External Sparsity Combined with Internal Low-Rankness for Image Denoising
2021 7th International Conference on Computer and Communications (ICCC)(2021)
摘要
Nonlocal self-similarity (NSS) as a remarkable prior has been successfully applied to image processing including image denoising. For each local patch in an image, we can search for many nonlocally similar patches under NSS prior and stack them into a group, and then process the group instead of a patch to better capture the nonlocal structure. Most existing methods consider the NSS either of the internal degraded image or from an external clean corpus, which may limit the denoising performance. In this paper, we propose a hybrid model to catch both the external and internal NSS. Specifically, we develop a model with two regularizers, the internal NSS is exploited by a low-rankness regularizer, and the external NSS is exploited by a sparse regularizer. An alternating minimization method is developed to solve our model. Experimental results demonstrate that our algorithm achieves better results compared with several state-of-the-art denoising methods.
更多查看译文
关键词
nonlocal self-similarity,low-rankness,sparse regularization,image denoising
AI 理解论文
溯源树
样例
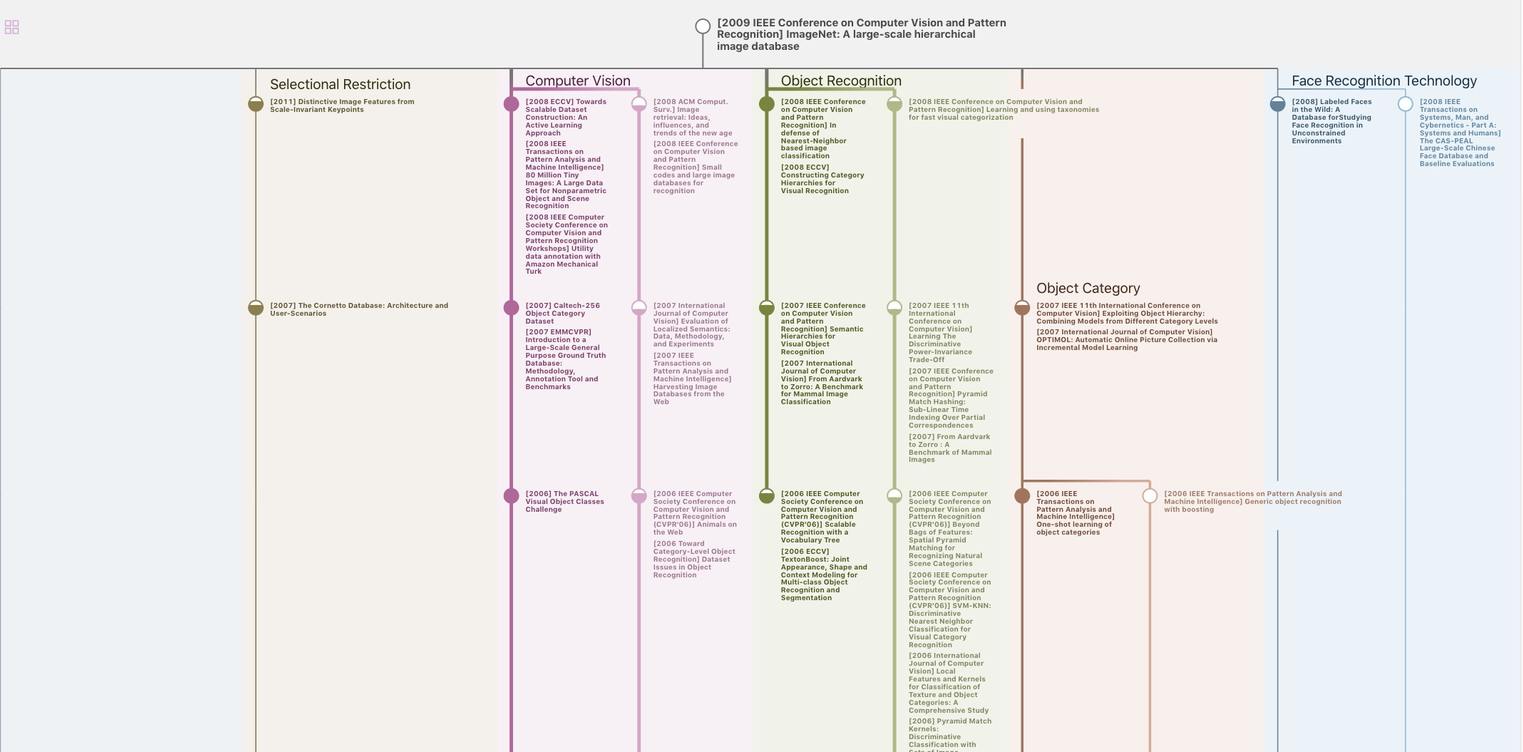
生成溯源树,研究论文发展脉络
Chat Paper
正在生成论文摘要