A Hybrid Prognostic Approach Based on UKF and Optimized RBF
2019 IEEE 8th Data Driven Control and Learning Systems Conference (DDCLS)(2019)
摘要
Prognostics and health management (PHM) is of great significance for complex systems. Making full use of the known information of systems is an urgent problem to be solved to ensure the efficient operation. So, utilizing both of the mechanism model and data generated during the operation could predicting as precisely as possible. In this paper, a hybrid approach based on unscented Kalman filter (UKF) and optimized Radial Basis Function (RBF) neural network is proposed. Firstly, UKF is utilized as model-based method to obtain the initial prognosis. Then, a neural network based on Radial Basis Function (RBF) intergrated with K-means clustering algorithm (K-means-RBF) is built and trained by the deviation series which is emerged in the UKF modeling process. Finally, initial prognosis is corrected with the predicted deviation. The lithium-ion battery data set of Maryland University is employed to verify the effectiveness of this given approach. The remaining useful life (RUL) of battery is predicted. The experimental results show that the prognosis of the hybrid approach is credible. The accuracy of appended data-driven method is higher than that of single source algorithm.
更多查看译文
关键词
Fault prediction,Data-driven,Radial Basis Function network,K-means clustering,Remaining useful life
AI 理解论文
溯源树
样例
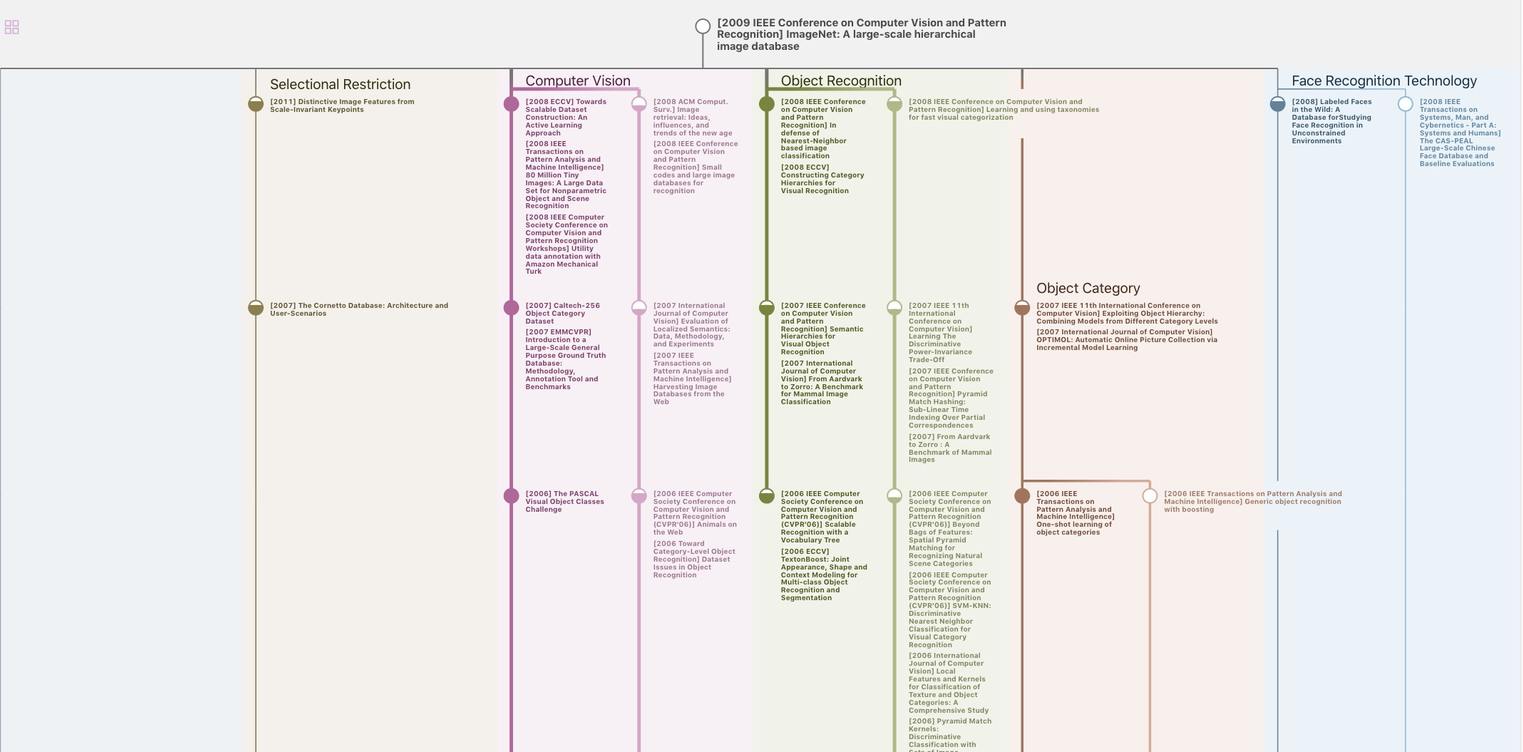
生成溯源树,研究论文发展脉络
Chat Paper
正在生成论文摘要