Decision-Driven Regularization: A Blended Model for Predict-then-Optimize
Social Science Research Network(2021)
摘要
In contextual optimization, the decision-maker seeks optimal decisions to minimize a cost, that varies based on observed features. This context is common in many business applications ranging from on-demand delivery and retail operations to portfolio optimization and inventory management. In this paper, we study the predict-then-optimize approach, which first learns how outcomes result from the features, and then selects optimal decisions based on these outcomes. We identify in the literature an ambiguity in the definition of the cost function, arising out of the lack of access to the true outcomes. To address this, we propose a blended predict-then-optimize framework that may lead to biased predictions on the outcome, but tractably incorporates the optimization problem into the prediction stage. This is achieved by a decision-driven regularization. We critically show that there are three perspectives, from which the predict-then-optimize problem may be approached, namely the regularization, the robust optimization, and the regret minimization approaches; and prove that these perspectives are equivalent to or can be naturally approximated to arrive at our proposed model. As a consequence, our framework generalizes models such as SPO+ in Elmachtoub and Grigas (2020) and JERO in Zhu et al. (2020). Building on our framework, we propose hybrid models that we show numerically to outperform SPO+ under low mis-specification.
更多查看译文
AI 理解论文
溯源树
样例
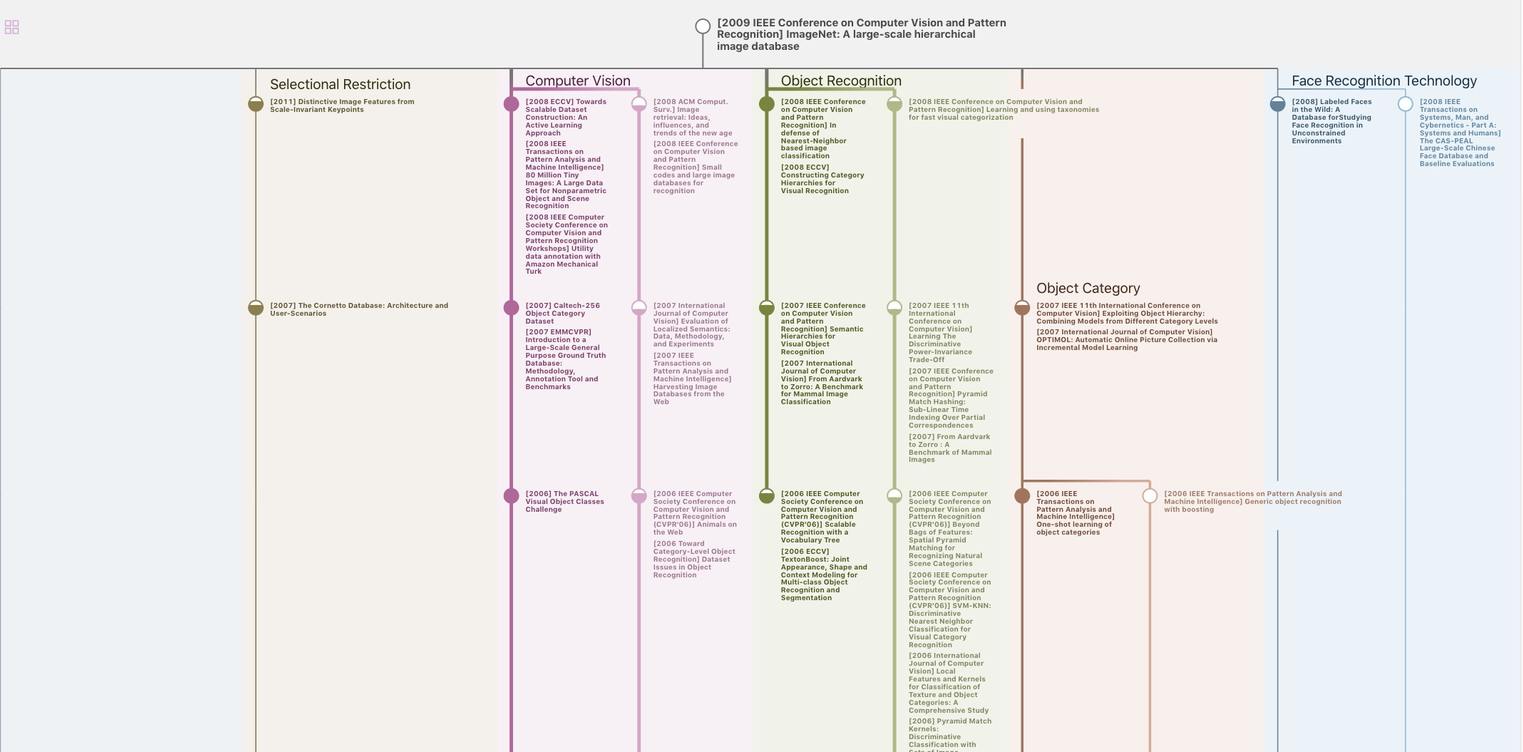
生成溯源树,研究论文发展脉络
Chat Paper
正在生成论文摘要