Pandemic Lock-down, Isolation, and Exit Policies Based on Machine Learning Predictions
Social Science Research Network(2021)
摘要
The COVID-19 pandemic highlighted the criticality of research on pandemic management when medical solutions, such as vaccines are not available. We present a framework to combine a standard epidemiological SEIR model (susceptible-exposed-infected-removed) with equally standard machine learning classification models for clinical severity risk, defined by the risk of an individual needing intensive care (ICU) if infected. We then simulate isolation and exit policies using COVID-19 data and estimates for France as of spring 2020. We show that policies considering clinical risk predictions could relax isolation restrictions for millions of the lowest-risk population months faster while abiding to the ICU capacity at all times. Exit policies without risk predictions would exceed the ICU capacity by a multiple, or they should isolate a substantial portion of population for over a year to not overwhelm the medical system. Sensitivity analyses further decompose the impact of various elements of our models on the observed effects.
The main implication of our work is that predictive modelling, based on machine learning and artificial intelligence -- arguably, the main innovations of the last few decades, -- could bring significant value to managing pandemics. But for that, governments need to develop policies and invest in infrastructure to operationalize personalized isolation and exit policies based on risk predictions at scale. This involves health data policies to train predictive models and apply them to all residents, policies to support targeted resource allocation to maintain strict isolation for high risk individuals, and the likes.
更多查看译文
关键词
Epidemiology,Clinical Characteristics
AI 理解论文
溯源树
样例
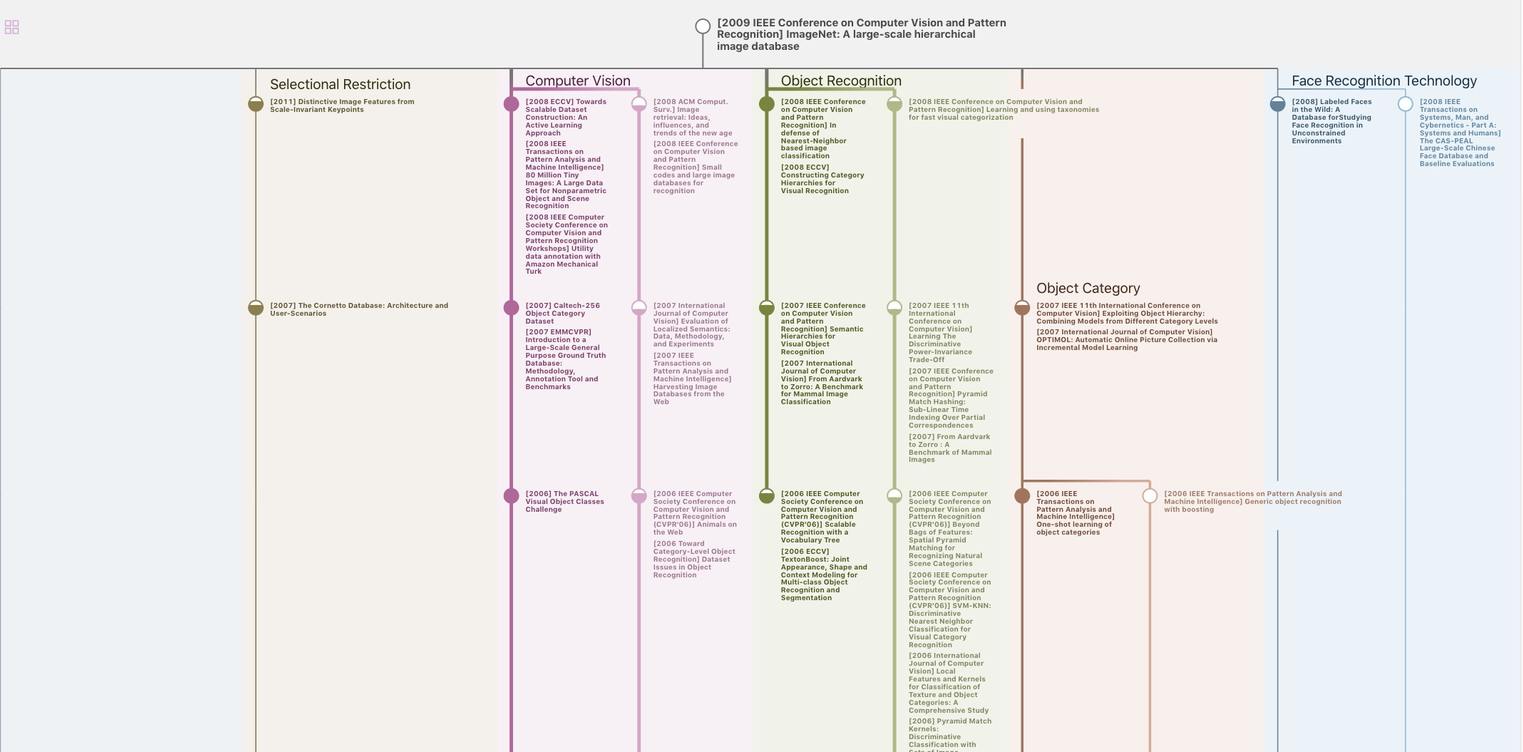
生成溯源树,研究论文发展脉络
Chat Paper
正在生成论文摘要