Facilitating Energy-Efficient Operation of Smart Building using Data-driven Approaches
2021 North American Power Symposium (NAPS)(2021)
Abstract
The building operations and control have become automated with the help of information and communication technologies (ICT) leading to a new paradigm shift i.e. Smart Buildings, which can improve the comfort and efficiency of the user while consuming less energy than a traditional building. Smart buildings may also interact with the power grid, which is becoming increasingly crucial for utility demand response programs, which necessitates precise prediction of the smart buildings electricity usage. Hence, the paper focuses on the data-driven approaches for predicting electricity usage in a smart building in absence of the system model. The technique such as dynamic mode decomposition (DMD) and deep learning models such as recurrent neural network (RNN), long short term memory (LSTM), and gated recurrent unit (GRU) are considered in this paper. The paper proposes the development of a hybrid model which is a blend of the best features of RNN and GRU for predicting electricity consumption. Another highlight of the paper is the proposition of hyperparameters which ensures to improve the prediction accuracy of the deep learning methods. For testing the effectiveness of all the methods in predicting electricity usage, a comparative study is carried out on two different types of smart buildings. Finally, based on the result it can be claimed that the proposed hybrid model along with the introduction of hyperparameter outperforms other methods of deep learning and DMD as validated by error metrics.
MoreTranslated text
Key words
Deep Learning model,Dynamic Mode Decomposition,Energy Management,Smart Building
AI Read Science
Must-Reading Tree
Example
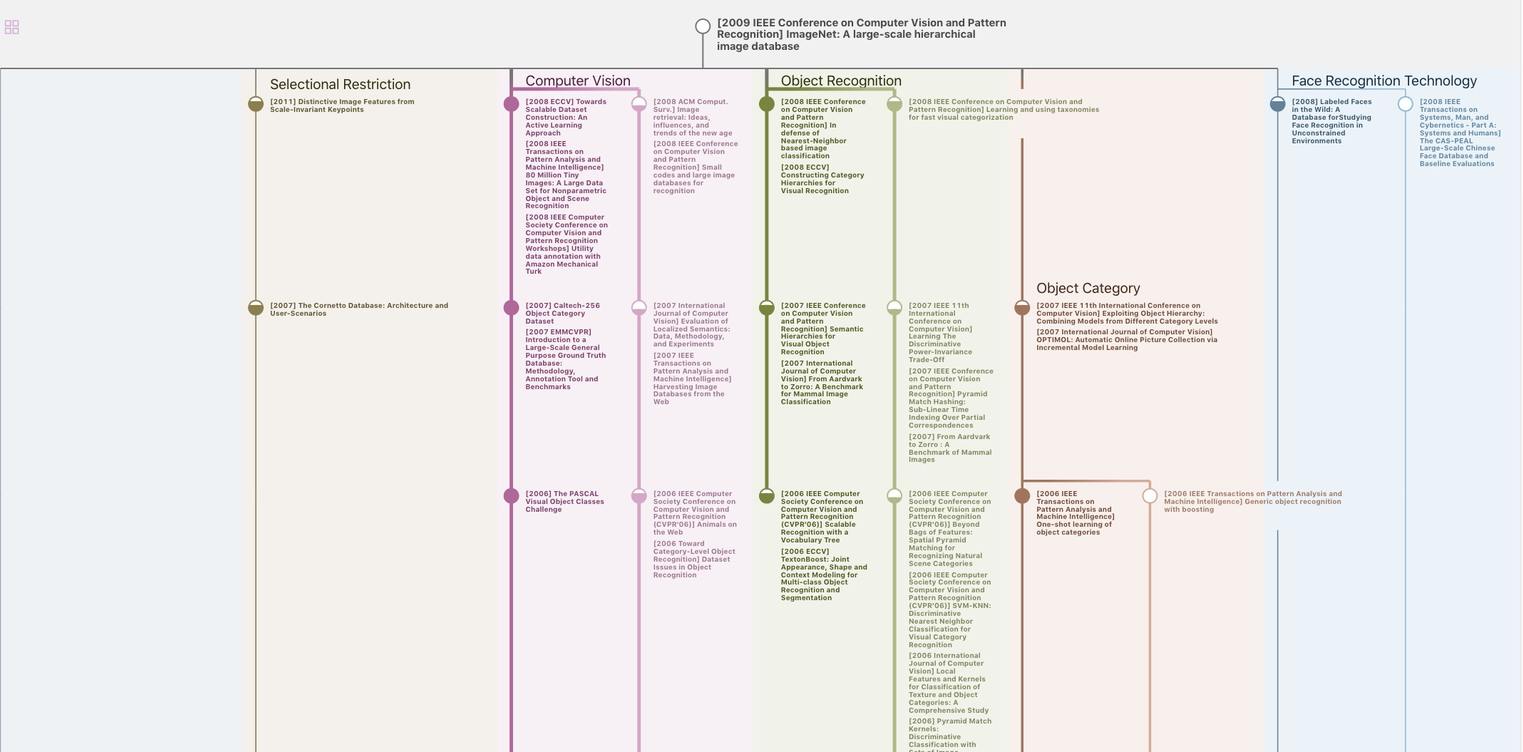
Generate MRT to find the research sequence of this paper
Chat Paper
Summary is being generated by the instructions you defined