A Comparison of Missing Data Handling Techniques
soft computing(2021)
Abstract
Missing data is a regular concern on data that professionals have to deal with. Efficient analysis techniques have to be followed to find interesting patterns. In this study, we are comparing 16 different imputation methods namely Linear, Index, Values, Nearest, Zero, slinear, Quadratic, Cubic, Barycentric, Krogh, Polynomial, Spline, Piecewise Polynomial, From derivatives, Pchip and Akima. These techniques are performed on real time UCI dataset and are under Missing Completely at a Random (MCAR) assumption, our result suggests the nearest, zero, quadratic and polynomial imputation methods which provides above 96% of accuracy when compared to the other techniques.
MoreTranslated text
Key words
Functional Data,Outlier Detection
AI Read Science
Must-Reading Tree
Example
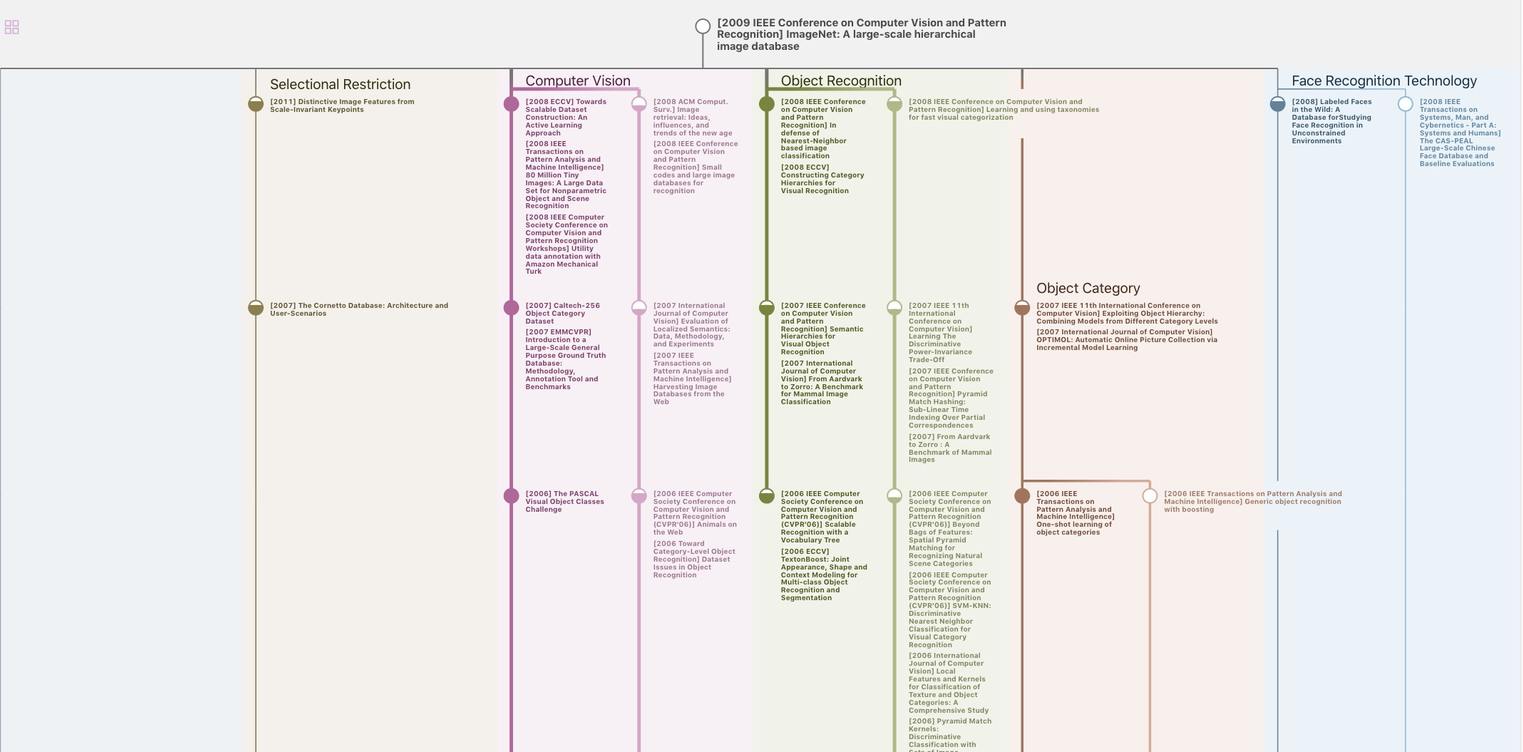
Generate MRT to find the research sequence of this paper
Chat Paper
Summary is being generated by the instructions you defined