An Efficient Learning Framework for Multi-Product Inventory Systems with Customer Choices
Social Science Research Network(2021)
摘要
In this paper, we first introduce a periodic-review multi-product inventory system where each customer's demand is affected by the product availabilities and the customer's preference. As customer preferences are not directly observable and hard to estimate, when the full distributional information of the demand is not available, the decision-maker has to learn the information on-the-fly, through the partial and censored feedback of customers. For this learning problem, if one ignores the inventory dynamic and simply treat this as a Multi-Armed Bandit problem and directly applies some existing algorithms, e.g., the Upper Confidence Bound (UCB) algorithm, the convergence can be extremely slow due to the high-dimensionality of the policy space. We propose a UCB-based learning framework that utilizes the demand information based on two improvement ideas. We illustrate how these two ideas can be incorporated by considering two specific systems: 1) multi-product inventory system with stock-out substitutions, 2) multi-product inventory assortment problem for urban warehouses. We develop improved UCB algorithms for both systems, using the two improvements. For both systems, the algorithm can achieve a tight worst-case convergence rate (up to a logarithmic term) on the planning horizon T. Extensive numerical experiments are conducted to demonstrate the efficiency of the improved UCB algorithms for the two systems. In the experiments, when there are more than 1000 candidate policies to choose from, the algorithms can achieve around 15% average expected regret within 50 periods and continues to steadily improve as time increases.
更多查看译文
关键词
demand censoring, inventory control, multiproduct, online learning
AI 理解论文
溯源树
样例
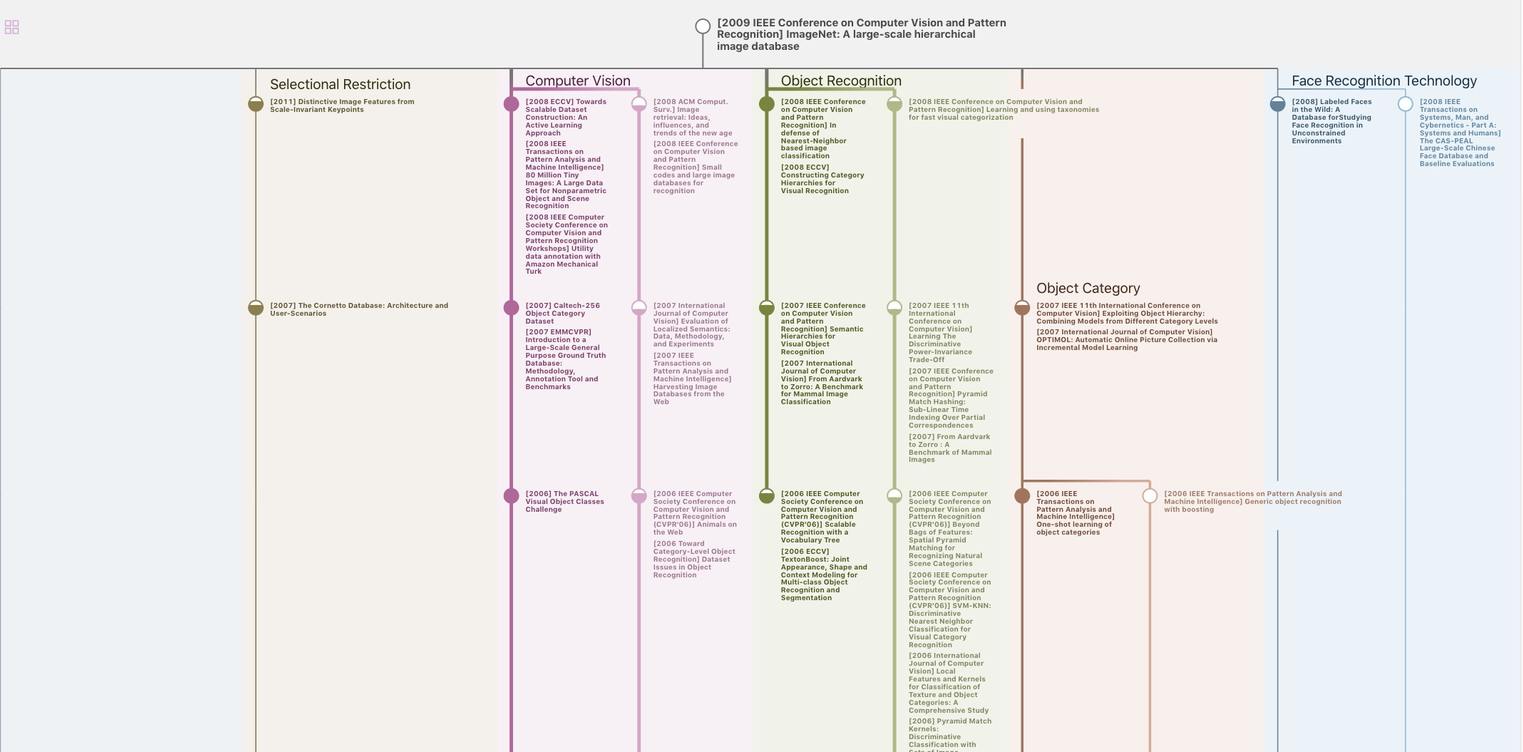
生成溯源树,研究论文发展脉络
Chat Paper
正在生成论文摘要