Integrating artificial intelligence and color Doppler US for automatic hemorrhage detection
internaltional ultrasonics symposium(2021)
摘要
Hemorrhage control has been identified as a priority focus area for the United States military because exsanguination is the most common cause of preventable death in battlefield. Non-compressible torso hemorrhage (NCTH) has high mortality rate and there are no available therapies for NCTH. New therapies, which include High Intensity Focused Ultrasound (HIFU) has emerged as a promising method for hemorrhage control as it can non-invasively cauterize bleeding tissue deep within the body without injuring uninvolved regions. A major challenge in the application of HIFU is the accurate targeting of therapeutic beam to the location of the bleed, requiring an expert sonographer to interpret images in real-time, currently limiting the utility of this therapy in remote environments. In this work, we investigated the use of an unsupervised anomaly detection network that learns a manifold of normal blood flow variability and subsequently identifies anomalous flow patterns that fall outside the learned manifold. As an initial feasibility study, we collected ultrasound color Doppler images of femoral arteries in an animal model of vascular injury ( $\mathrm{N}=5$ pigs). The images were pre-processed to mask out velocities in surrounding tissues and were subsequently cropped, resized, augmented and normalized. The network was trained on normotensive images from 4 pigs and tested on normotensive, immediately after injury and 10 minutes post-injury images of 1 other pig. The residual images or the reconstructed error maps show promise in detecting hemorrhages with 81% and 64% sensitivity immediately and 10 minutes post-injury respectively and 70% specificity.
更多查看译文
关键词
hemorrhage detection,color Doppler ultrasound,unsupervised anomaly detection,generative adversarial network,deep learning
AI 理解论文
溯源树
样例
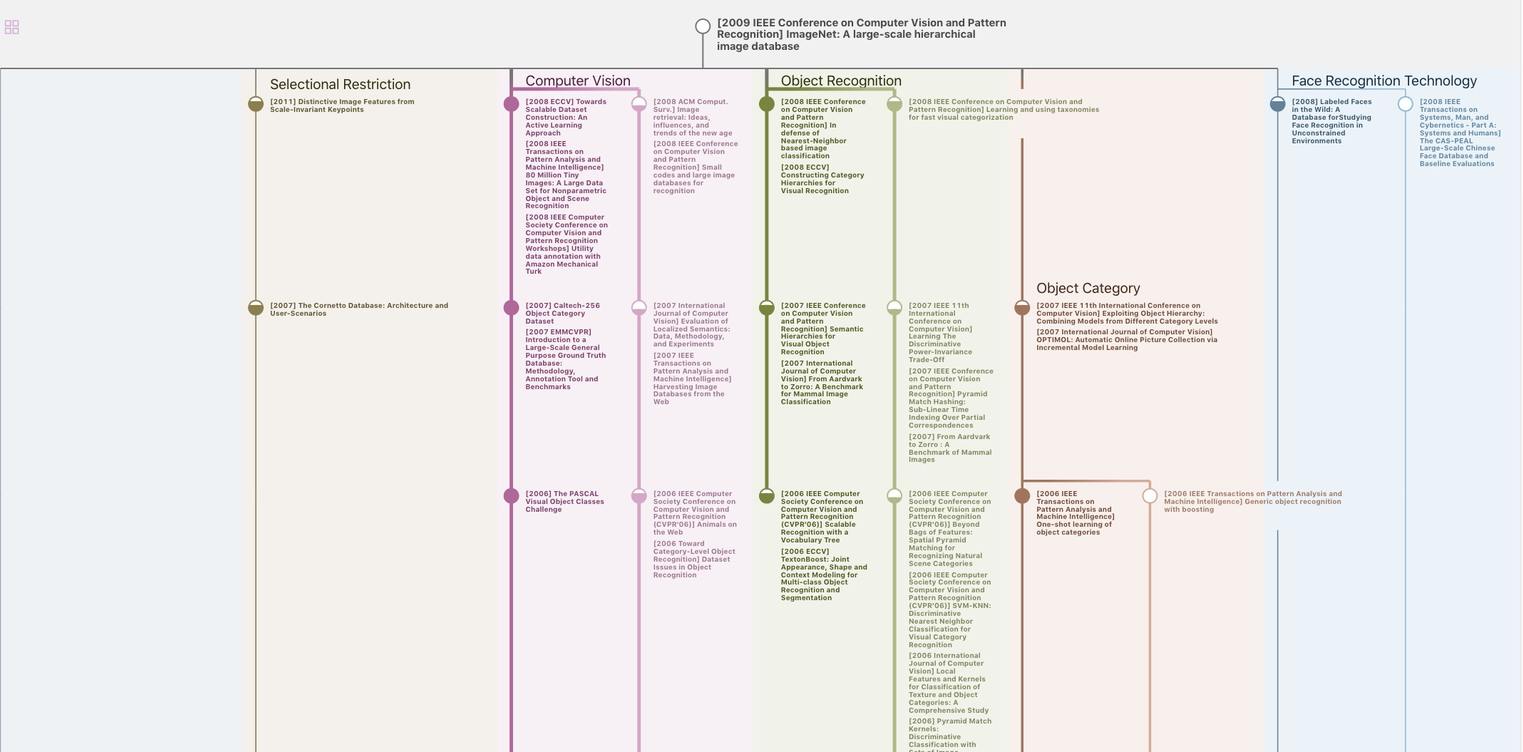
生成溯源树,研究论文发展脉络
Chat Paper
正在生成论文摘要