Unsupervised clustering of coral reef fish calls
Journal of the Acoustical Society of America(2020)
摘要
An unsupervised process is proposed for clustering and identifying fish calls in an acoustically active coral reef soundscape. First, potentially localizable acoustic events were detected on three directional autonomous seafloor acoustic recorders (DASARs), sampled at 1 kHz, using an automatic directional detector. A maximum likelihood localization algorithm was used to remove unlocalizable events. For each localizable event, standard acoustic metrics were extracted from the spectrogram and timeseries, while user-agnostic latent features were extracted from the spectrograms by an undercomplete convolutional autoencoder (CAE) neural network. Unsupervised clustering methods, including K-means and agglomerative hierarchical, identified distinct acoustic classes from both feature sets. The unsupervised clustering process was used to analyze data collected near a Hawaiian coral reef in February 2020. During a 24-h period, about 1 event per second was detected, with diel variation in the number of detected events. Compared to a hand labeled test set, the unsupervised clustering process with standard acoustic metrics was 91% accurate at identifying fish call trains, which were associated with dusk chorusing, as opposed to single pulse calls or Humpback whale sound. The tradeoffs between physical features and CAE latent features for unsupervised call discovery are discussed.
更多查看译文
关键词
unsupervised clustering,fish
AI 理解论文
溯源树
样例
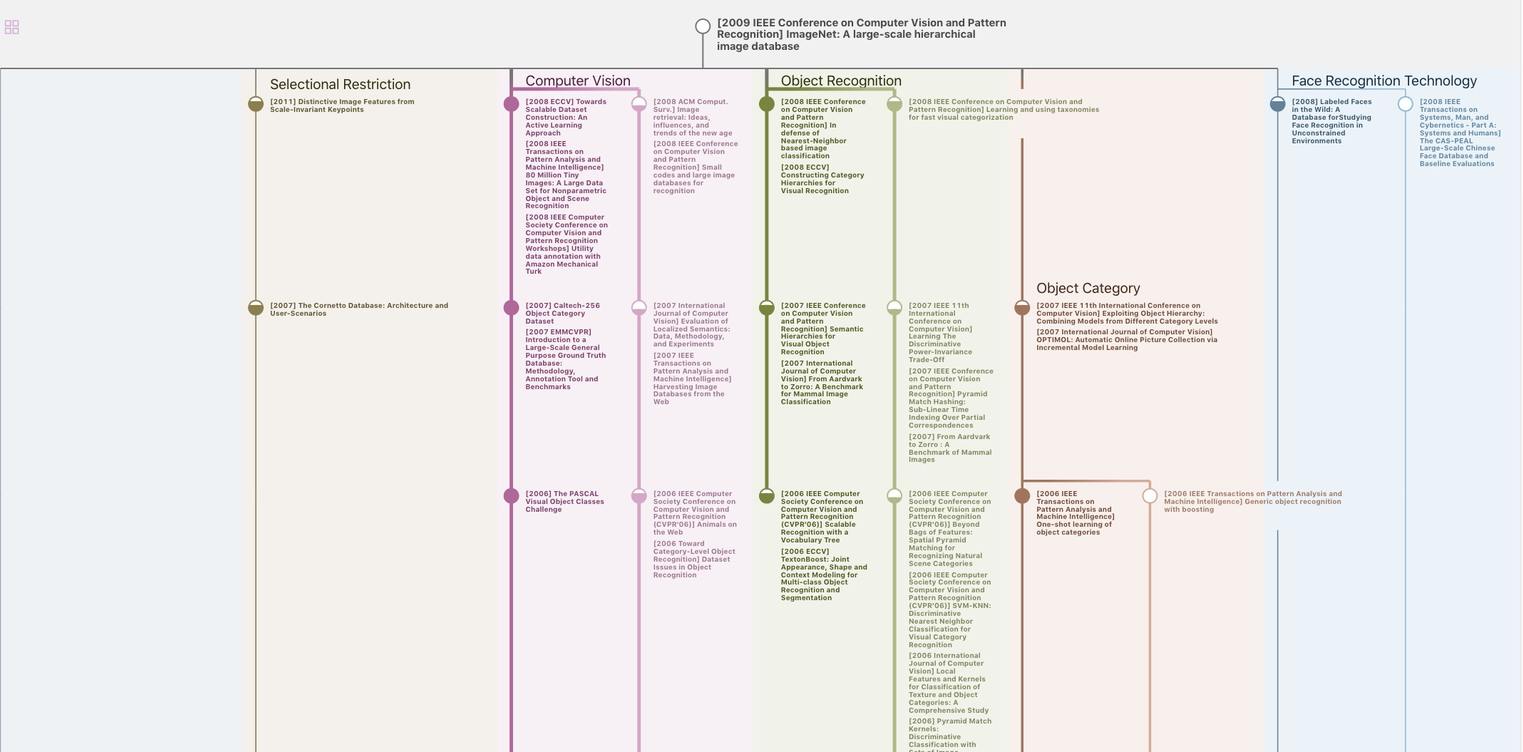
生成溯源树,研究论文发展脉络
Chat Paper
正在生成论文摘要