Dynamic Fusion of Electromyographic and Electroencephalographic Data towards Use in Robotic Prosthesis Control
Journal of Physics: Conference Series(2021)
摘要
We demonstrate improved performance in the classification of bioelectric data for use in systems such as robotic prosthesis control, by data fusion using low-cost electromyography (EMG) and electroencephalography (EEG) devices. Prosthetic limbs are typically controlled through EMG, and whilst there is a wealth of research into the use of EEG as part of a brain-computer interface (BCI) the cost of EEG equipment commonly prevents this approach from being adopted outside the lab. This study demonstrates as a proof-of-concept that multimodal classification can be achieved by using low-cost EMG and EEG devices in tandem, with statistical decision-level fusion, to a high degree of accuracy. We present multiple fusion methods, including those based on Jensen-Shannon divergence which had not previously been applied to this problem. We report accuracies of up to 99% when merging both signal modalities, improving on the best-case single-mode classification. We hence demonstrate the strengths of combining EMG and EEG in a multimodal classification system that could in future be leveraged as an alternative control mechanism for robotic prostheses.
更多查看译文
关键词
electroencephalographic data,dynamic fusion,prosthesis
AI 理解论文
溯源树
样例
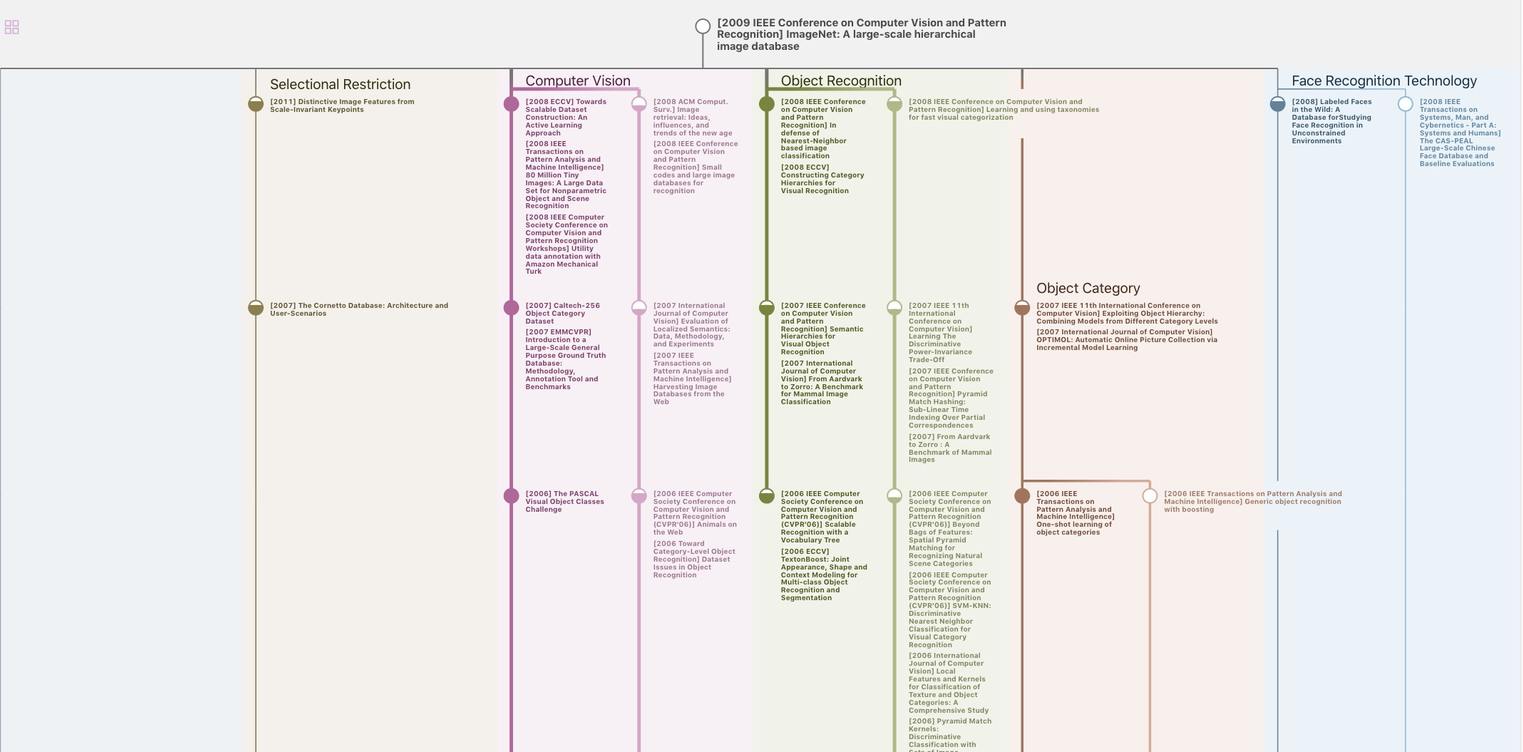
生成溯源树,研究论文发展脉络
Chat Paper
正在生成论文摘要