A Probabilistic Model for Discriminative and Neuro-Symbolic Semi-Supervised Learning
arXiv: Learning(2021)
Abstract
Strong progress has been achieved in semi-supervised learning (SSL) by combining several methods, some of which relate to properties of the data distribution p(x), others to the model outputs p(y|x), e.g. minimising the entropy of unlabelled predictions. Focusing on the latter, we fill a gap in the standard text by introducing a probabilistic model for discriminative semi-supervised learning, mirroring the classical generative model. Several SSL methods are theoretically explained by our model as inducing (approximate) strong priors over parameters of p(y|x). Applying this same probabilistic model to tasks in which labels represent binary attributes, we theoretically justify a family of neuro-symbolic SSL approaches, taking a step towards bridging the divide between statistical learning and logical reasoning.
MoreTranslated text
Key words
discriminative,learning,probabilistic model,neuro-symbolic,semi-supervised
AI Read Science
Must-Reading Tree
Example
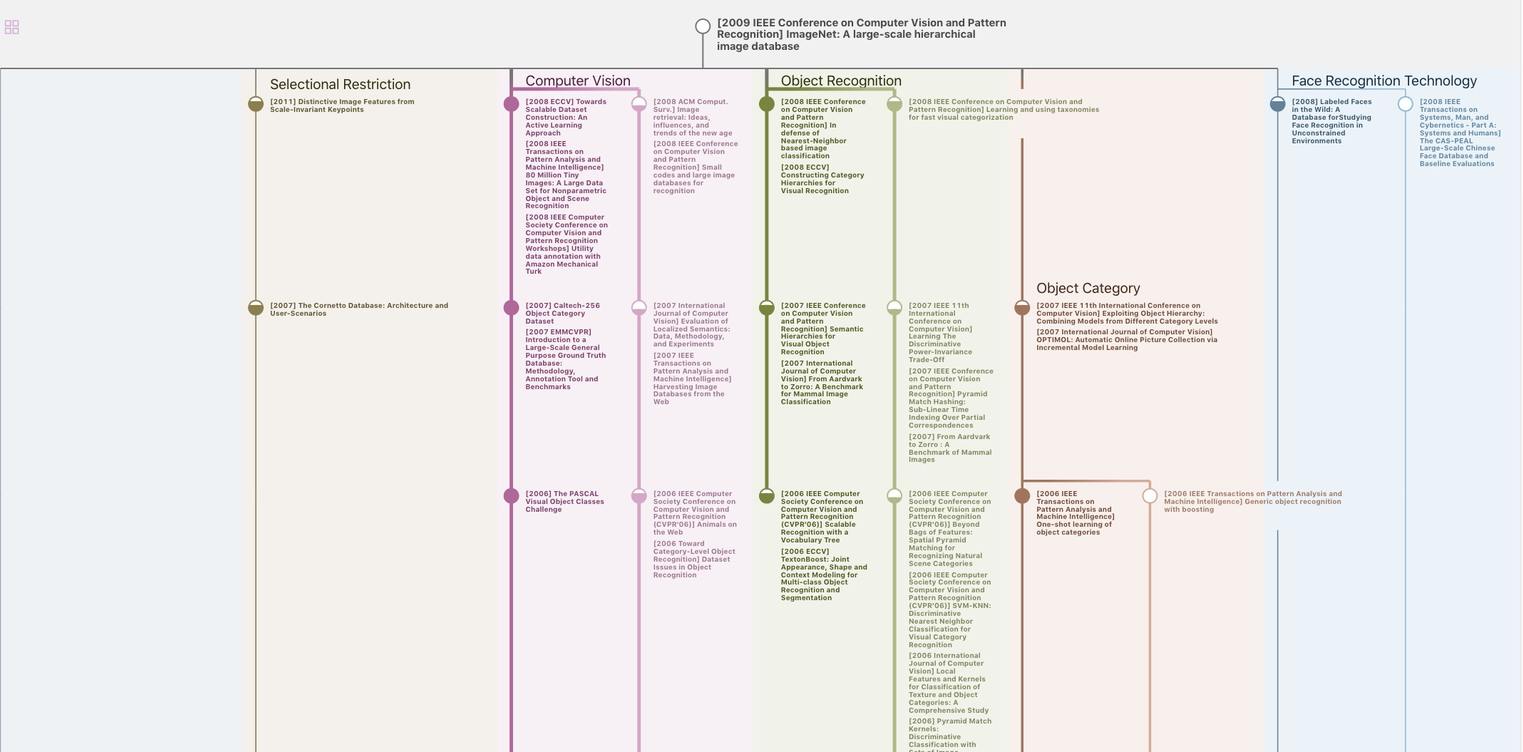
Generate MRT to find the research sequence of this paper
Chat Paper
Summary is being generated by the instructions you defined