One Versus All for Deep Neural Network for Uncertainty (OVNNI) Quantification
IEEE ACCESS(2022)
Abstract
Deep neural networks (DNNs) are powerful learning models, yet their results are not always reliable. This drawback results from the fact that modern DNNs are usually overconfident, and consequently their epistemic uncertainty cannot be straightforwardly characterized. In this work, we propose a new technique to quantify easily the epistemic uncertainty of data. This method consists in mixing the predictions of an ensemble of DNNs trained to classify One class versus All the other classes (OVA) with predictions from a standard DNN trained to perform All versus All (AVA) classification. First of all, the adjustment provided by the AVA DNN to the score of the base classifiers allows for a more fine-grained inter-class separation. Moreover, the two types of classifiers enforce mutually their detection of out-of-distribution (OOD) samples, circumventing entirely the requirement of using such samples during training. The additional cost involved by the construction of the ensemble is offset by the ease of use of our proposed strategy and by its enhanced generalization potential, as it does not bind its performance in a given context to specific OOD datasets. The extensive experiments confirm the wide applicability of our approach, and our method achieves state of the art performance in quantifying OOD data across multiple datasets and architectures while requiring little hyper-parameter tuning.
MoreTranslated text
Key words
Uncertainty,Training,Task analysis,Anomaly detection,Training data,Semantics,Deep learning,Uncertainty estimation,DNN ensembles,one vs all classification,all vs all classification
AI Read Science
Must-Reading Tree
Example
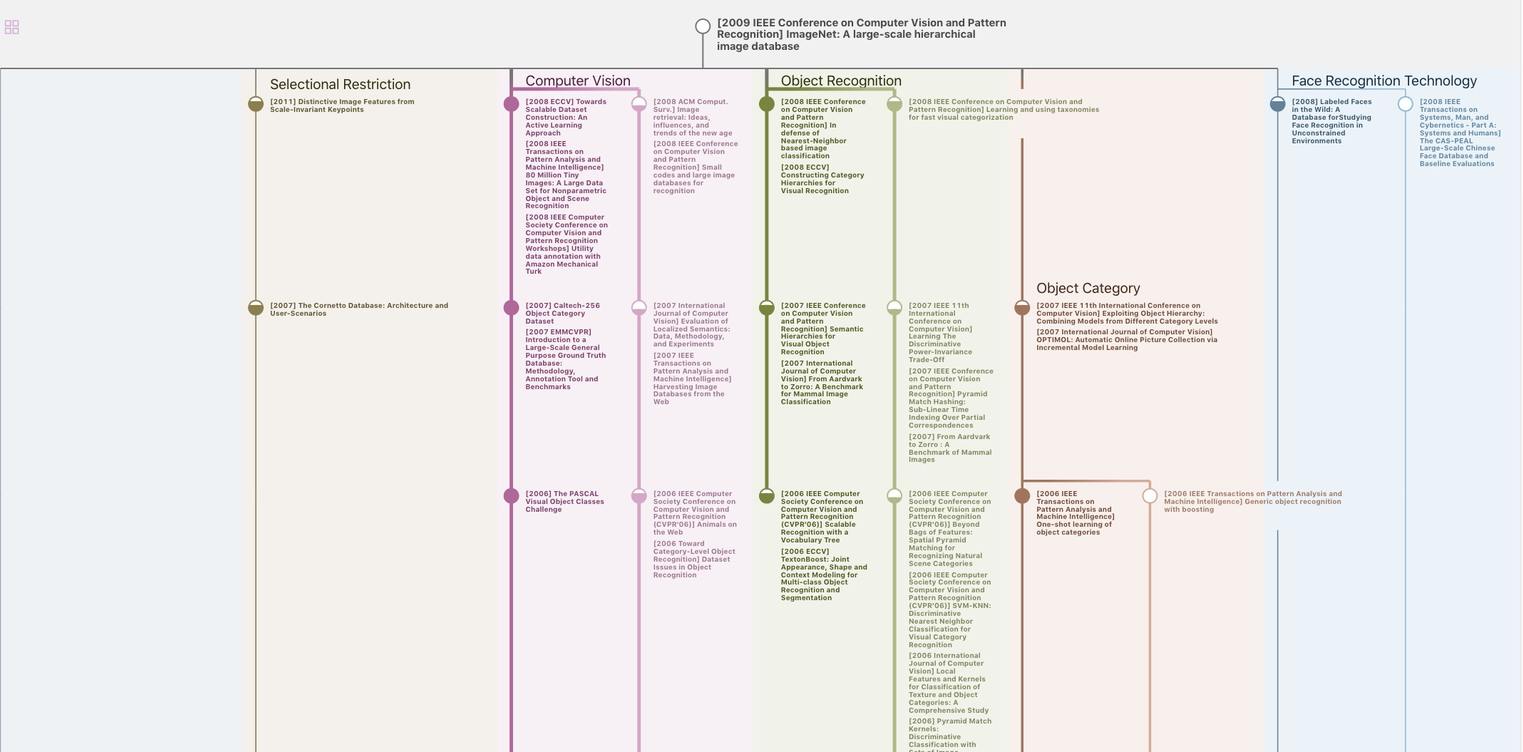
Generate MRT to find the research sequence of this paper
Chat Paper
Summary is being generated by the instructions you defined