Off-Policy Training for Truncated TD(\(\lambda \)) Boosted Soft Actor-Critic
pacific rim international conference on artificial intelligence(2021)
摘要
TD(\(\lambda \)) has become a crucial algorithm of modern reinforcement learning (RL). By introducing the trace decay parameter \(\lambda \), TD(\(\lambda \)) elegantly unifies Monte Carlo methods (\(\lambda =1\)) and one-step temporal difference prediction (\(\lambda =0\)), which can learn the optimal value significantly faster than extreme cases with an intermediate value of \(\lambda \). However, it is mainly used in tabular or linear function approximation cases, which limits its practicality in large-scale learning and prevents it from adapting to modern deep RL methods. The main challenge of combining TD(\(\lambda \)) with deep RL methods is the “deadly triad” problem between function approximation, bootstrapping and off-policy learning. To address this issue, we explore a new deep multi-step RL method, called SAC(\(\lambda \)), to relieve this dilemma. Firstly, our method uses a new version of Soft Actor-Critic algorithm which stabilizes the learning of non-linear function approximation. Secondly, we introduce truncated TD(\(\lambda \)) to reduce the impact of bootstrapping. Thirdly, we further use importance sampling as the off-policy correction. And the time complexity of the training process can be reduced via parallel updates and parameter sharing. Our experimental results show that SAC(\(\lambda \)) can improve the training efficiency and the stability of off-policy learning. Our ablation study also shows the impact of changes in trace decay parameter \(\lambda \) and emerges some insights on how to choose an appropriate \(\lambda \).
更多查看译文
AI 理解论文
溯源树
样例
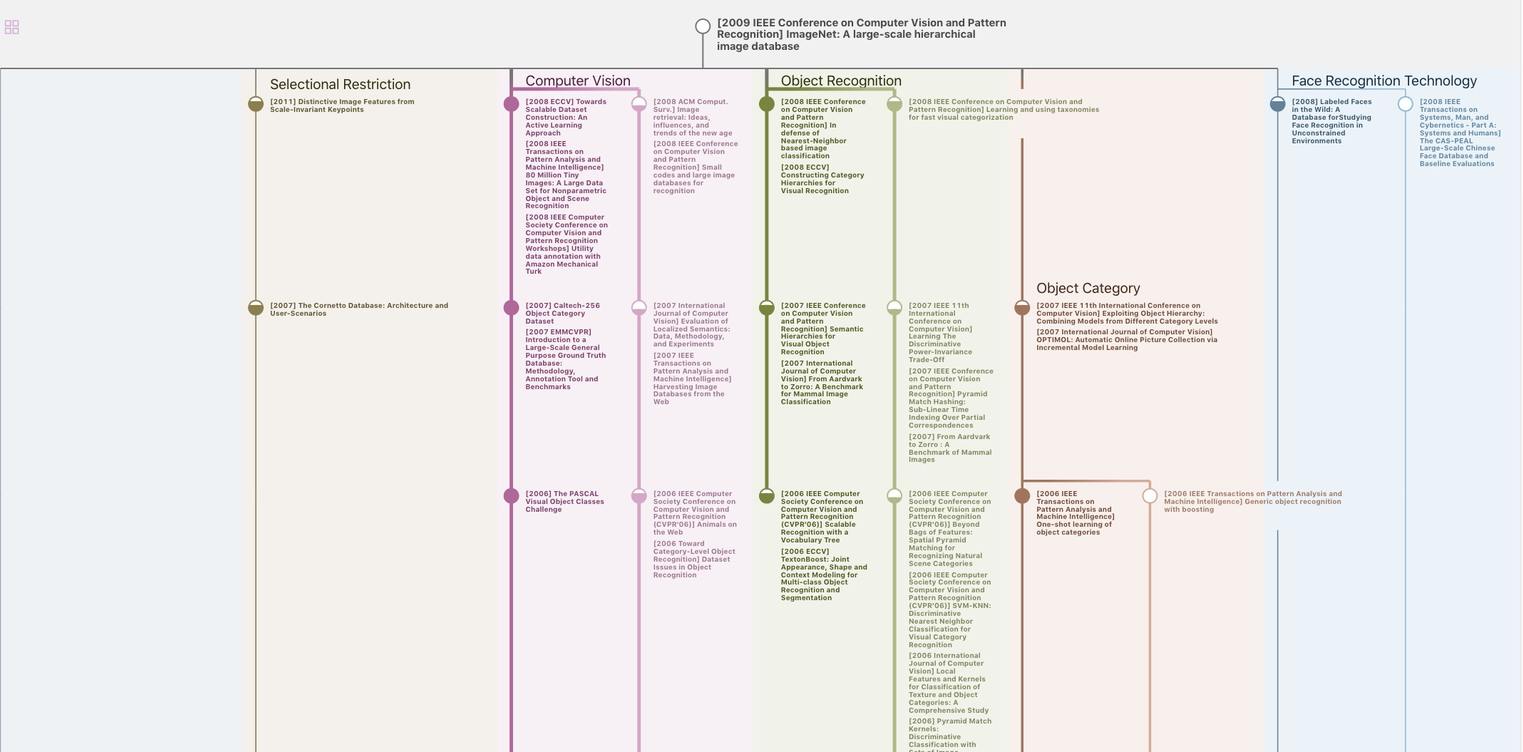
生成溯源树,研究论文发展脉络
Chat Paper
正在生成论文摘要