Plants Stress Response Detection by Selecting Minimal Bands of Hyperspectral Images
2019 9th International Conference on Advances in Computing and Communication (ICACC)(2019)
摘要
It is an important task in the agricultural domain to determine the stress levels in plants. Drought conditions can have an adverse effect on crop yield. Hyperspectral Imaging (HSI) combined with classical Machine Learning algorithms are in current use to determine the stress levels. Every spectral band in an HSI does not contain useful information regarding the stress levels. For this reason, some vegetation indices are selected by agricultural researchers, based on reflectance ratios where a significant change in reflectance was observed because of stress. These indices do not always contain significant information because of changes in temperature, humidity or other atmospheric variations in different trials. There is no fixed set of vegetation indices which can be used to estimate stress levels accurately. In this paper, we demonstrated the working of Conditional Covariance Operator (CCM) which is used to select the most significant spectral bands from the collected Hyperspectral data itself. CCM is the most recent of the feature selection methods. This efficient feature selection method is used for the first time in this paper for plant stress analysis in rapid manner. It selects consistent discriminative spectral bands even when training examples per class are less than what other feature selection methods need. It can be seen that the Random Forest classifier model can classify the stress level into three categories (i) normal (ii) mild and (iii) severe stress with an accuracy of 99.7% when only 10 spectral bands are selected.
更多查看译文
关键词
Plant Phenotyping,Bands Selection,Hyperspectral Images (HSI),Conditional Co-variance Operator
AI 理解论文
溯源树
样例
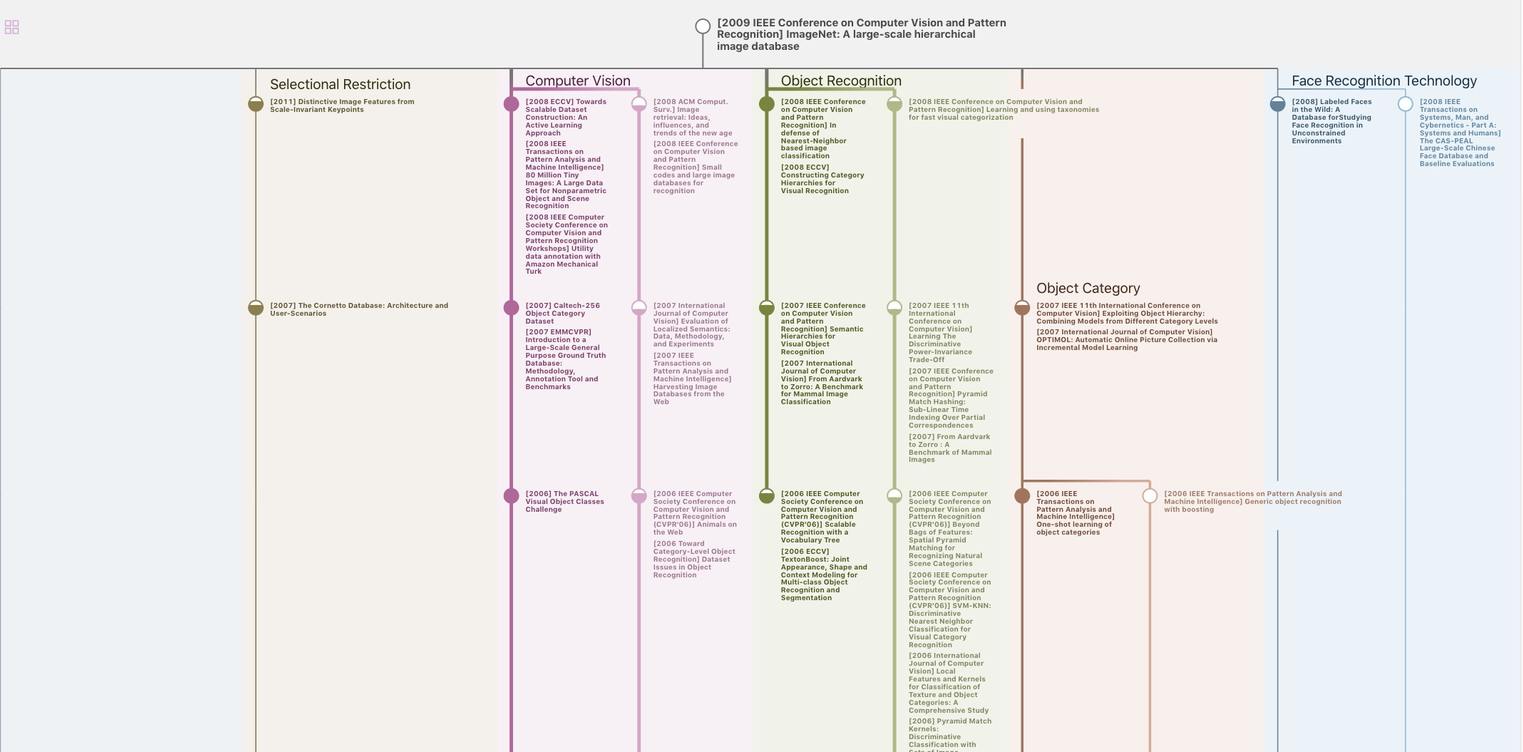
生成溯源树,研究论文发展脉络
Chat Paper
正在生成论文摘要