A Progressive Multi-View Learning Approach for Multi-Loss Optimization in 3D Object Recognition
IEEE SIGNAL PROCESSING LETTERS(2022)
摘要
3D object recognition is a well studied 2D multi-view object classification task that achieves high accuracy if the object textures are distinctive. However, if objects are texture-less and are only differentiable by their shapes but at certain viewpoints. Thus, the problem is still very challenging. Furthermore, the existing methods are mostly based on supervised learning with lots of images per object which are difficult to collect and label them for training. In this letter, we introduced a multi-loss view invariant stochastic prototype embedding to minimize and improve the recognition accuracy of novel objects at different viewpoints by using a progressive multi-view learning approach. An extensive experimental results show that the proposed method outperforms the state-of-the-art methods on different types datasets and also on different backbones.
更多查看译文
关键词
Object recognition,Training,Three-dimensional displays,Task analysis,Visualization,Prototypes,Solid modeling,3D unseen learning,DCNN,progressive multi-view learning,object detection,self-supervised learning
AI 理解论文
溯源树
样例
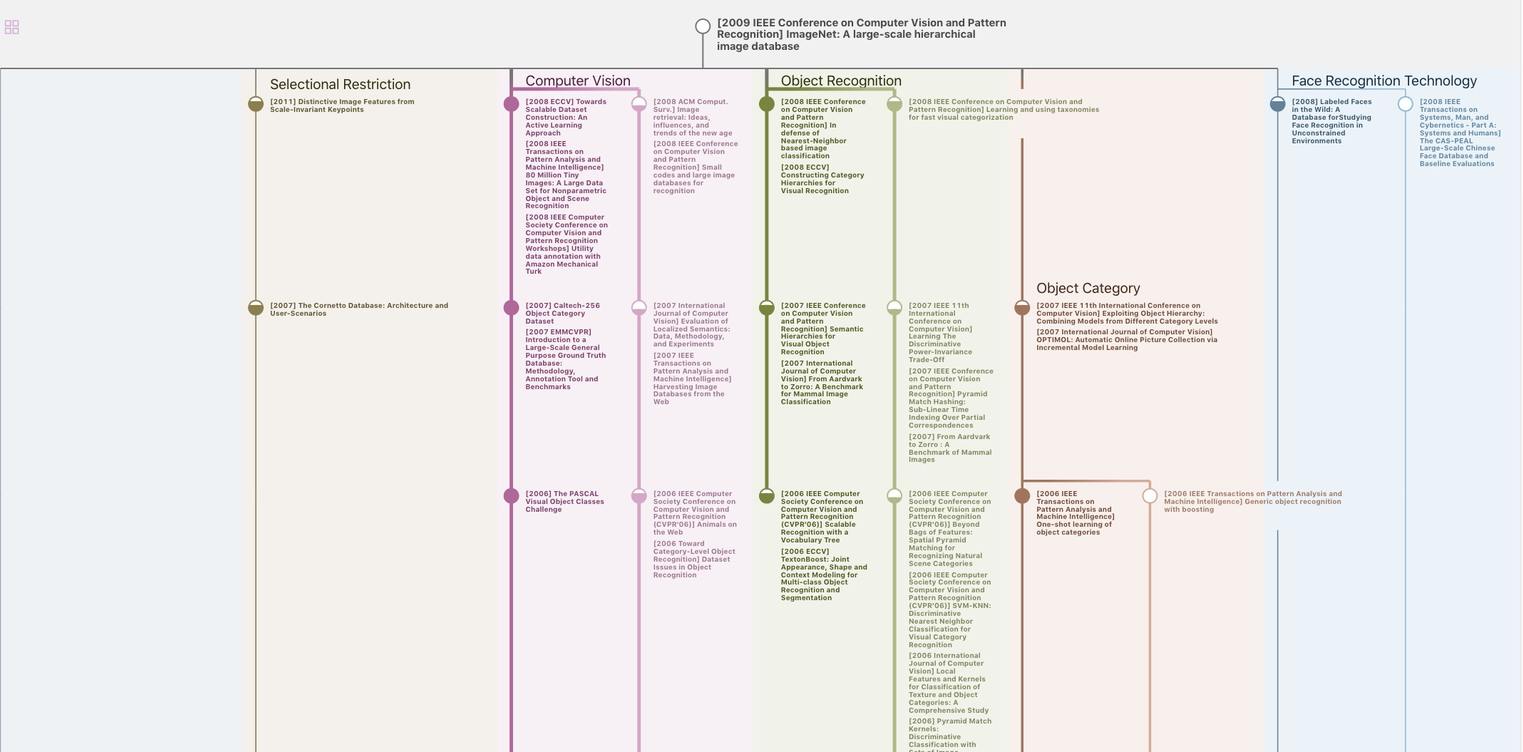
生成溯源树,研究论文发展脉络
Chat Paper
正在生成论文摘要