MINERVA: Learning the Rules of HLA Class I Peptide Presentation in Tumors with Convolutional Neural Networks and Transfer Learning
Social Science Research Network(2020)
摘要
Neoantigens act as surrogates for T cell immunoreactivity and have been shown to correlate with patients’ response to immune checkpoint inhibitors (ICI). Here, we have developed a multi-allele convolutional neural network-based model, MINERVA, for predicting HLA class I (HLA-I) presentation of tumor antigenic peptides using transfer learning with immunopeptidome data from cancer patients. We show that MINERVA recapitulates physicochemical properties of naturally presented peptides that are relevant to tumor biology and is more sensitive to tumor-derived HLA presented peptides than existing algorithms. Without a priori knowledge provided to MINERVA, the model was able to learn the determinants of peptide-HLA presentation and we utilized the learnt features to understand the impact of mutations on neoantigen presentation. Furthermore, we show that the diversity of tumor peptides being presented by a pair of alleles are significantly correlated with HLA sequence divergence. In conclusion, MINERVA is an open source software that can greatly facilitate tumor neoantigen discovery and guide cancer immunotherapies.
更多查看译文
AI 理解论文
溯源树
样例
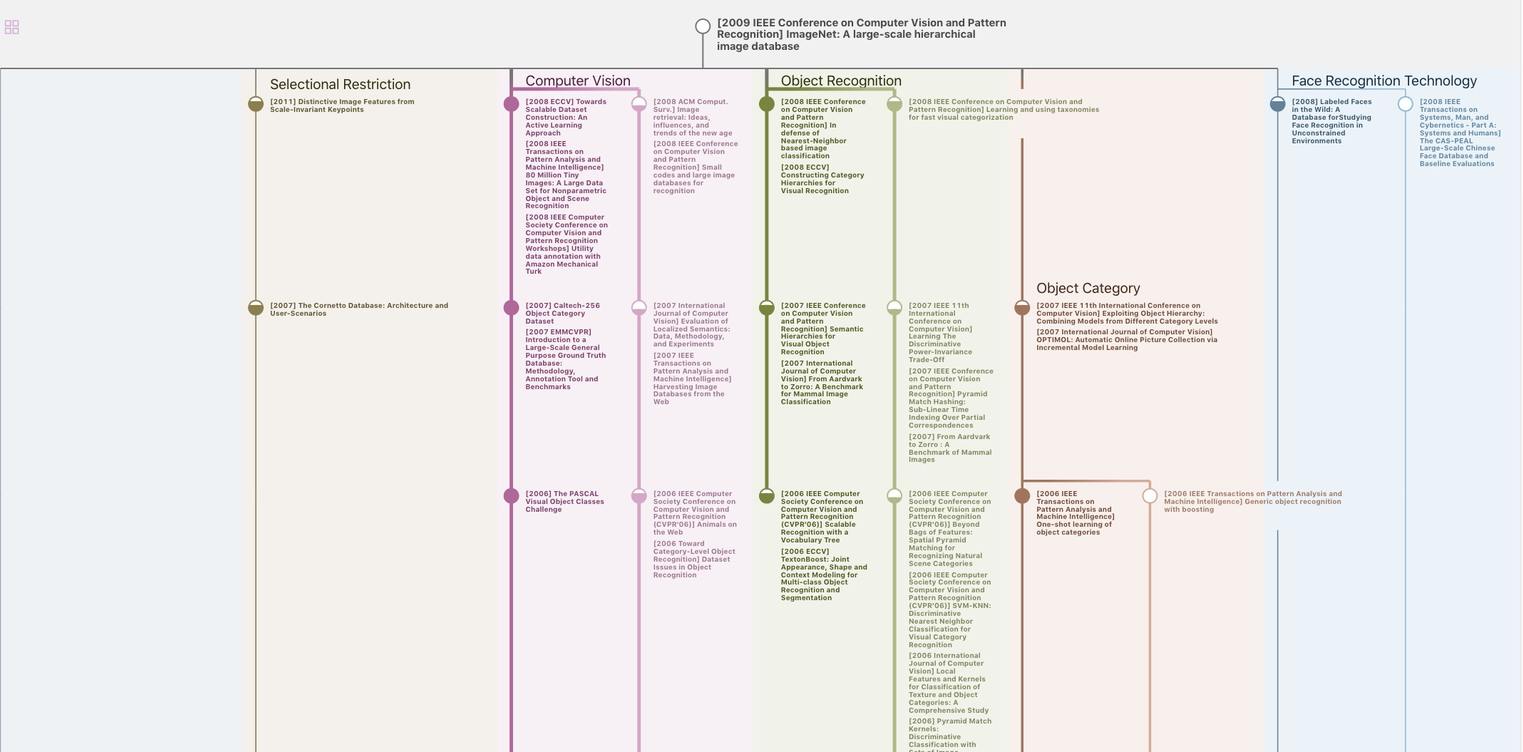
生成溯源树,研究论文发展脉络
Chat Paper
正在生成论文摘要