Deep Co-Image-Label Hashing for Multi-Label Image Retrieval
IEEE Transactions on Multimedia(2022)
摘要
Deep supervised hashing has greatly improved retrieval performance with the powerful learning capability of deep neural network. In multi-label image retrieval, existing deep hashing simply indicates whether two images are similar by constructing a similarity matrix. However, it ignores the dependency among multiple labels that has been shown important in multi-label application. To fulfill this gap, this paper proposes Deep Co-Image-Label Hashing (DCILH) to discover label dependency. Specifically, DCILH regards image and label as two views, and maps the two views into a common deep Hamming space. DCILH proposes to learn prototype for each label, and preserve similarity among images, labels, and prototypes. To exploit label dependency, DCILH further employs the label-correlation aware loss on the predicted labels, such that predicted output on positive label is enforced to be larger than that on negative label. Extensive experiments on several multi-label benchmarks demonstrate the proposed DCILH outperforms state-of-the-art deep supervised hashing on large-scale multi-label image retrieval.
更多查看译文
关键词
Hashing,multi-label,image retrieval
AI 理解论文
溯源树
样例
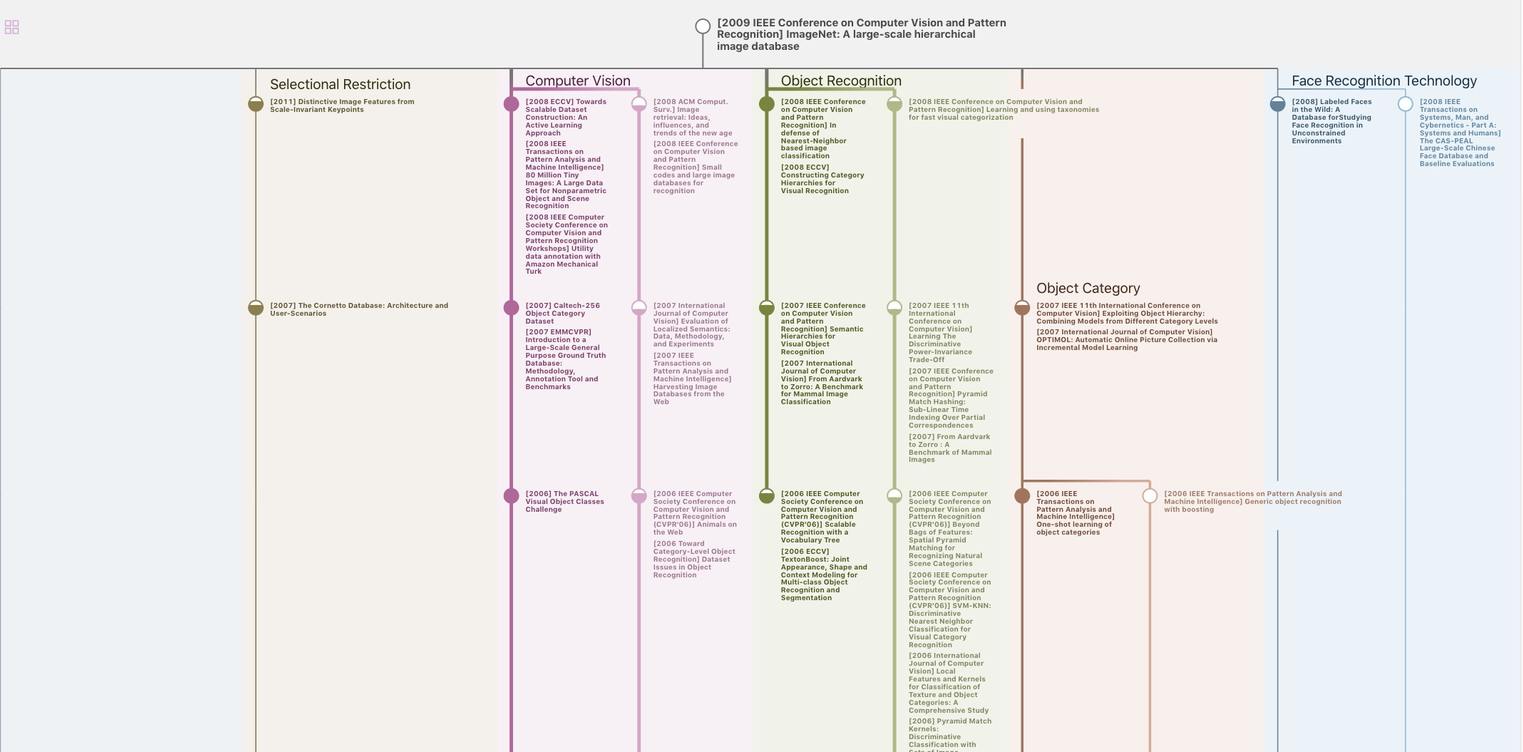
生成溯源树,研究论文发展脉络
Chat Paper
正在生成论文摘要