K-Means Bootstrap Analysis in the Birth Weight Classification of the Born Babies
International Journal of Advances in Scientific Research and Engineering (IJASRE), ISSN:2454-8006(2020)
摘要
Low birth weight babies are caused by poor nutritional status before and during pregnancy. The impact of low birth weight is the slowing of the growth of infants seen in weight gain that does not reach normal levels when one-year-old. Classification of birth weight babies will be grouped into several groups, namely, low birth weight, normal baby weight, and excess baby weight. Classification of birth weight is done by cluster analysis. Cluster analysis is one of the statistical analyzes which aims to group objects based on the similarity of characteristics between those objects. Clusters of K-Means begin by determining in advance the number of clusters desired, so the measure of inaccuracy must be measured again. The inaccurate size of the K-Means cluster analysis can be done with the application of Clustering Bootstrap. This study aims to classify the birth weight of a baby using a cluster and a bootstrap cluster. The results showed a grouping of birthweight babies grouped into three clusters, cluster I had a baby's birth weight above 3130.171 kilograms, cluster II had a baby's weight under 3033.327 kilograms and cluster III had a baby's birth weight below 2299.994 kilograms. K-Means Bootstrap results produce relatively the same cluster. The best method is K-Means Bootstrap B = 75, with a total accuracy rate (TAR) of 0.7993 and a total error rate (TER) of 0.2007. K-Means Bootstrap can be used as an alternative way to determine the group of birth weight babies.
更多查看译文
关键词
birth weight classification,born babies,k-means
AI 理解论文
溯源树
样例
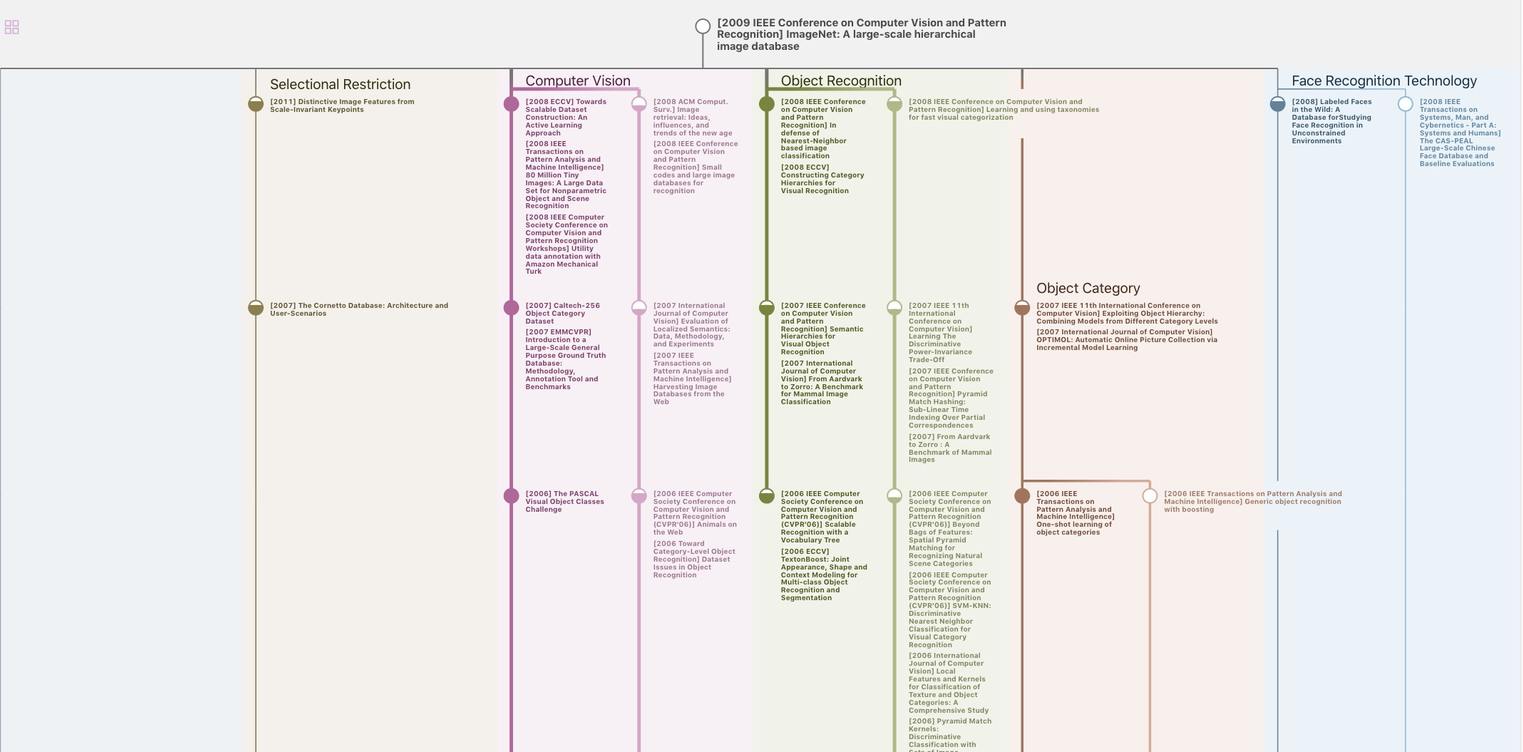
生成溯源树,研究论文发展脉络
Chat Paper
正在生成论文摘要