Deep Dynamic Scene Deblurring from Optical Flow.
IEEE transactions on circuits and systems for video technology(2022)
Abstract
Deblurring can not only provide visually more pleasant pictures and make photography more convenient, but also can improve the performance of objection detection as well as tracking. However, removing dynamic scene blur from images is a non-trivial task as it is difficult to model the non-uniform blur mathematically. Several methods first use single or multiple images to estimate optical flow (which is treated as an approximation of blur kernels) and then adopt non-blind deblurring algorithms to reconstruct the sharp images. However, these methods cannot be trained in an end-to-end manner and are usually computationally expensive. In this paper, we explore optical flow to remove dynamic scene blur by using the multi-scale spatially variant recurrent neural network (RNN). We utilize FlowNets to estimate optical flow from two consecutive images in different scales. The estimated optical flow provides the RNN weights in different scales so that the weights can better help RNNs to remove blur in the feature spaces. Finally, we develop a convolutional neural network (CNN) to restore the sharp images from the deblurred features. Both quantitatively and qualitatively evaluations on the benchmark datasets demonstrate that the proposed method performs favorably against state-of-the-art algorithms in terms of accuracy, speed, and model size.
MoreTranslated text
Key words
Deblurring,convolutional neural network,spatially variant recurrent neural network,optical flow
AI Read Science
Must-Reading Tree
Example
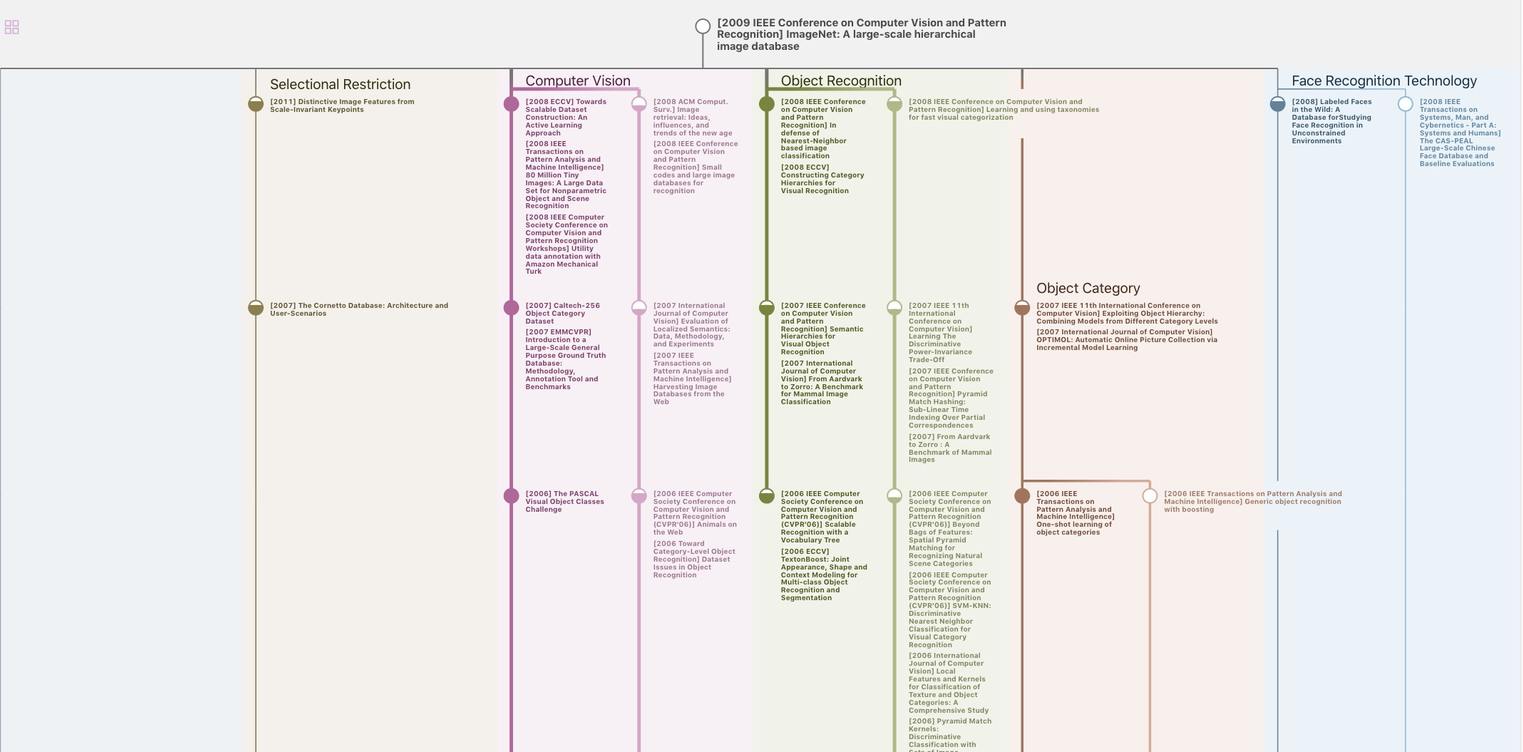
Generate MRT to find the research sequence of this paper
Chat Paper
Summary is being generated by the instructions you defined