Generative adversarial network and transfer-learning-based fault detection for rotating machinery with imbalanced data condition
Measurement Science and Technology(2022)
摘要
Intelligent fault diagnosis achieves tremendous success in machine fault diagnosis because of its outstanding data-driven capability. However, the severely imbalanced dataset in practical scenarios of industrial rotating machinery is still a big challenge for the development of intelligent fault diagnosis methods. In this paper, we solve this issue by constructing a novel deep learning model incorporated with a transfer learning (TL) method based on the time-generative adversarial network (Time-GAN) and efficient-net models. Firstly, the proposed model, called Time-GAN-TL, extends the imbalanced fault diagnosis of rolling bearings using time-series GAN. Secondly, balanced vibration signals are converted into two-dimensional images for training and classification by implementing the efficient-net into the transfer learning method. Finally, the proposed method is validated using two types of rolling bearing experimental data. The high-precision diagnosis results of the transfer learning experiments and the comparison with other representative fault diagnosis classification methods reveal the efficiency, reliability, and generalization performance of the presented model.
更多查看译文
关键词
fault diagnosis, rotating machinery, imbalanced data, generative adversarial networks, transfer learning
AI 理解论文
溯源树
样例
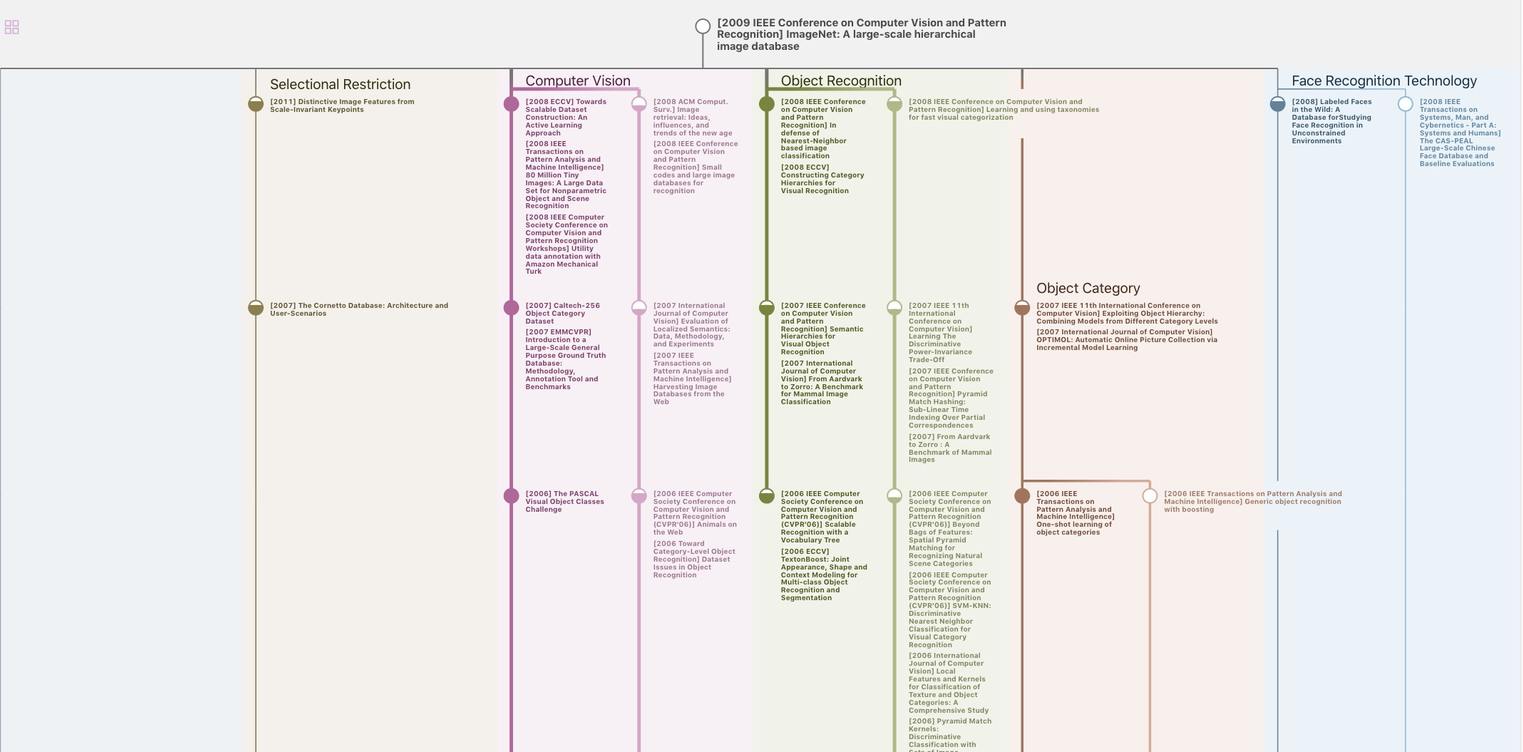
生成溯源树,研究论文发展脉络
Chat Paper
正在生成论文摘要