Improving Classification Performance of Skewed Biomass Data
IOP conference series(2021)
摘要
The importance of biomass in power generation towards the attainment of a sustainable bio-economy is well documented. But, like most real-life data streams, biomass data are characterised by class imbalances and are often tagged as skewed. Therefore, there is a need for a model which can accurately predict the biomass data classes while correctly identifying the minority data class considering the imbalances within the classes. In this study, RUSBoost is introduced to alleviate this challenge. RUSBoost provides dual benefits of data sampling and boosting, therefore proving an easy and proficient method for enhancing classification performance during training and testing. This study proposes a RUSBoost model to classify biomass dataset from various sources. The main objective is to optimally predict the classes, therefore this study only focuses on the RUSBoost algorithm while analysing how it fares in predicting the classes. The performance assessment was based on several know indices which are accuracy (65.2%), error rate (34.8%), sensitivity (74.8%), specificity (94.8%) FPR (5.2%), Kappa statistics (72.3%), G-mean (84.21%) and computation time (12.4 secs). It was concluded that learning based on RUSBoost is satisfactory for skewed data class though further work can improve the overall accuracy.
更多查看译文
AI 理解论文
溯源树
样例
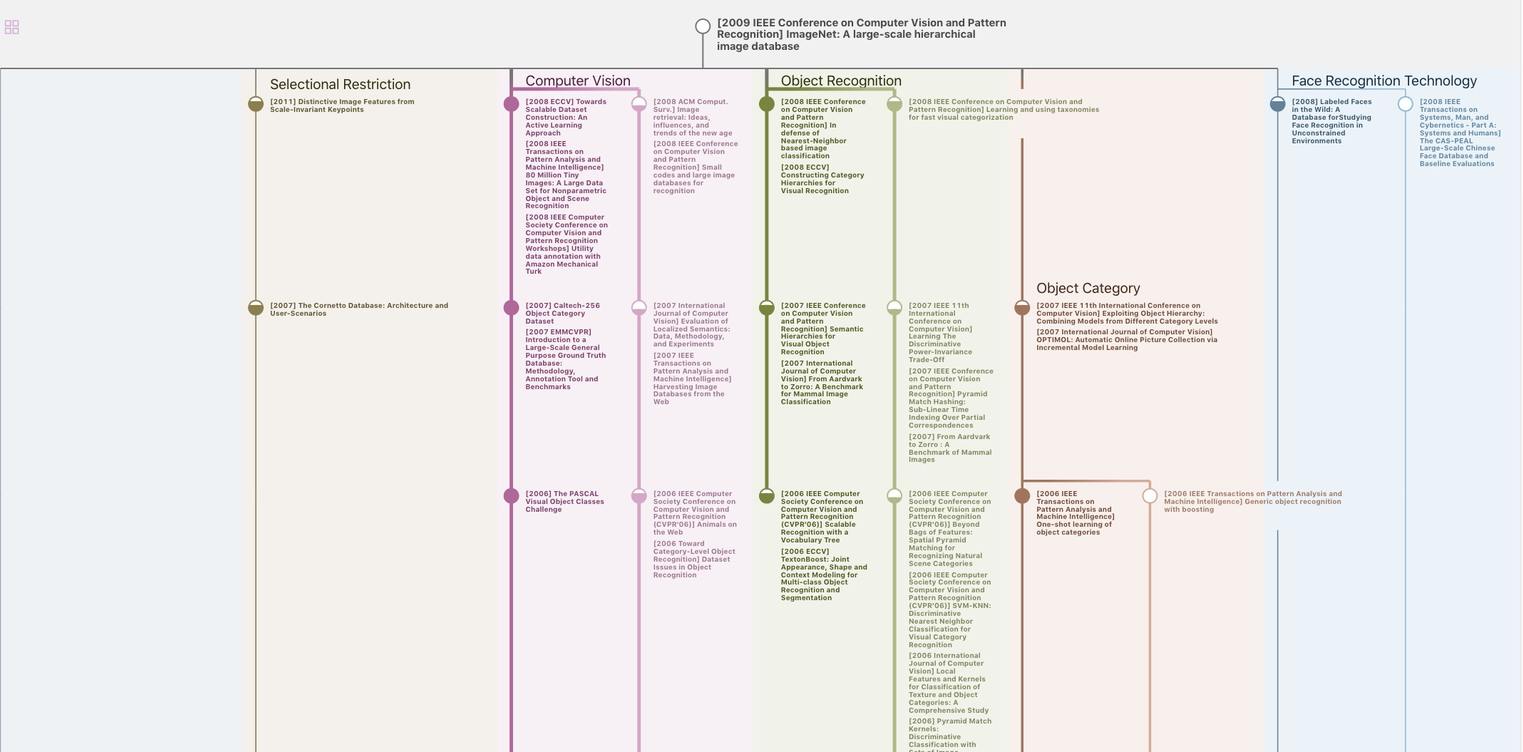
生成溯源树,研究论文发展脉络
Chat Paper
正在生成论文摘要