Partial Label Learning Based on Disambiguation Correction Net With Graph Representation
IEEE Transactions on Circuits and Systems for Video Technology(2022)
摘要
Partial Label Learning (PLL) is a weakly supervised learning framework where each training instance is associated with more than one candidate label. This learning method is dedicated to finding out the true label for each training instance. Most of the current PLL algorithms directly disambiguate the candidate labels without correcting the disambiguated results, making the algorithms vulnerable to the influence of instances easily misjudged. In this paper, GraphDCN, an innovative disambiguation correction net with inductive graph representation learning model is proposed. GraphDCN consists of a disambiguation model and a correction model. For a given instance, the disambiguation model tries to fit its underlying ground-truth label through the candidate label distribution of the instances connected with the given one, while the correction model tries to maximize the distance between the disambiguated labels and non-candidate labels, and uses the label probability thresholds to correct the disambiguated labels that may be wrong. As the training goes on, both the disambiguation and correction models alternately and iteratively boost their performance. Moreover, when considering the implementation of the disambiguation model, a partial cross entropy formulation is proposed to estimate the ground-truth label loss by updating the ambiguity confidence matrix, which can be proved satisfying convergence in PLL. Simulation results reveal the overwhelming performance of GraphDCN.
更多查看译文
关键词
Partial label learning,GraphDCN,partial cross entropy,label probability threshold
AI 理解论文
溯源树
样例
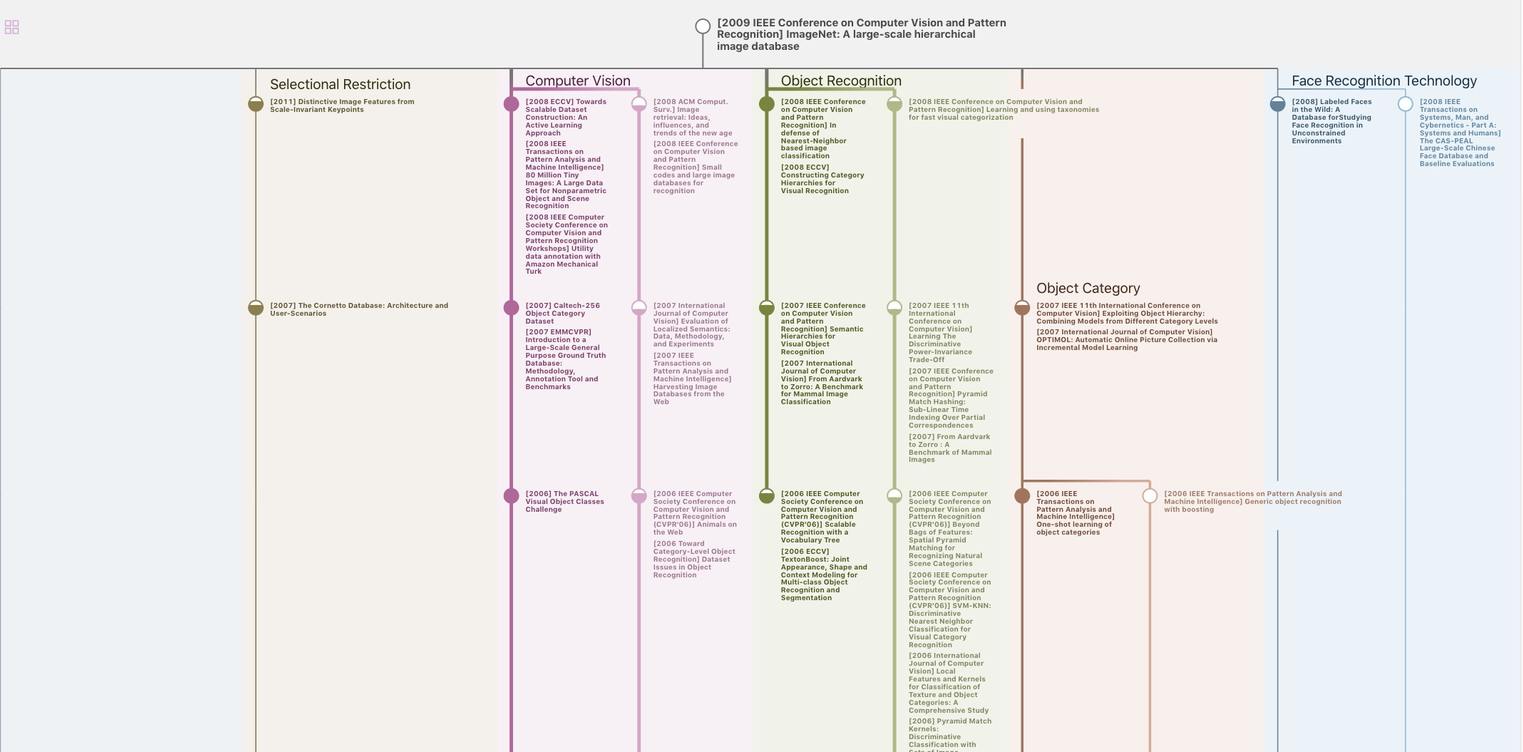
生成溯源树,研究论文发展脉络
Chat Paper
正在生成论文摘要