Reinforcement Learning for Energy Storage Optimization in the Smart Grid
2020 IEEE International Conference on Power Electronics, Smart Grid and Renewable Energy (PESGRE2020)(2020)
Abstract
In the existing electrical grid, there is a large demand supply mismatch which results in unutilized power potential. A demand based pricing approach can be achieved by implementing dynamic pricing in the smart grid. But such an approach can only be effective when the consumer has a mechanism to adapt their power usage based on the price of power. This paper looks into the implementation of Reinforcement Learning algorithms- specifically, Q-learning and SARSA [1] - to control batteries to optimize energy storage at a larger scale. We also demonstrate a non-linear algorithm for “bucketizing” which allows the incorporation of states with highly uneven distributions of visit frequency into the model.
MoreTranslated text
Key words
reinforcement learning,smart grid,Q-learning,battery agent
AI Read Science
Must-Reading Tree
Example
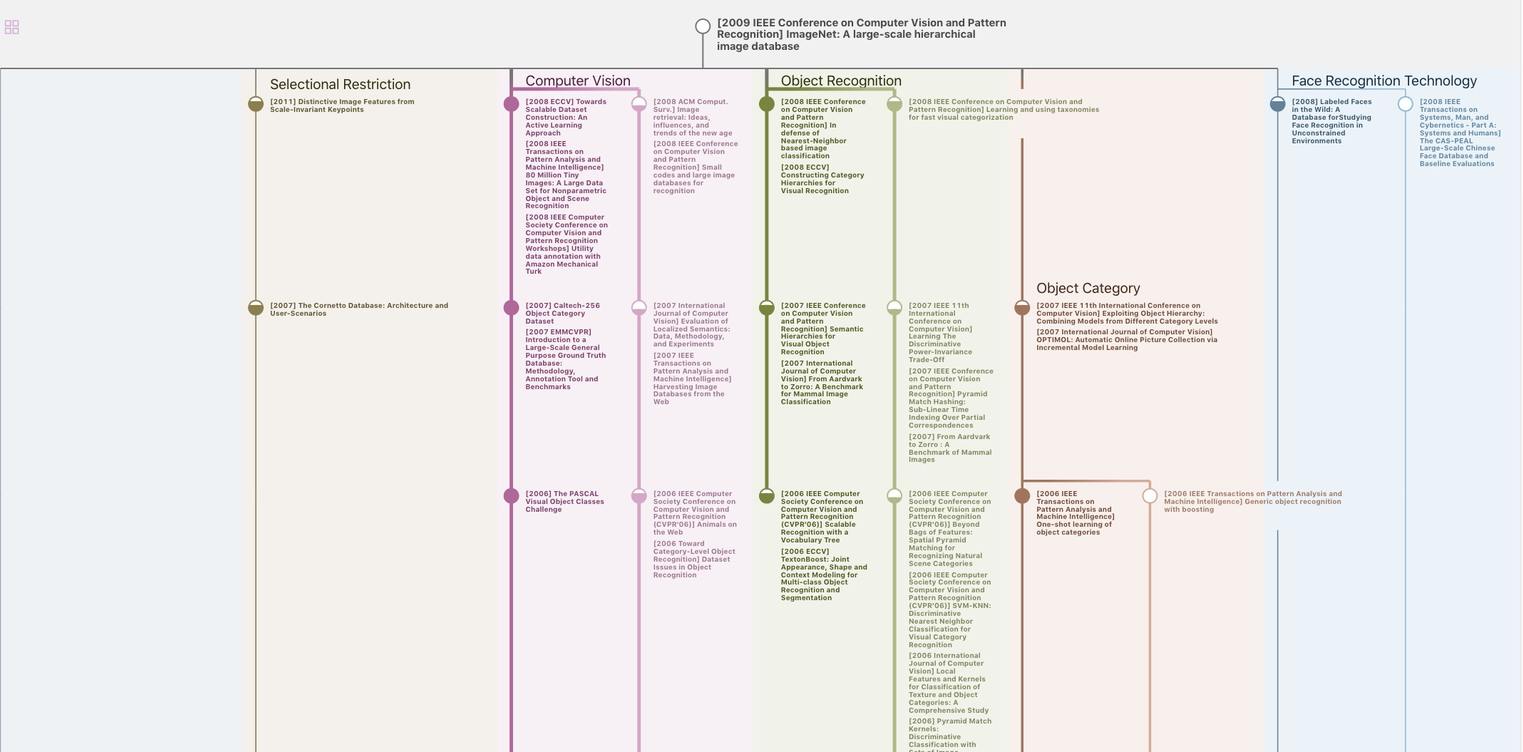
Generate MRT to find the research sequence of this paper
Chat Paper
Summary is being generated by the instructions you defined