Airborne DLSLA 3-D SAR Image Reconstruction by Combination of Polar Formatting and $L_1$ Regularization
IEEE Transactions on Geoscience and Remote Sensing(2016)
Abstract
Airborne downward-looking sparse linear array 3-D synthetic aperture radar (DLSLA 3-D SAR) operates downward-looking observation and obtains the 3-D microwave scattering information of the observed scene. The cross-track physical sparse linear array is often configured to obtain uniform virtual phase centers in order to adopt the frequency-domain algorithm. However, the virtual phase centers usually have to be nonuniformly and sparsely distributed due to the array elements' installation locations restricted by the airborne platform and the airborne wing tremor effect. In this state, the frequency-domain algorithm cannot be directly used. In this paper, a DLSLA 3-D SAR image reconstruction algorithm that combines polar formatting and $L_1$ regularization is presented. Wave propagation and along-track dimensional imaging are first finished after polar formatting and wavefront curvature phase error compensation; then, cross-track dimensional imaging is completed with the $L_1$ regularization technique. The proposed algorithm is applicable to airborne DLSLA 3-D SAR imaging under nonuniformly and sparsely distributed virtual phase centers condition. The proposed algorithm was verified by 3-D distributed scene simulation experiment (P-band circular SAR image was selected as radar cross-section input, and X-band digital elevation model of the same area was selected as the coordinate positions of the scene) and the field experiment. Image reconstruction results and image reconstruction performances, such as normalized radar cross section, height errors, and orthographic projection image grayscale distribution, are demonstrated and analyzed with different signal-to-noise ratios, different array sparsity, and the incomplete compensated residual oscillation error 3-D distributed scene simulation experiments. Simulation and field experimental results show the good performance in focusing and the robustness of the proposed algorithm.
MoreTranslated text
Key words
polar formatting,image reconstruction,regularization,inline-formula,tex-math,tex-math,inline-formula
AI Read Science
Must-Reading Tree
Example
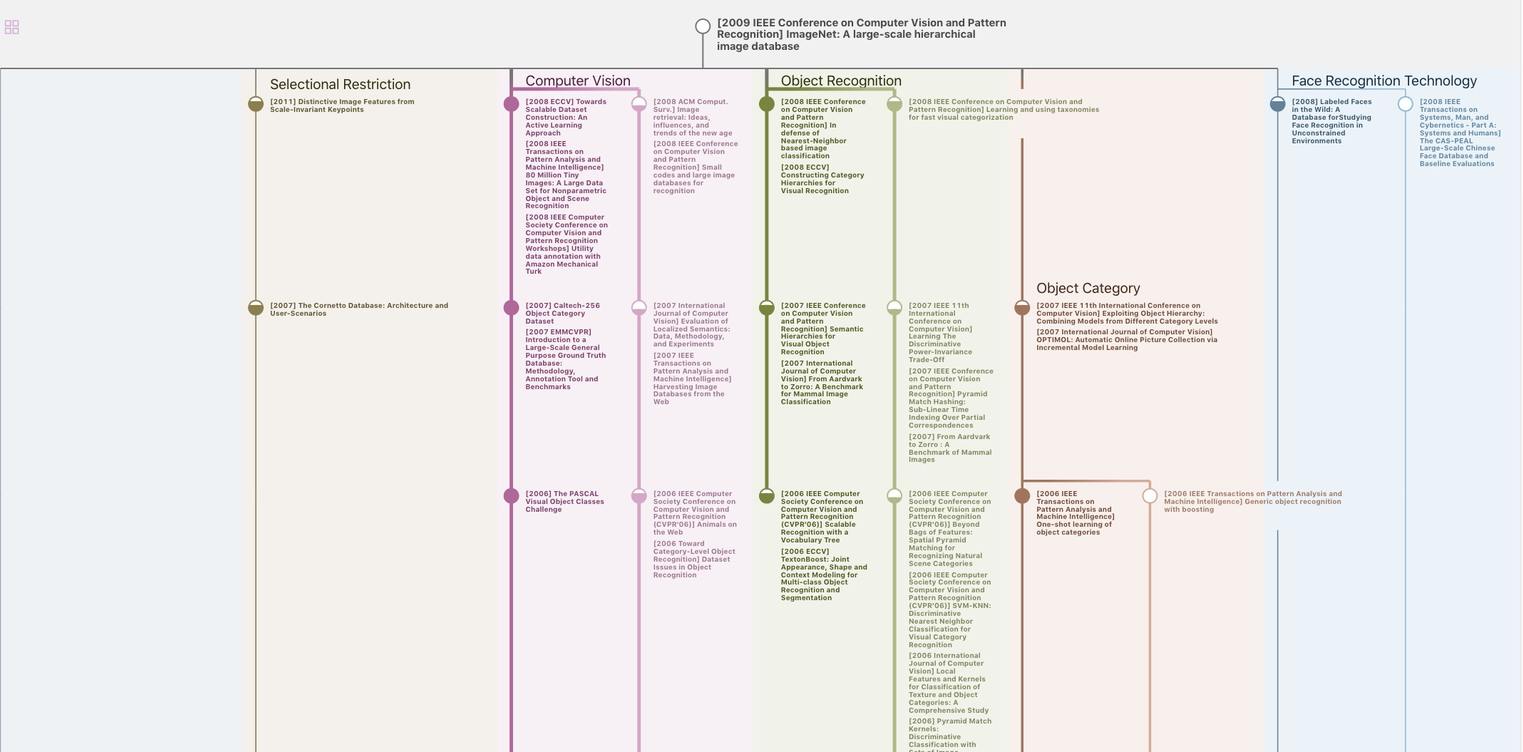
Generate MRT to find the research sequence of this paper
Chat Paper
Summary is being generated by the instructions you defined