Unsupervised Learning for Human Mobility Behaviors
INFORMS JOURNAL ON COMPUTING(2022)
摘要
Learning human mobility behaviors from location-sensing data are crucial to mobility data mining because of its potential to address a range of analytical purposes in mobile context reasoning, including exploration, inference, and prediction. However, existing approaches suffer from two practical problems: temporal and spatial sparsity. To address these shortcomings, we present two unsupervised learning methods to model the mobility behaviors of multiple users (i.e., a population), considering efficiency and accuracy. These methods intelligently overcome the sparsity in individual data by seeking temporal commonality among users' heterogeneous location behaviors. The advantages of our models are highlighted through experiments on several real-world mobility data sets, which also show how our methods can realize the three analytical purposes in a unified manner.
更多查看译文
关键词
human mobility behaviour,unsupervised learning,mobile sensing,sparslty
AI 理解论文
溯源树
样例
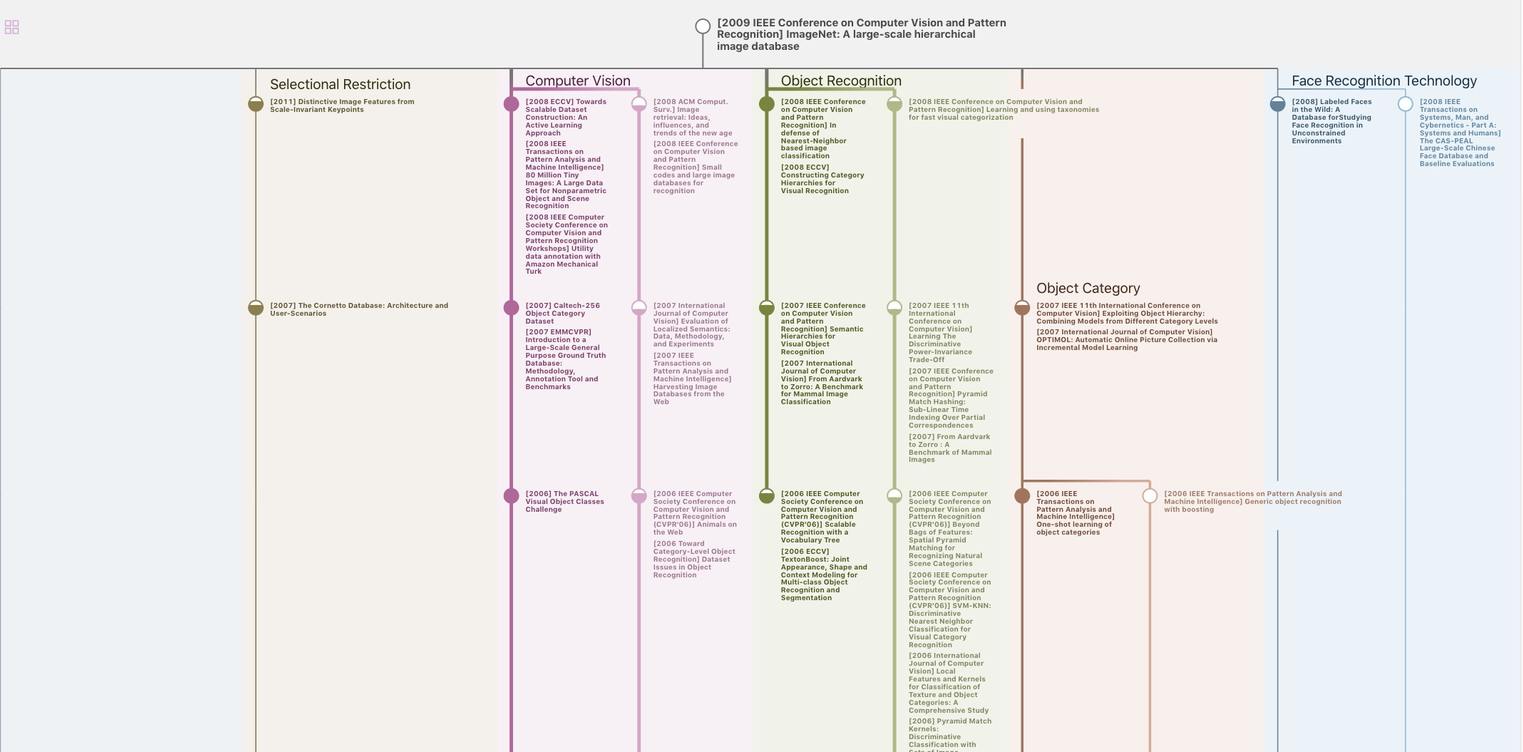
生成溯源树,研究论文发展脉络
Chat Paper
正在生成论文摘要