Testing Mean and Covariance Structures with Reweighted Least Squares
STRUCTURAL EQUATION MODELING-A MULTIDISCIPLINARY JOURNAL(2022)
摘要
Chi-square tests based on maximum likelihood (ML) estimation of covariance structures often incorrectly over-reject the null hypothesis: Sigma = Sigma(theta) when the sample size is small. Reweighted least squares (RLS) avoids this problem. In some models, the vector of parameter must contain means, variances, and covariances, yet whether RLS also works in mean and covariance structures remains unexamined. This research extends RLS to mean and covariance structures, evaluating a generalized least squares function with ML parameter estimates. A Monte Carlo simulation study was carried out to examine the statistical performance of ML vs RLS with multivariate normal data. Based on empirical rejection frequencies and empirical averages of test statistics, this study shows that RLS performs much better than ML in mean and covariance structure models when sample sizes are small, whereas it does not perform better than ML to reject misspecified models.
更多查看译文
关键词
Mean and covariance structure,reweighted least squares,goodness-of-fit test,structural equation models,small sample size
AI 理解论文
溯源树
样例
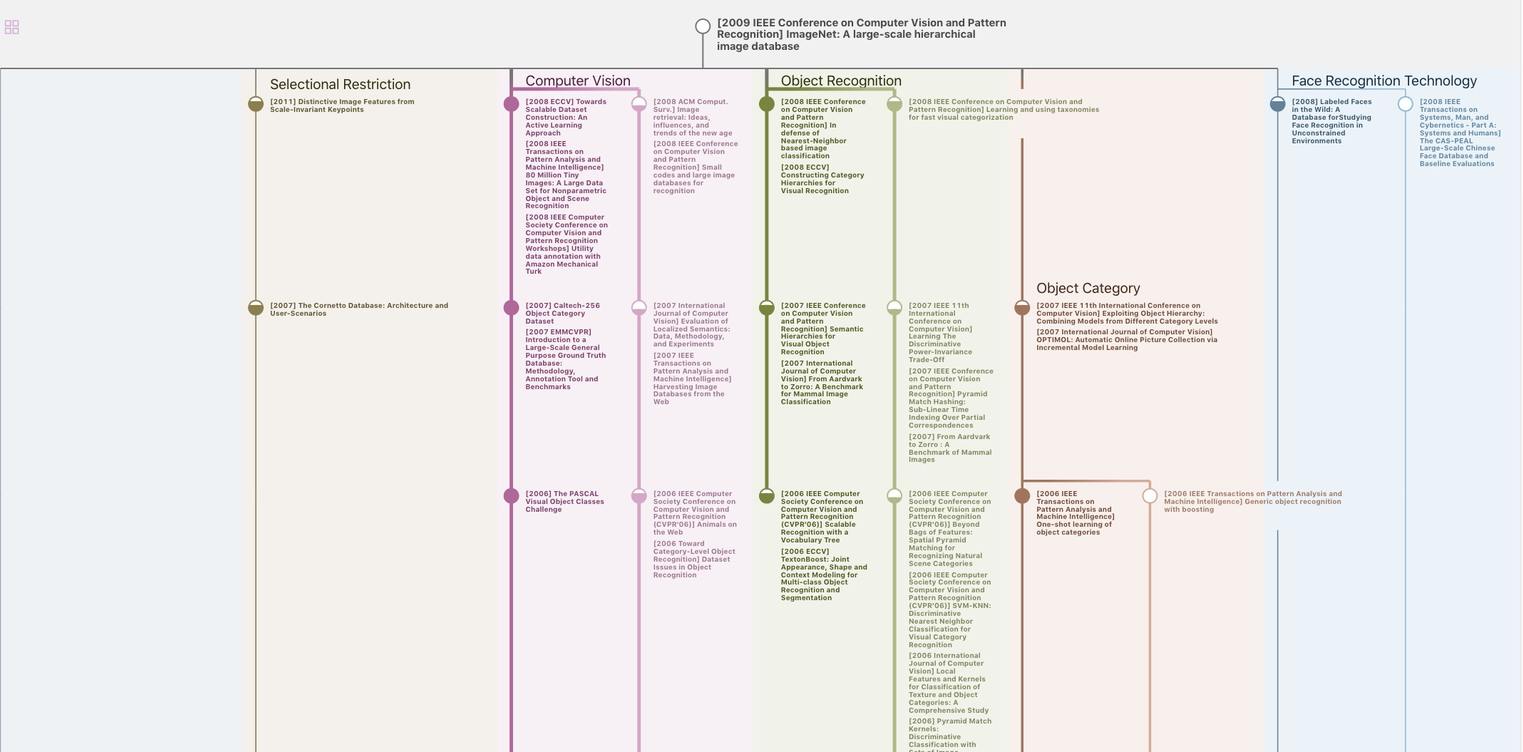
生成溯源树,研究论文发展脉络
Chat Paper
正在生成论文摘要