Direction-Aware Spatial Context Features for Shadow Detection
computer vision and pattern recognition(2018)
摘要
Shadow detection is a fundamental and challenging task, since it requires an understanding of global image semantics and there are various backgrounds around shadows. This paper presents a novel network for shadow detection by analyzing image context in a direction-aware manner. To achieve this, we first formulate the direction-aware attention mechanism in a spatial recurrent neural network (RNN) by introducing attention weights when aggregating spatial context features in the RNN. By learning these weights through training, we can recover direction-aware spatial context (DSC) for detecting shadows. This design is developed into the DSC module and embedded in a CNN to learn DSC features at different levels. Moreover, a weighted cross entropy loss is designed to make the training more effective. We employ two common shadow detection benchmark datasets and perform various experiments to evaluate our network. Experimental results show that our network outperforms state-of-the-art methods and achieves 97% accuracy and 38% reduction on balance error rate.
更多查看译文
关键词
attention weights,aggregating spatial context features,detecting shadows,spatial recurrent neural network,direction-aware attention mechanism,direction-aware manner,image context,global image semantics,direction-aware spatial context features,weighted cross entropy loss
AI 理解论文
溯源树
样例
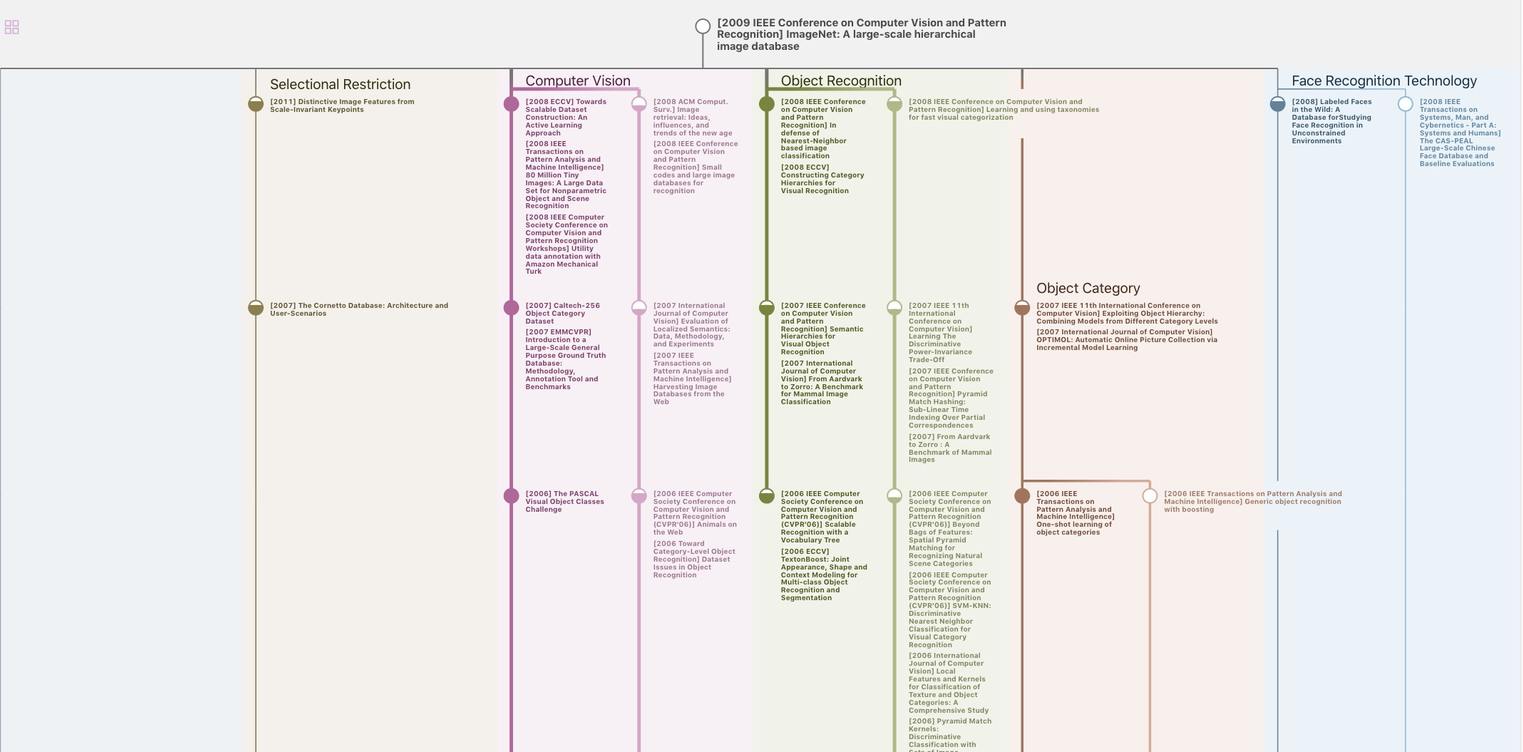
生成溯源树,研究论文发展脉络
Chat Paper
正在生成论文摘要