Abstract IA05: New methods and better theory for pre-clinical cancer pharmacogenomics
Cancer Research(2017)
Abstract
Drug dose-response measurements are the cornerstone of pre-clinical assessments of new and existing therapeutics. As a means to identify biomarkers of drug response, high-throughput studies have attempted to relate drug sensitivity across hundreds of cell lines and hundreds of drugs to inhibition of mitogensis and induction of apoptosis. And, by analogy with antibiotic susceptibility testing, it is increasingly possible to screen primary human tumor cells as a means to personalize therapy for individual patients. I will describe new ways of performing such studies that promise to avoid common confounders. The metrics usually used to parameterize drug response (IC50, Emax, or AUC) are based on assessing the cell count of a treated condition relative to an untreated control. All of these metrics suffer from a fundamental flaw: cell lines divide at very different rates and those that undergo more divisions over the course of an assay are scored as more sensitive than cell lines with fewer divisions, even if their inherent drug sensitivities are identical. We developed a new method to parameterize drug response, the growth rate inhibition (GR) metrics, which is based on the ratio of growth rates under treatment conditions in relation to an untreated control. GR metrics are independent of cell growth over the course of the experiment and thus enable us to accurately compare cell lines with varying growth rates or experimental conditions that can alter growth rates. I will describe the use of GR metrics to analyze six breast cancer cell lines treated with ~100 small molecule inhibitors at six doses. We focused on inhibitors targeting key signaling nodes such as PI3K, AKT, or MAPK, as well as receptor tyrosine kinases (RTKs) and cyclin-dependent kinases (CDKs), and many of the drugs studied are currently in clinical trials. We measured the cell9s transcriptional response at 3 hours and 24 hours using the L1000 transcriptional profiling assay and scored phenotypes by imaging at 3 days. We find that some drugs, particularly those targeting cell cycle kinases and chaperones, elicit near identical responses in all cell lines both transcriptionally and phenotypically. Kinase inhibitors, on the other hand, predominantly elicit cell type-specific responses at the molecular and phenotypic levels. Interestingly, a subset of signaling kinase inhibitors induces significant change at the transcriptional level without affecting cell growth. Analysis of the transcriptional profiles under these conditions allows us to identify synergistic drug combinations, which act by blocking adaptive drug resistance. Thus, inexpensive, high-throughput transcript profiling can uncover subtle mechanisms of drug sensitivity and resistance and guide the design of effective drug combinations. Our work shows the value of using drug response metrics that are independent of growth rate. This applies to high-throughput profiling of cell lines, but also to patient-derived tumor and normal cells. GR metrics are also useful whenever cells are manipulated in ways that can affect division times (e.g. by varying the microenvironment or introducing genetic changes). We expect GR metrics to improve pre-clinical pharmacology and precision therapeutic assays employing patient-derived tumor cells. Citation Format: Mario Niepel, Marc Hafner, Qiaonan Duan, Aravind Subramanian, Avi Ma9ayan, Peter K. Sorger. New methods and better theory for pre-clinical cancer pharmacogenomics. [abstract]. In: Proceedings of the AACR Special Conference on Engineering and Physical Sciences in Oncology; 2016 Jun 25-28; Boston, MA. Philadelphia (PA): AACR; Cancer Res 2017;77(2 Suppl):Abstract nr IA05.
MoreTranslated text
Key words
abstract ia05,cancer,pre-clinical
AI Read Science
Must-Reading Tree
Example
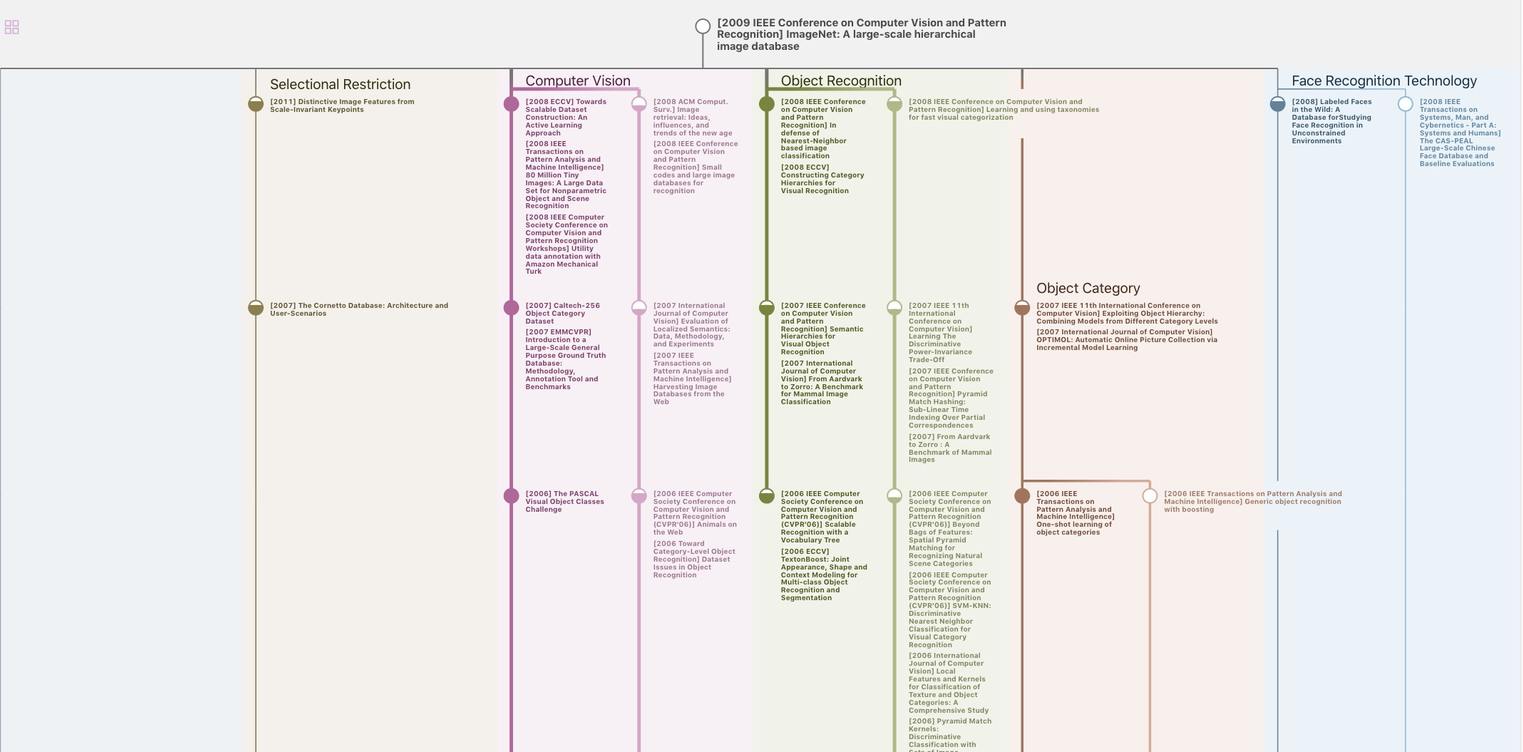
Generate MRT to find the research sequence of this paper
Chat Paper
Summary is being generated by the instructions you defined