previous work focused on mRNA biomarkers from this tissue.
Citation Format: Ana Pavel, Joshua Campbell, Gang Liu, Sherry Zhang, Hanqiao Liu, Steven Dubinett, David Elashoff, Kate Porta, Duncan Whitney, Marc Lenburg, Avrum Spira. Biomarker development for lung cancer diagnosis using integrative microRNA and gene expression networks. [abstract]. In: Proceedings of the 105th Annual Meeting of the American Association for Cancer Research; 2014 Apr 5-9; San Diego, CA. Philadelphia (PA): AACR; Cancer Res 2014;74(19 Suppl):Abstract nr 1485. doi:10.1158/1538-7445.AM2014-1485
MoreTranslated text
Key words
biomarker development,lung cancer diagnosis,lung cancer,gene expression
AI Read Science
Must-Reading Tree
Example
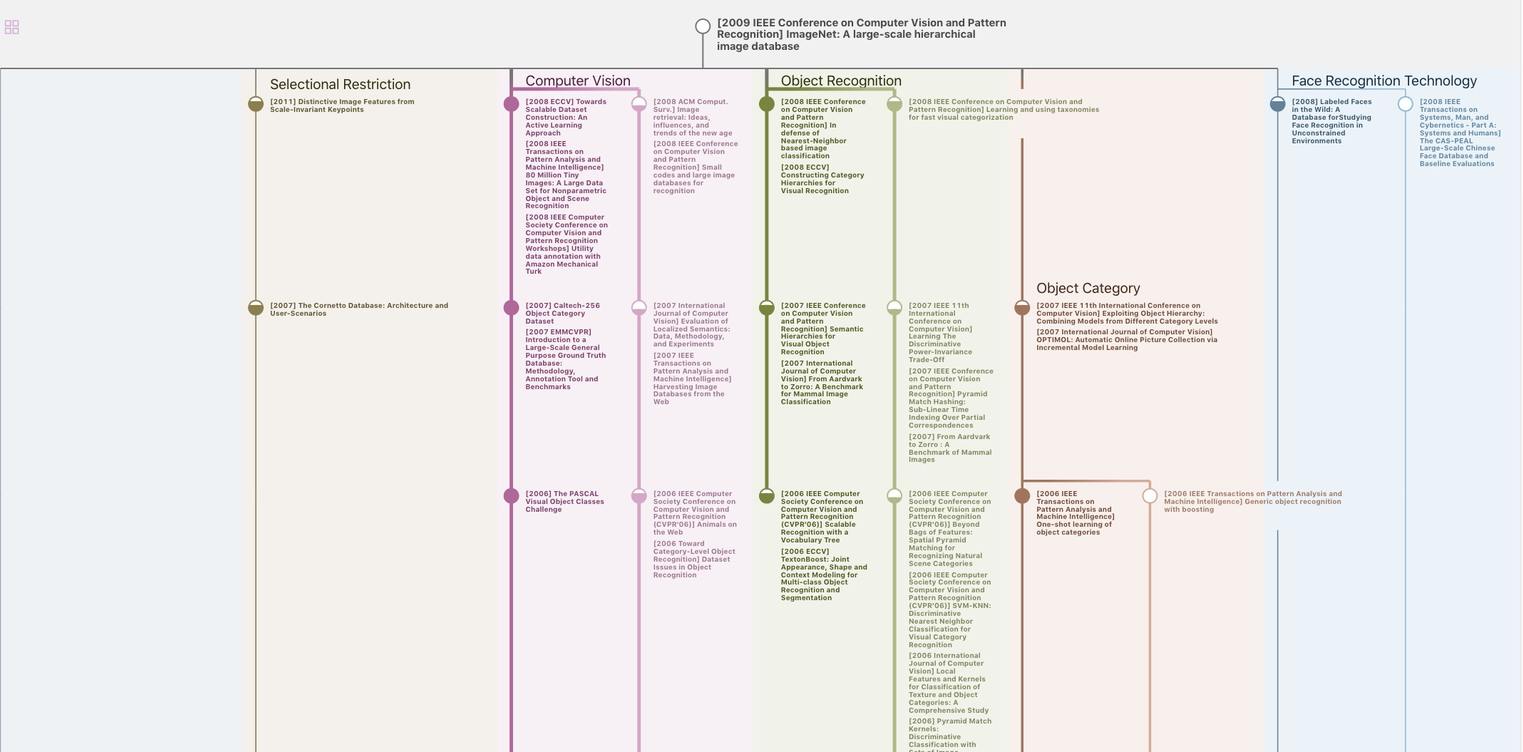
Generate MRT to find the research sequence of this paper
Chat Paper
Summary is being generated by the instructions you defined