A Data Enhancement Approach to Improve Machine Learning Performance for Predicting Health Status Using Remote Healthcare Data
2020 2nd International Conference on Advanced Information and Communication Technology (ICAICT)(2020)
摘要
Machine Learning (ML) is becoming tremendously important to improve the performance of remote healthcare systems. Portable health clinic (PHC), a remote healthcare system contains a triage function that classifies the patients in two major groups - (a)healthy and (b)unhealthy. Unhealthy patients require regular health checkups. This paper aims to predict the status of the registered patients to decide the follow-up date and frequency. Health management cost can be reduced by decreasing the number of follow-up frequency. We carried out an experiment on 271 corporate members and monitored their health status in every three months and collected four phases of data. The data records contain clinical data, socio-demographical data, dietary behavior data. However, most of the machine learning algorithms can not directly work with categorical data. Several encoding techniques are available which can also enhance the prediction performance. In this paper, We applied three encoding techniques and proposed a new encoding approach to handle categorical variables. The result shows that Random Forest Classifier performs the best with 95.33% accuracy. A comparison chart displaying the performance of eight different supervised learning algorithms in terms of three existing encoding mechanisms is reported.
更多查看译文
关键词
Machine Learning,Supervised Learning Algorithm,Remote Healthcare,Portable Health Clinic (PHC),Data Preprocessing,Categorical Variables,Encoding
AI 理解论文
溯源树
样例
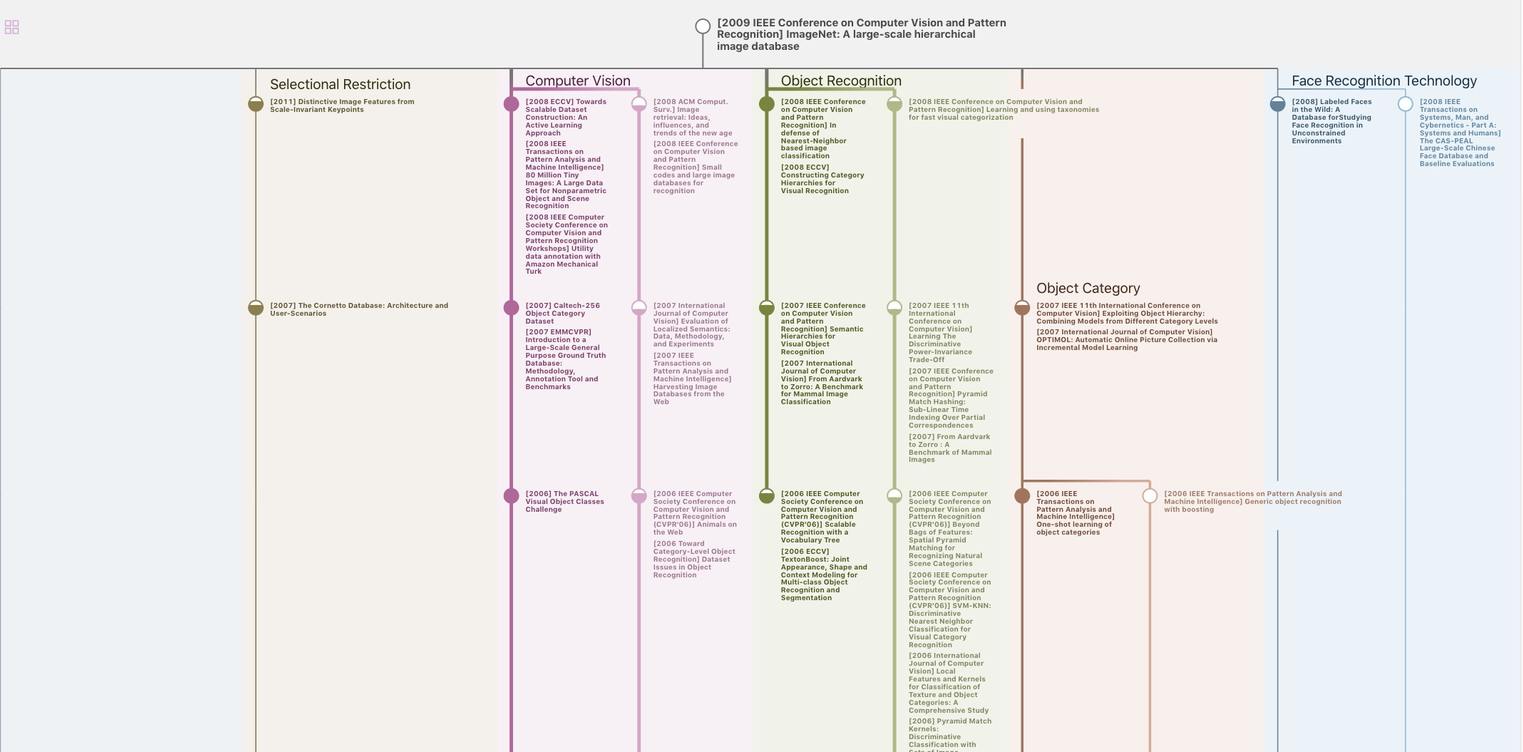
生成溯源树,研究论文发展脉络
Chat Paper
正在生成论文摘要