GS-RNN: A Novel RNN Optimization Method based on Vanishing Gradient Mitigation for HRRP Sequence Estimation and Recognition
2020 IEEE 3rd International Conference on Electronics Technology (ICET)(2020)
摘要
The High-Resolution Range Profile (HRRP) has attracted great concern in the field of Radar Automatic Target Recognition (RATR) for it contains abundant target structure information. However, single HRRP sample does not contain the spatiotemporal correlation between adjacent samples, which plays an important role in recognition, meanwhile, Recurrent Neural Network (RNN) is designed for sequence data specially, unfortunately, in practical application scenarios, RNN often shows the vanishing gradient problem. In this paper, we aim to address the two problems by proposing a Gradient Stabilized Recurrent Neural Network (GS-RNN) and applied it to HRRP sequence estimation and recognition. The GS-RNN can exploit the spatiotemporal correlation between adjacent HRRPs and avoid the vanishing gradient problem effectively. Three main procedures are included in our method, which are estimating the missing samples, training the proposed model and outputting the recognized result. Experiment results on the Moving and Stationary Target Acquisition and Recognition (MSTAR) dataset show the effectiveness of GS-RNN in sample estimation and recognition, additionally, the proposed method outperforms other traditional methods in low SNR, showing excellent anti-noise performance.
更多查看译文
关键词
high-resolution range profile sequence,radar automatic target recognition,recurrent neural network,sample estimation,vanishing gradient
AI 理解论文
溯源树
样例
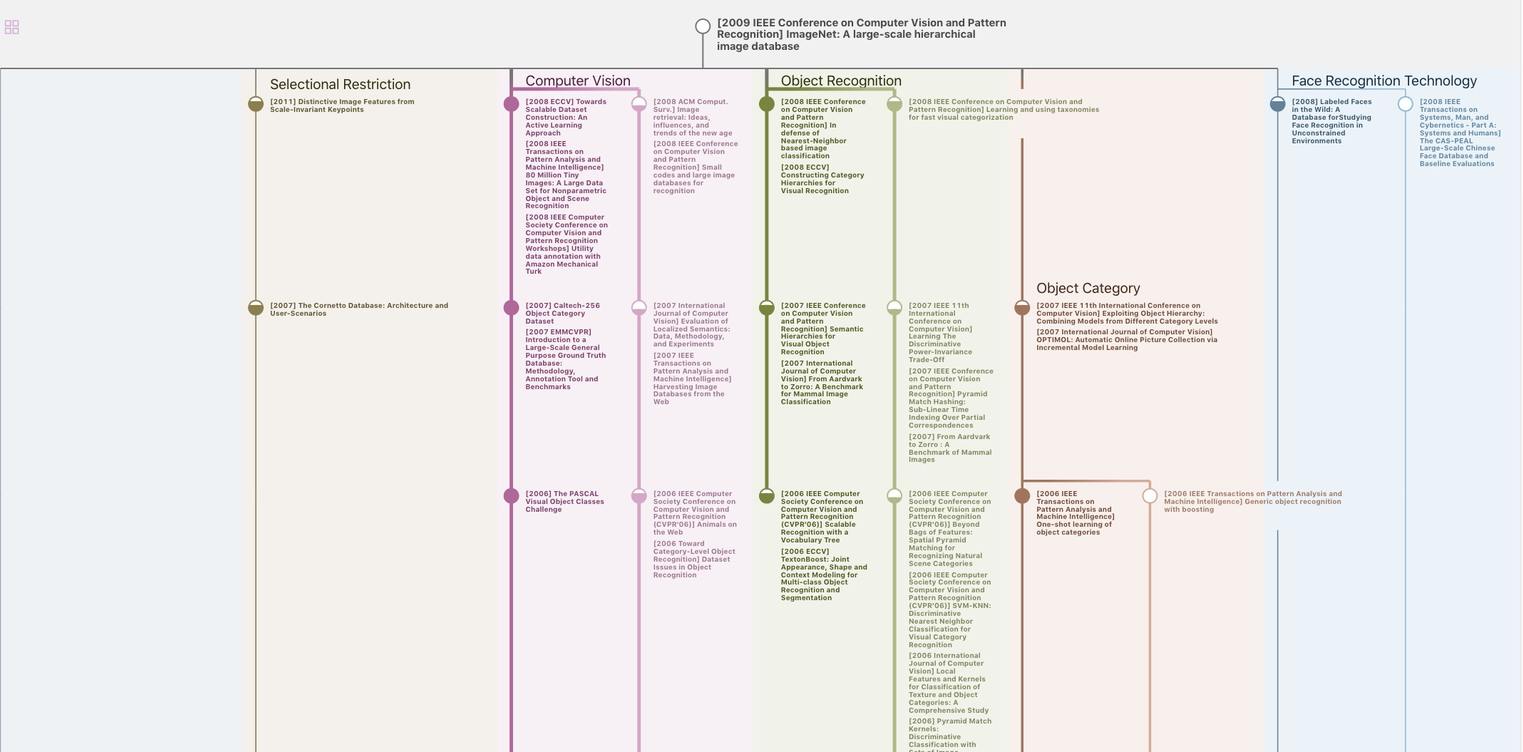
生成溯源树,研究论文发展脉络
Chat Paper
正在生成论文摘要