Tailoring electric field signals of nonequilibrium discharges by the deep learning method and physical corrections
PLASMA PROCESSES AND POLYMERS(2022)
Abstract
Smart modulation of discharges is necessary to generate specific reactive species in an energy-efficient way. A physics corrected plasma + deep learning framework, the DeePlaskin, is proposed. This framework can be used for the nonequilibrium plasma systems that can be described by a global chemistry model (assuming global uniformity, e.g., in spark channels or the early afterglow of the fast ionization wave discharges). Knowing the kinetics scheme and the predefined temporal evolution of target species, we will be able to reconstruct the temporal profile of the reduced electric field E / N and all the other species. To generate the same concentration of O atom at the end of the discharge, the electric field profiles customized by the DeePlaskin differ significantly depending on the predefined evolution, resulting in different energy consumption. The combination of the deep learning method and plasma physics/chemistry model shows great potential in optimizing the design of plasma sources in practical applications.
MoreTranslated text
Key words
deep learning,global model,inverse model,plasma chemistry,plasma sources
AI Read Science
Must-Reading Tree
Example
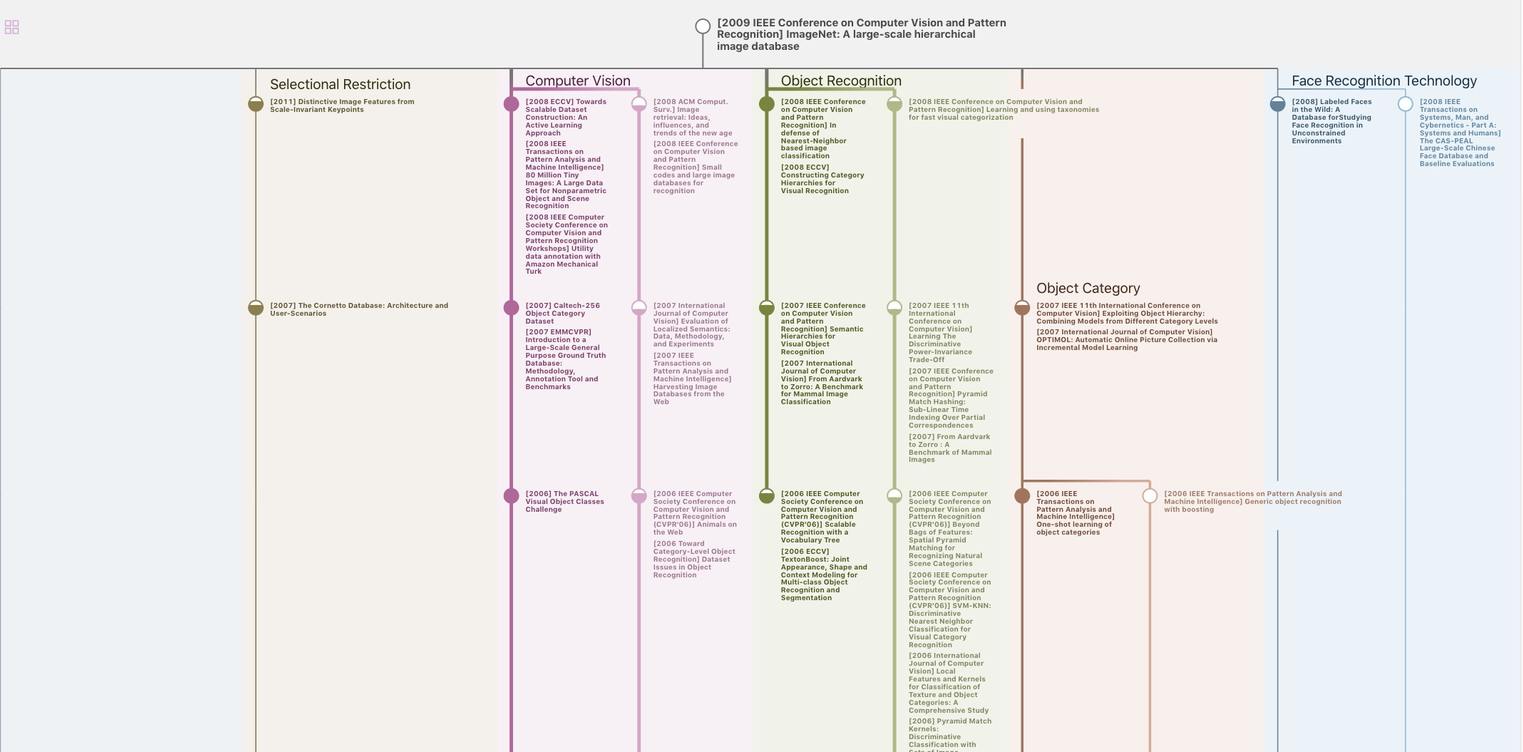
Generate MRT to find the research sequence of this paper
Chat Paper
Summary is being generated by the instructions you defined