Timing it Right: Balancing Inpatient Congestion versus Readmission Risk at Discharge
Social Science Research Network(2019)
摘要
When to discharge a patient plays an important role in hospital patient flow management as well as the quality of care and patient outcomes. In this work, we develop and implement a data-integrated decision support framework to aid hospitals in managing the delicate balance between readmission risk at discharge and ward congestion. We formulate a large-scale Markov Decision Process (MDP) that integrates a personalized readmission prediction model to dynamically prescribe both how many and which patients to discharge on each day. Due to patient heterogeneity and the fact that length-of-stay is not memoryless, the MDP suffers the curse of dimensionality. We leverage structural properties and analytical solutions for a special cost setting to transform the MDP into a univariate optimization; this leads to a novel, efficient dynamic heuristic. Further, for our decision framework to be implementable in practice, we build a unified prediction model that integrates several statistical methods and provides key inputs to the decision framework; existing off-the-shelf readmission prediction models alone could not adequately parametrize our decision support.
Through extensive counterfactual analyses, we demonstrate the value of our discharge decision tool over our partner hospital's historical discharge behavior. We also obtain generalizable insights by applying the tool to a broad range of hospital types through a high-fidelity simulation. Lastly, we showcase an implementation of our tool at our partner hospital, to demonstrate broader applicability through our framework's plug-and-play design for integration with general hospital data-systems and workflows.
更多查看译文
AI 理解论文
溯源树
样例
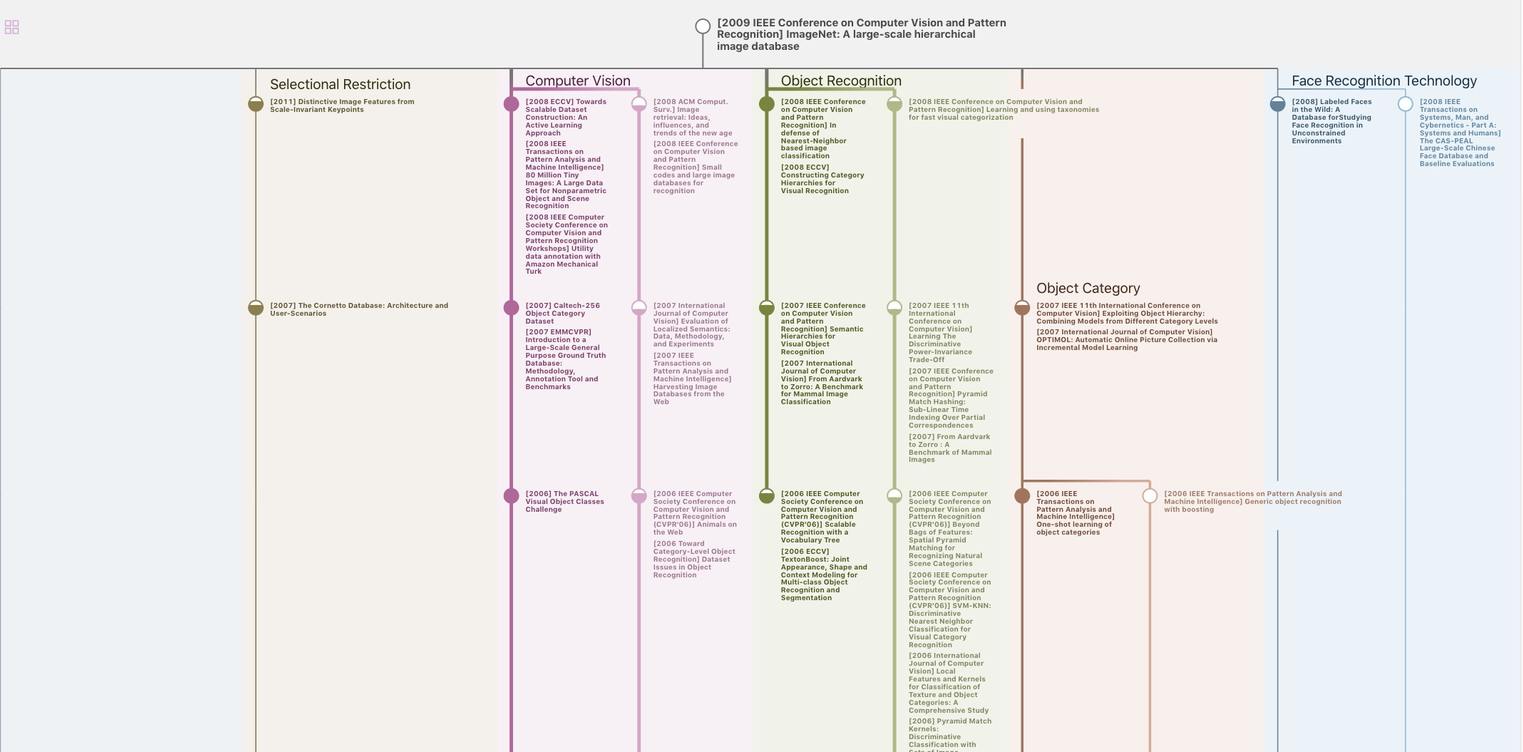
生成溯源树,研究论文发展脉络
Chat Paper
正在生成论文摘要