Effective Detection of Compensated Cirrhosis Using Machine Learning
Social Science Research Network(2020)
摘要
Background: Cirrhosis is often diagnosed once complications such as ascites, encephalopathy and variceal bleeding have occurred. Current non-invasive tests to detect cirrhosis have limited performance, with many patients falling into the indeterminate category.
Objectives: To identify patients with well-compensated liver cirrhosis of all-causes using machine learning algorithms (MLAs) trained on routinely measured clinical and laboratory parameters.
Design, Setting, and Participants: Retrospective, cross-sectional study on patients with F0, F1, and F4 fibrosis staged via biopsy at the Toronto Liver Clinic, validated on 508 patients with F0 to F4 fibrosis staged via biopsy locally as well as at the McGill University Health Centre. Patients with decompensated cirrhosis were excluded. We trained 7 MLAs to identify patients with compensated cirrhosis. Performance was benchmarked against the Aspartate aminotransferase (AST)-to-Platelet Ratio Index (APRI), Fibrosis-4 index (FIB-4), nonalcoholic fatty liver disease (NAFLD) fibrosis score (NFS), Transient Elastography (TE), and an independent panel of 5 experts.
Results: The best MLA achieved a cross-validation area under the receiver operating characteristic curve (AUROC) of 0.88 for identifying patients with well-compensated cirrhosis, with less than 8% indeterminate classifications. The MLA achieved higher or comparable AUROC to FIB-4 on both validation sets with 20% fewer indeterminate classifications. Additionally, we show that by altering cutoffs, the MLA outperformed expert hepatologists and the NFS score and achieved superior specificity to transient elastography.
Conclusion: In this study, we have demonstrated that an MLA outperforms non-imaging based methods in detecting well-compensated cirrhosis across multiple etiologies of liver disease. Our MLA was superior to APRI, FIB-4, and NFS with significantly fewer indeterminate classifications, while achieving performance comparable to an independent panel of experts. MLAs using routinely collected data could flag patients with well-compensated liver cirrhosis among patients with chronic liver disease, allowing intervention prior to onset of decompensation and associated complications.
Funding Statement: This study was supported by ICES, which is funded by an annual grant from the Ontario Ministry of Health and Long-Term Care (MOHLTC).
Declaration of Interests: We have no competing interests to declare.
Ethics Approval Statement: Ethics approval was obtained from the Research Ethics Boards at the University Health Network, SickKids Research Institute, and MUHC.
更多查看译文
关键词
compensated cirrhosis,machine learning,effective detection
AI 理解论文
溯源树
样例
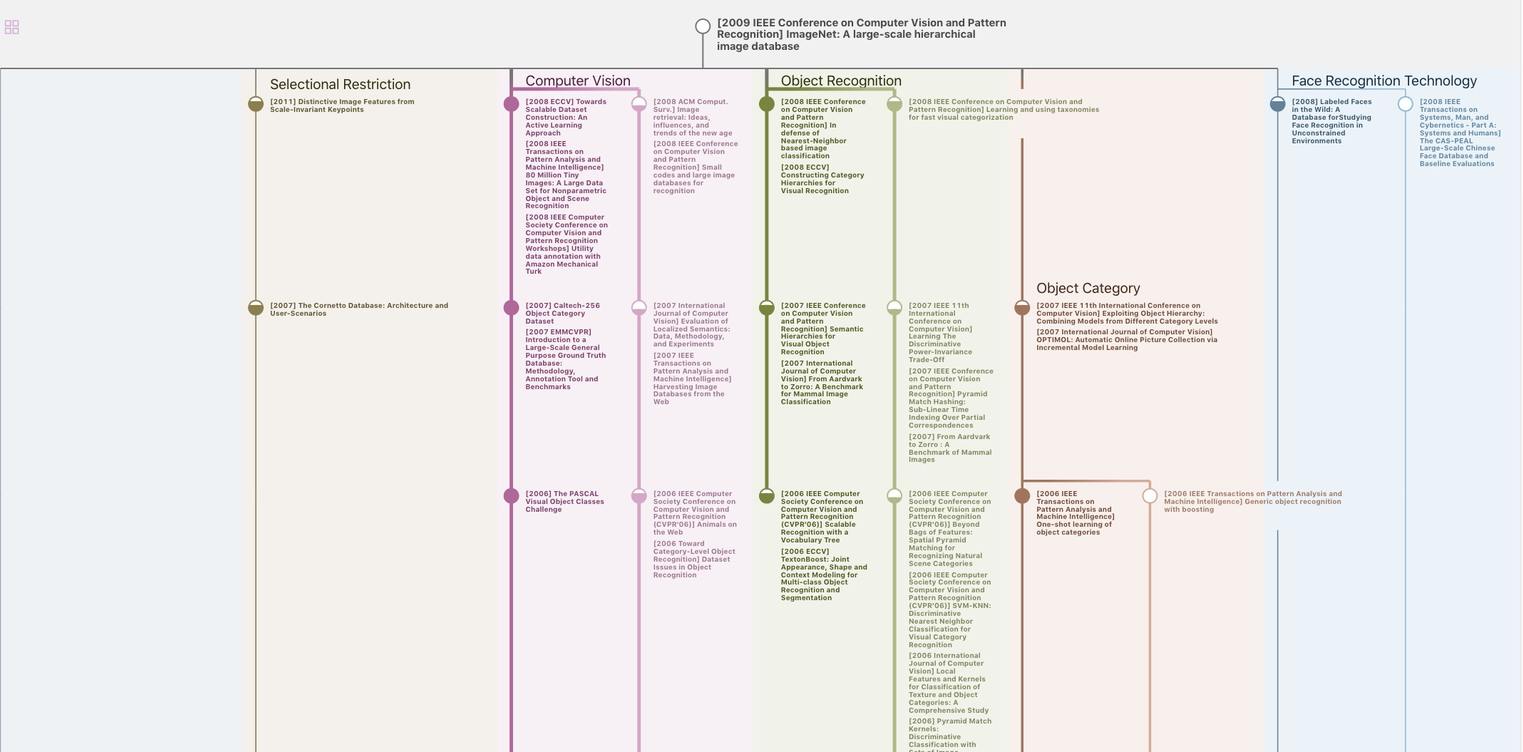
生成溯源树,研究论文发展脉络
Chat Paper
正在生成论文摘要