Convolutional Neural Network-Based Automatic Classification for Incomplete Antibody Reaction Intensity in Solid Phase Anti-Human Globulin Test Image.
Medical & biological engineering & computing(2022)
Abstract
The precise classification of incomplete antibody reaction intensity (IARI) in hydrogel chromatography medium high density medium solid-phase Coombs test is essential for haemolytic disease screening. However, an automatic and contactless method is required for accurate classification of IARI. Here, we present a deep ensemble learning model that integrates five different convolutional neural networks into a single model for IARI classification. A dataset, including 1628 IARI images and corresponding labels of IARI categories ((-), (1 +), (2 +), (3 +), and (4 +)), was used. We trained our model using 1302 IARIs and validated its performance using 326 IARIs. The proposed model achieved 100%, 99.4%, 99.4%, 100%, and 100% accuracies in the ( −), (1 +), (2 +), (3 +), and (4 +) categories, respectively. The results were compared with those of manual classification by immunologists (average accuracy: 99.8% vs. 88.3%, p < 0.01). Following model assistance, all three immunologists achieved increased accuracy (average accuracy: + 6.1%), with the average accuracy of junior immunologists maximum increasing by 11.3%. The time required for model classification was 0.094 s·image–1, whereas that required manually was 5.528 s·image–1. The proposed model can thus substantially improve the accuracy and efficiency of IARI classification and facilitate the automation of haemolytic disease screening equipment.
MoreTranslated text
Key words
Antibody,Coombs test,Neural network,Blood transfusion
AI Read Science
Must-Reading Tree
Example
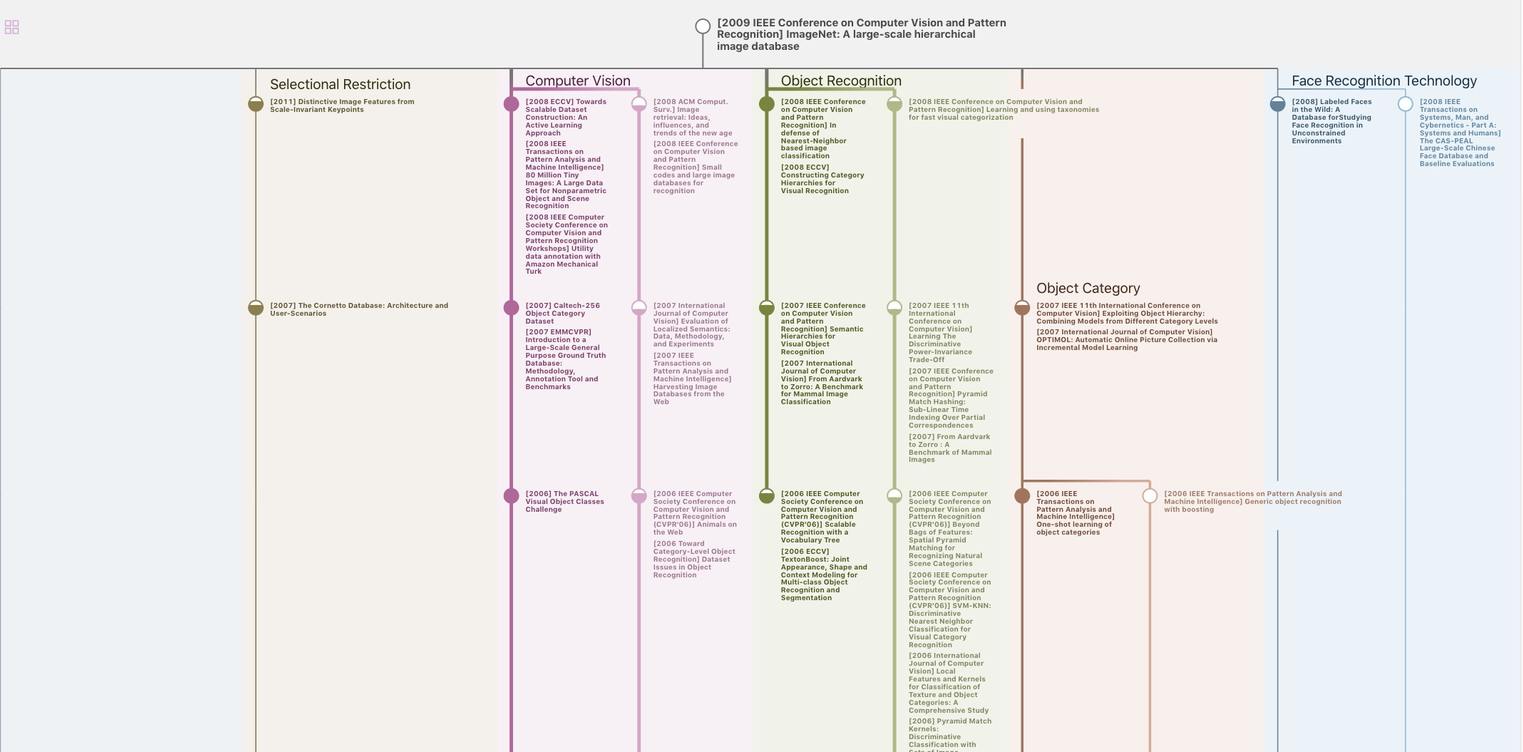
Generate MRT to find the research sequence of this paper
Chat Paper
Summary is being generated by the instructions you defined