Self-Triggered Adaptive NN Tracking Control for a Class of Continuous-Time Nonlinear Systems With Input Constraints
IEEE Transactions on Systems, Man, and Cybernetics: Systems(2022)
摘要
This article develops a self-triggered adaptive neural network (NN) tracking controller for a class of continuous-time nonlinear systems, that is, input constrained and with unknown drift and input dynamics. Since the drift and input dynamics are both unknown, an NN is built within a self-triggered update paradigm to approximate the unknown tracking control. The error derivative used in the weight update algorithm is derived using a robust exact differentiator technique. To address input constraints, an auxiliary compensator is designed for the unimplemented control effort. Through rigorous Lyapunov analyses, we can guarantee that all the tracking and weight errors are uniformly ultimately bounded. Finally, to show the effectiveness of the proposed control performance, simulation results of a two-link robot are provided and analyzed.
更多查看译文
关键词
Adaptive tracking control,differentiator,input constraints,neural networks (NNs),self-triggered control
AI 理解论文
溯源树
样例
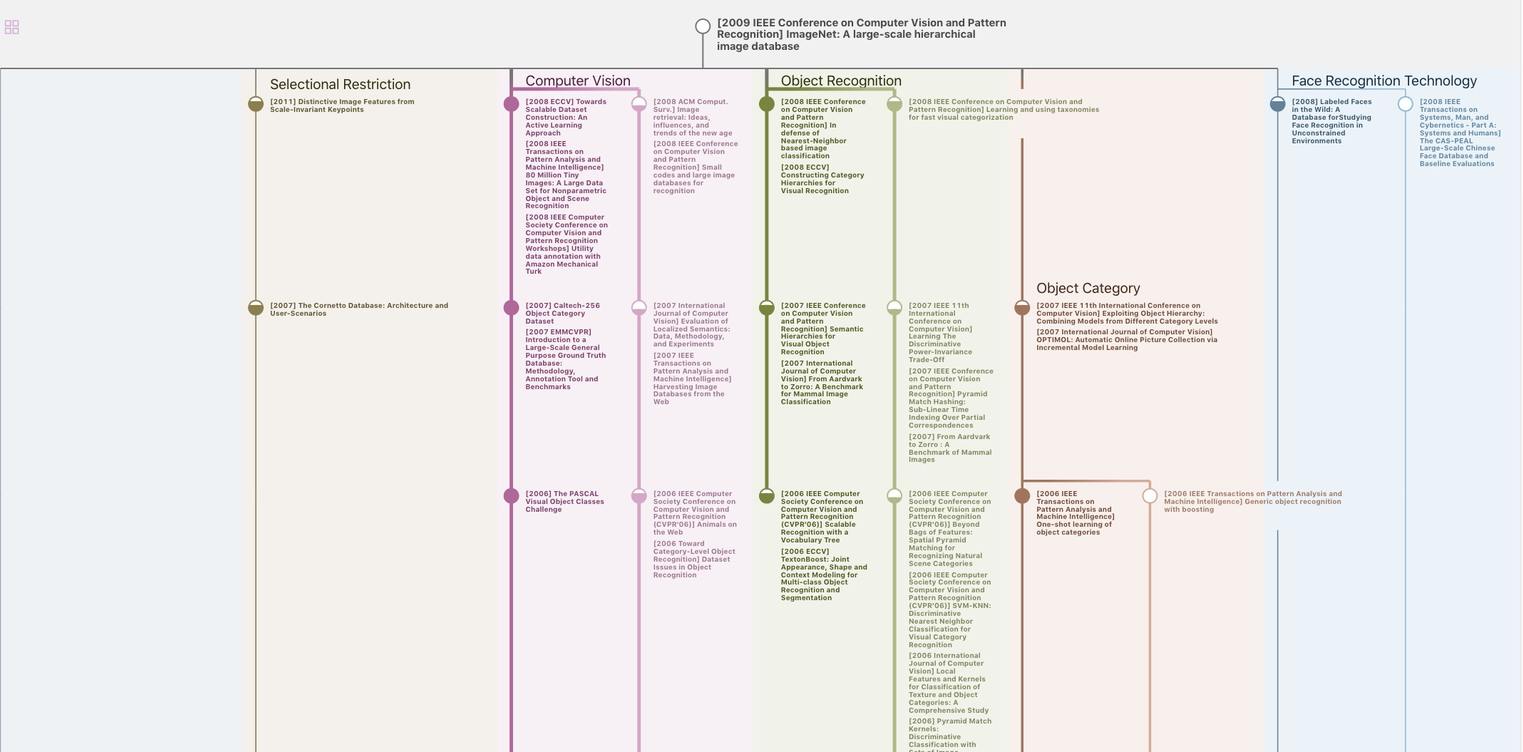
生成溯源树,研究论文发展脉络
Chat Paper
正在生成论文摘要