Proyección del precio de criptomonedas basado en Tweets empleando LSTM
Ingeniare. Revista chilena de ingeniería(2019)
摘要
espanolEl modelamiento y prediccion de series temporales constituye una tarea ardua y esencial para los procedimientos de optimizacion financiera. Numerosos estudios han sido elaborados con la finalidad de reducir la incertidumbre del inversor, mediante el pronostico de precio de monedas y acciones. Sin embargo, el surgimiento de un nuevo tipo de monedas con caracteristicas propias, conocidas como cryptocurrencies, plantea retos adicionales. En este sentido, el paper plantea analizar en que medida las publicaciones en las redes sociales pueden capturar las expectativas colectivas de los inversores, y afectar el valor futuro de la moneda. Nuestro objetivo es pronosticar el desempeno diario de un mercado en base a dos componentes: aquellos que definen el comportamiento de la criptomoneda en si (volumen, valor de apertura, valor de cierre, valor maximo y valor minimo) y las expectativas e interacciones del entorno, obtenidas de los tweets recolectados. Para ello, proponemos el uso de un tipo de red neuronal recurrente, conocida como Long Short Term Memory (LSTM). La metodologia empleada para el preprocesamiento de los datos y la aplicacion de esta tecnica de pronostico de series temporales nos permite obtener una prediccion con un Error Porcentual Absoluto Medio de 34.92%; lo que indica que la representacion de la variable de percepcion en redes social no ha sido la pertinente y, por lo tanto, motiva nuevos trabajos con la finalidad de modelar esta variable mediante el uso de otras tecnicas de NLP. EnglishThe modeling and prediction of time series is an arduous and essential task for financial optimization procedures. Numerous studies have been carried out to reduce investor uncertainty, by forecasting the price of currencies and shares. However, the emergence of a new type of coins with their own characteristics, known as cryptocurrencies, present additional challenges. In this sense, the paper seeks to understand up to what extent comments in social networks can capture the collective expectations of investors, and affect the future value of the currency. The objective is to predict the daily performance of a market based on two components: those that define the behavior of the cryptocurrency itself (volume, opening value, closing value, maximum value and minimum value) and the expectations and interactions of the environment, through the collected tweets. For this, the use of a type of recurrent neural network known as Long Short Term Memory (LSTM) is proposed. The methodology usedfor the preprocessing of the data and the application of this time series forecasting technique allows obtaining a prediction with a Mean Absolute Percent of 34.92%; This indicates that the representation of the perception variable in social networks has not been that relevant and, therefore, motivates new works for better representation using other NLP techniques.
更多查看译文
关键词
tweets,en
AI 理解论文
溯源树
样例
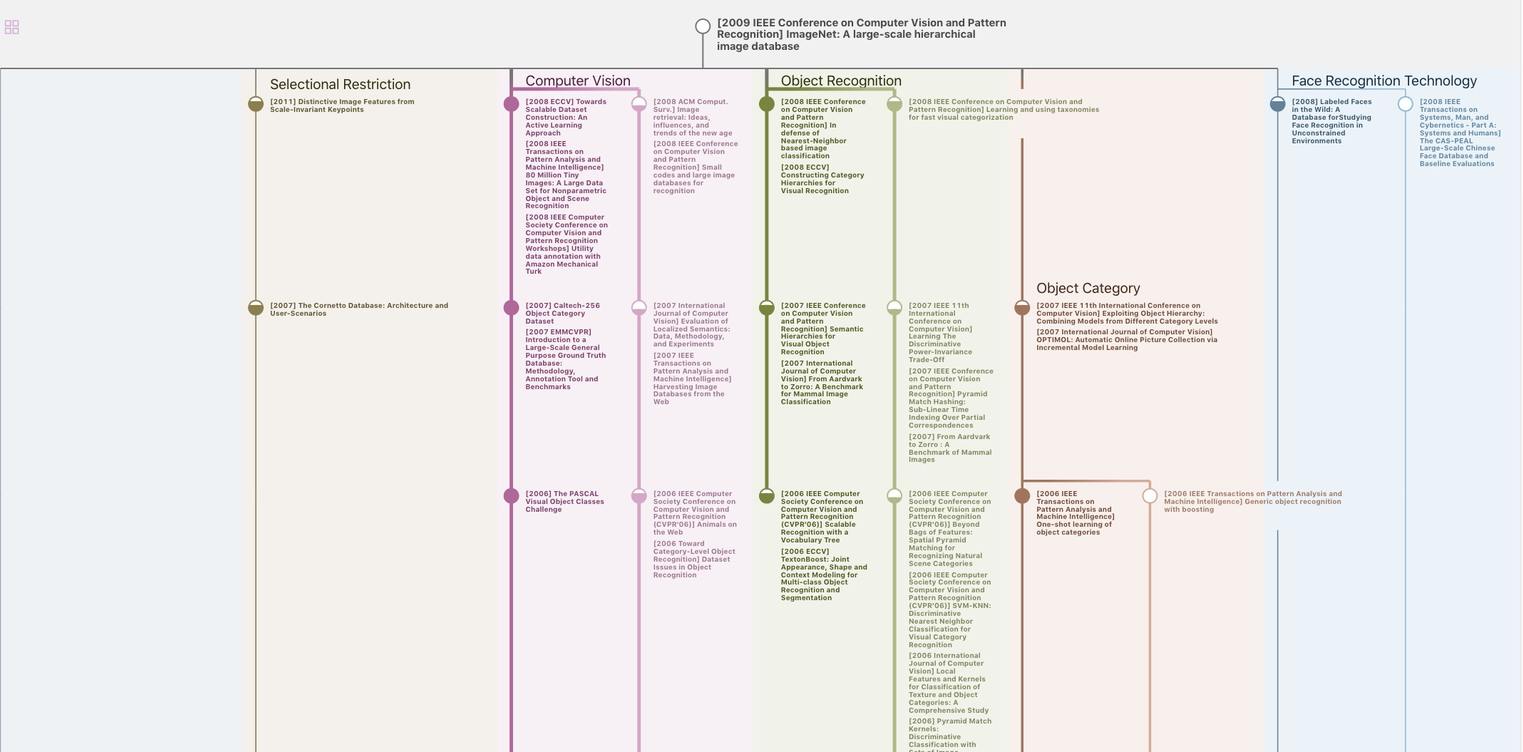
生成溯源树,研究论文发展脉络
Chat Paper
正在生成论文摘要