An Improved Convolutional Neural Network for Rolling Bearing Fault Diagnosis
ieee international conference on cyber technology in automation control and intelligent systems(2021)
Abstract
Aiming at the problems of low accuracy of the existing fault diagnosis methods for rolling bearings, an improved convolution neural network model for rolling bearing fault diagnosis is proposed. By training the sub-convolution neural network model (sub-CNN) for a single fault, the sub-CNN is combined into a sub-CNN group, and the transfer learning method is used to transfer to the combiner. The combiner is composed of several fully connected layers. The proposed neural network model solves the problem that the existing end-to-end neural network model is not accurate and improves the accuracy of fault diagnosis. The experiment results show that the classification accuracy of the improved convolution neural network is 99.95%, and the F1 value is 0.9966. Compared with existing models, the highest classification accuracy of the proposed model is improved by 11.4%, which is demonstrated that the proposed model is higher than the existing models. The superiority of our model is proved.
MoreTranslated text
Key words
Rolling Bearing,Fault Diagnosis,Convolutional Neural Network (CNN),Transfer Learning
AI Read Science
Must-Reading Tree
Example
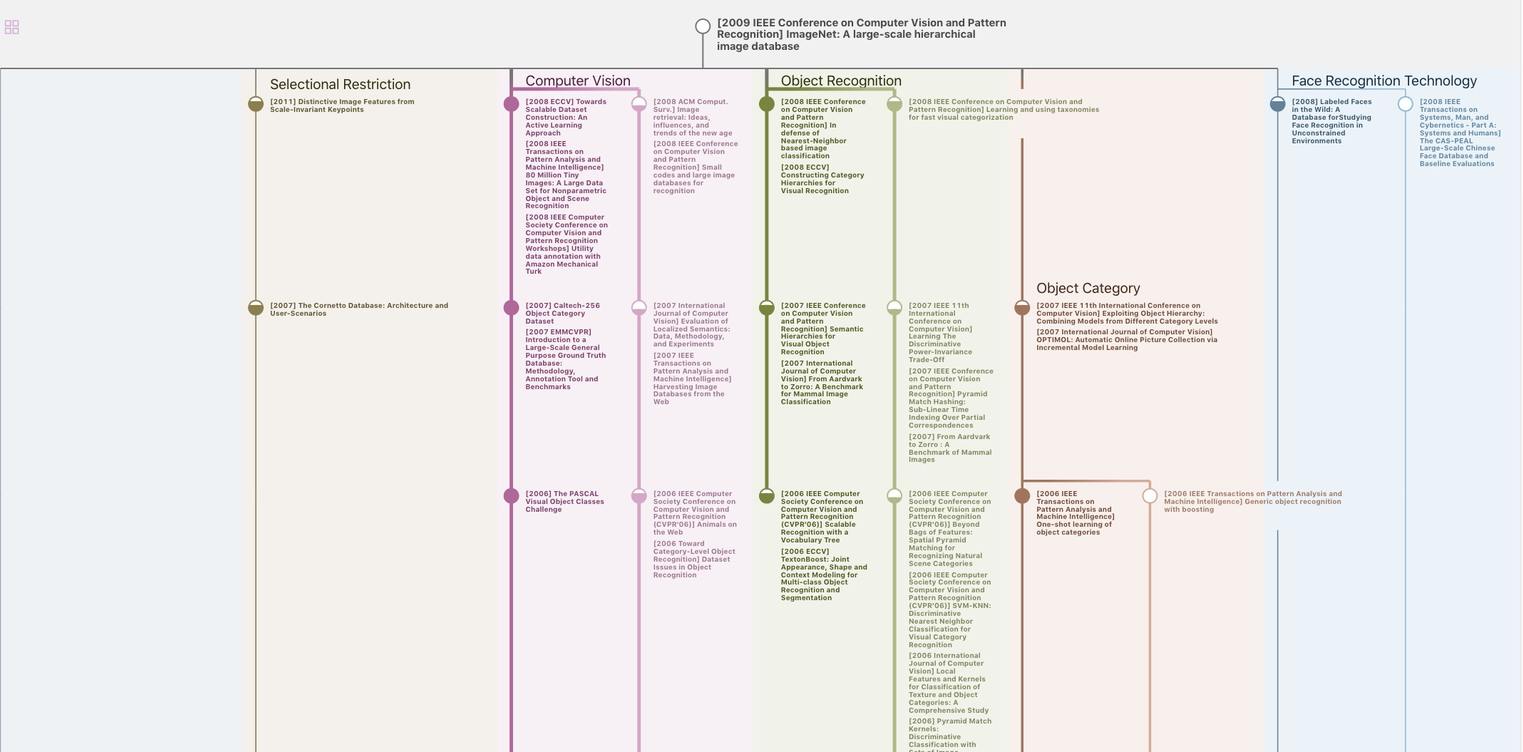
Generate MRT to find the research sequence of this paper
Chat Paper
Summary is being generated by the instructions you defined