Unsupervised Domain-Invariant Feature Learning for Cloud Detection of Remote Sensing Images
IEEE TRANSACTIONS ON GEOSCIENCE AND REMOTE SENSING(2022)
摘要
The detection of clouds in remote sensing (RS) images is an important task, and convolutional neural networks (CNNs) have been used to perform it. However, supervised cloud detection CNNs rely heavily on a large number of samples annotated at pixel level to tune their parameter. Annotating RS images is a labor-intensive procedure and requires expert-level human knowledge. To reduce the labeling cost, we propose an unsupervised domain adaptation (UDA) approach to enable the model trained on labeled source satellite images to generalize to unlabeled target satellite images. Specifically, we propose a fine-grained feature alignment (FGFA) domain adaptation strategy that encourages a cloud detection network to extract domain-invariant representations, which improves the accuracy of cloud detection in unlabeled target satellite images. The proposed FGFA strategy consists of two steps: 1) fine-grained class-relevant feature selection based on an attention-guided mechanism and 2) class-relevant feature alignment (FA) based on a proposed grouped FA approach. Experimental results on the "Landsat-8 -> ZY-3" and "GF-1 -> ZY-3" domain adaptation tasks demonstrate the effectiveness of our method and its superiority to existing state-of-the-art UDA approaches.
更多查看译文
关键词
Cloud detection,domain-invariant feature,fine-grained feature alignment (FGFA),remote sensing (RS) imagery
AI 理解论文
溯源树
样例
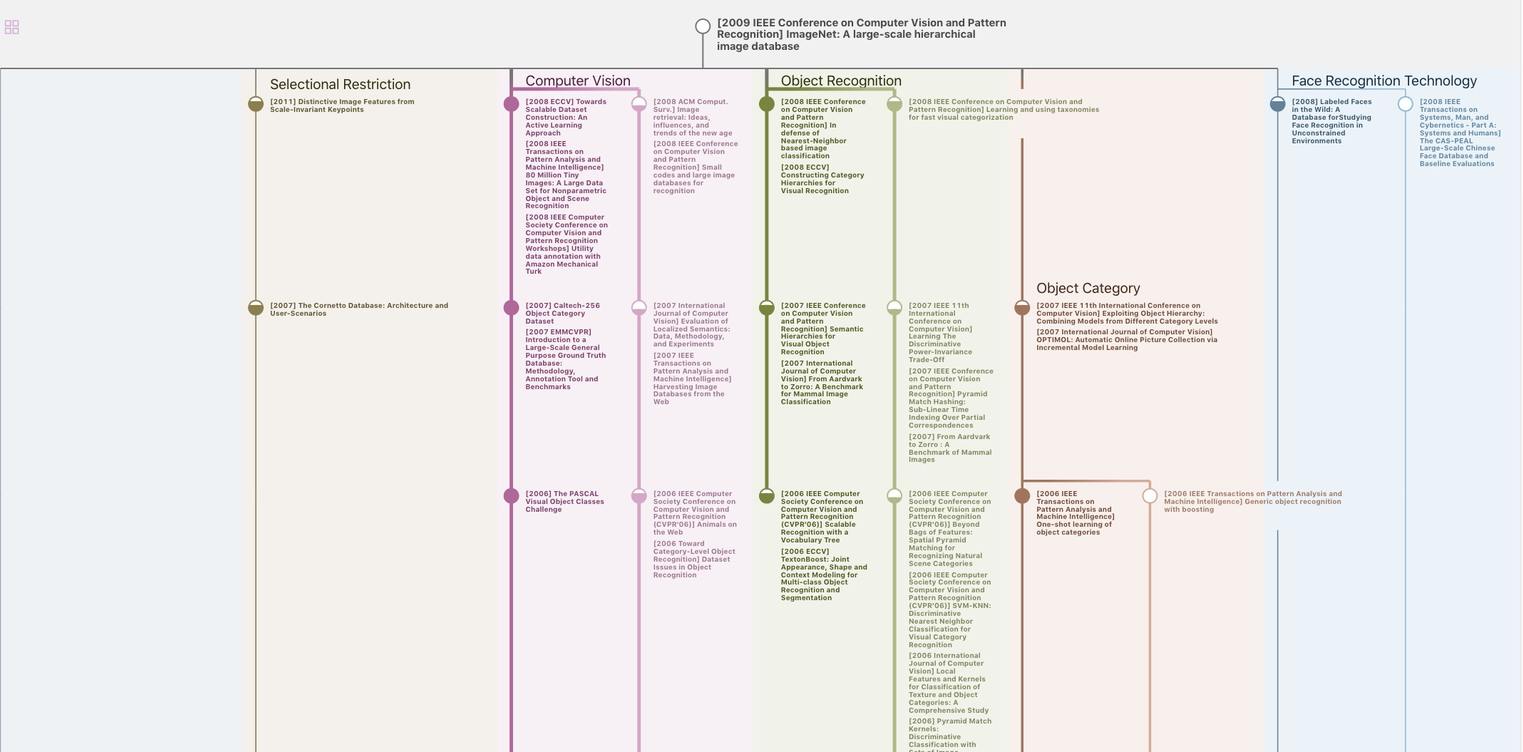
生成溯源树,研究论文发展脉络
Chat Paper
正在生成论文摘要