Efficient Task Offloading Using Reinforcement Learning in Mobile Edge Computing for Network RTK
2021 7th International Conference on Computer and Communications (ICCC)(2021)
摘要
Mobile Edge Computing (MEC) is a promising approach to satisfy the growing demand for computationally intensive computing tasks in network Real-Time Kinematic (RTK). The device can offload the computing task to a close edge server, which is conducive to the energy saving of the device but results in the increase of the transmission latency and the network load. In this paper, a mobile edge computing model is built based on networks RTK (RTK-MEC). For the purpose of balancing the relationship between energy consumption and time delay in this model, an efficient strategy of channel allocation and task offloading is proposed to obtain the minimum cost of the entire system. Considering the mixed-integer nonlinear problem (MINLP) in computation offloading, we adopt the Q-learning method of Reinforcement Learning (RL) to address this problem. Numerical results demonstrate that the proposed strategy can significantly reduce the total system cost by 15.5% compared to the baseline algorithm.
更多查看译文
关键词
RTK,MEC,Offloading,Q-learning
AI 理解论文
溯源树
样例
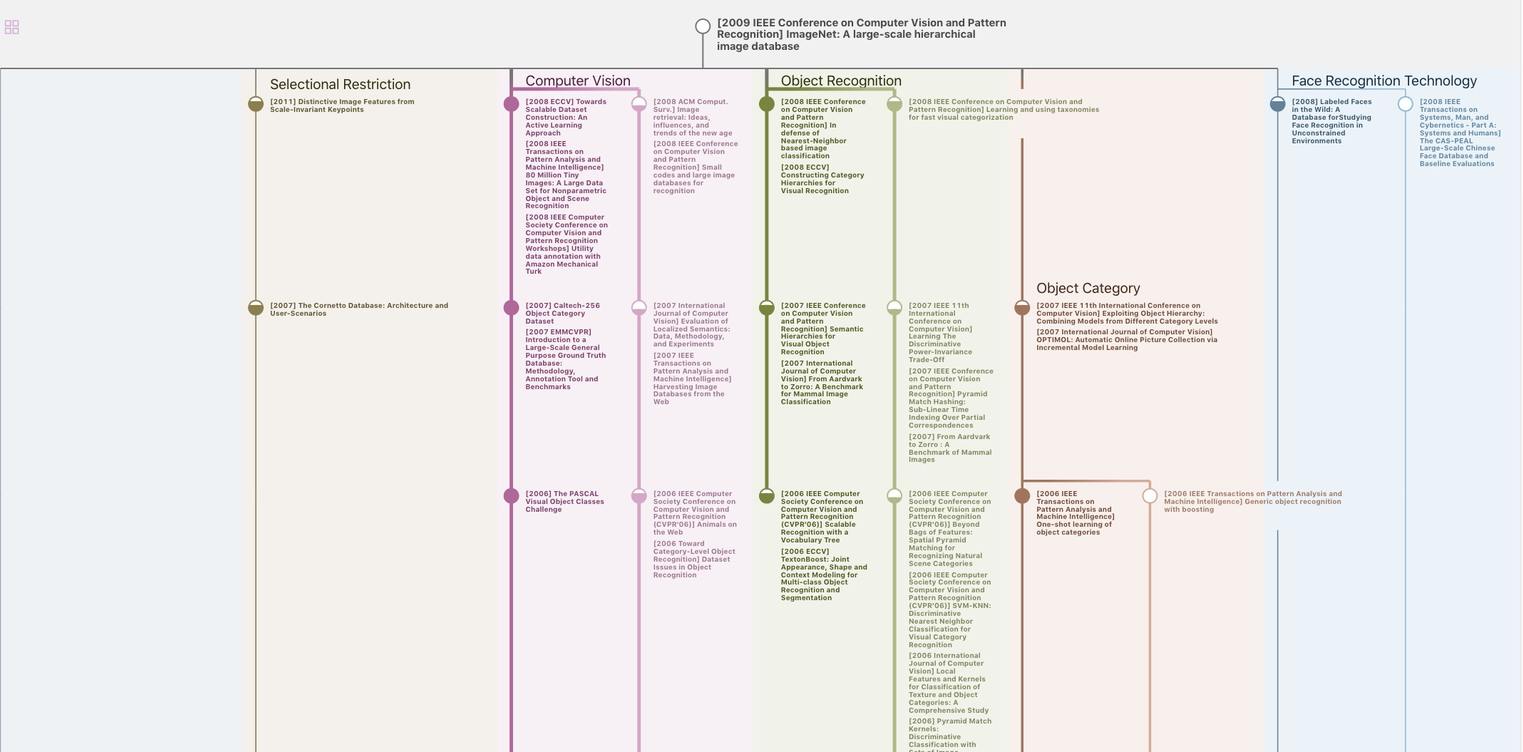
生成溯源树,研究论文发展脉络
Chat Paper
正在生成论文摘要