Optimal state selection and tuning parameters for a degradation model in bearings using Mel-Frequency Cepstral Coefficients and Hidden Markov Chains
Ingeniare. Revista chilena de ingeniería(2016)
摘要
espanolEl mantenimiento preventivo es una filosofia para la administracion de activos que persigue el fin de maximizar la operacion mediante rutinas de inspeccion, las cuales aumentan su frecuencia a medida que no se presenta un estado anormal, conllevando a que se incremente la probabilidad de falla debido a la mayor cantidad de intervenciones y por el error humano inherente. Recientemente la investigacion de pronostico ha sido utilizada con el objetivo de alcanzar estrategias efectivas de mantenimiento y con el fin de evaluar y administrar el riesgo residual en equipos que sufren degradacion. El pronostico se relaciona con la estimacion de vida util restante (RUL) de un activo al predecir su estado de salud durante la progresion de la degradacion. Este articulo presenta el desarrollo de un sistema automatico para identificar tipos de fallos en rodamientos, empleando Coeficientes Cepstrales en la escala de Mel (MFCC) como conjunto de caracteristicas y Cadenas Ocultas de Markov (HMC) con observaciones discretas como clasificador. Se hace hincapie en la seleccion optima de estados del clasificador HMM mediante el criterio de curvas ROC (Receiver Operating Characteristic). Las caracteristicas son discretizadas empleando agrupamiento por k-medias. Las senales de estudio son datos de vibracion provenientes de rodamientos. Se emplean dos bases de datos que presentan cuatro escenarios diferentes de operacion, a saber: normal, falla en pista interna, falla en pista externa y falla en bola. Una de las bases permite diferenciar niveles de severidad para cada escenario de operacion. EnglishPreventive maintenance is a philosophy for assets management that aims to maximize operation through routine inspections with increasing frequency when no abnormalities are exhibit. This leads to an increase in the probability of failure due to the repetitive intervention and the inherent human error. Recently, forecasting research, or predictive research, have been addressed in order to obtain effective maintenance strategies and evaluate and manage the residual risk in equipment susceptible to degradation. Predictive research is related to the estimation of an active's Remaining Useful Life (RUL) by predicting its health state through the progression of its degradation. This article presents the development of an automated system that identifies types of faults in bearings, using Cepstral Coefficients on the Mel scale (MFCC) as the features set for description, and Hidden Markov Chains (HMC) with discrete observations as the classification method. Here we emphasizes on the optimal selection of the states in the HMM classifier using the ROC (Receiver Operating Characteristic) curves criteria. Features are discretized using clustering by k-means. Signals in this study are vibrations signals from the bearings in electrical machinery. The two databases used here are labeled with four different operation scenarios: normal, inner ring fault, outer ring fault, and rolling element fault. One of the databases allows for differentiation in severity levels for each scenario.
更多查看译文
AI 理解论文
溯源树
样例
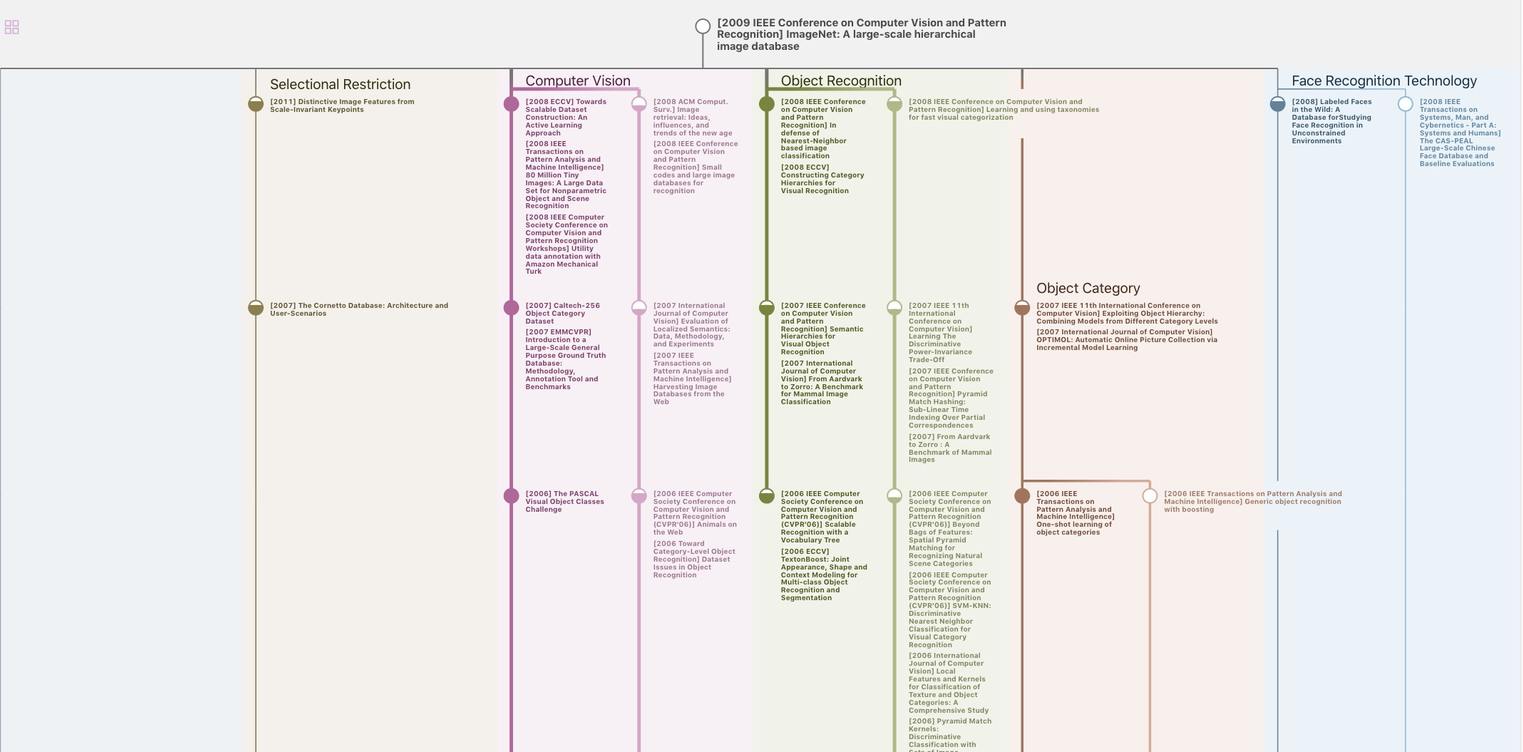
生成溯源树,研究论文发展脉络
Chat Paper
正在生成论文摘要