Solving Combinatorial Problems through Off-Policy Reinforcement Learning Methods
2020 International Conference on Electrical, Communication, and Computer Engineering (ICECCE)(2020)
摘要
In recent years, reinforcement learning (RL) has emerged as a strong candidate to solve many decision-making problems. It fundamentally combines the benefits of both deep neural networks and control engineering practices to accomplish human-level or even superior competency in decision-concise systems. This work extends this very idea and proves the applicability of RL algorithms to combinatorial puzzles such as river-crossing puzzle and seating arrangement problems. In that regard, this work applied quality-networks (QN), deep-quality-networks (DQN), and double-deep-quality-networks (DDQN) to environments mentioned above, and afterward, evaluated their performance through analyzing reward and performance plots. The study concludes that all these methods effectively completed the puzzle tasks with unnoticeable performance differences for the given environments.
更多查看译文
关键词
reinforcement learning,combinatorial optimization,puzzle-solving,deep neural networks
AI 理解论文
溯源树
样例
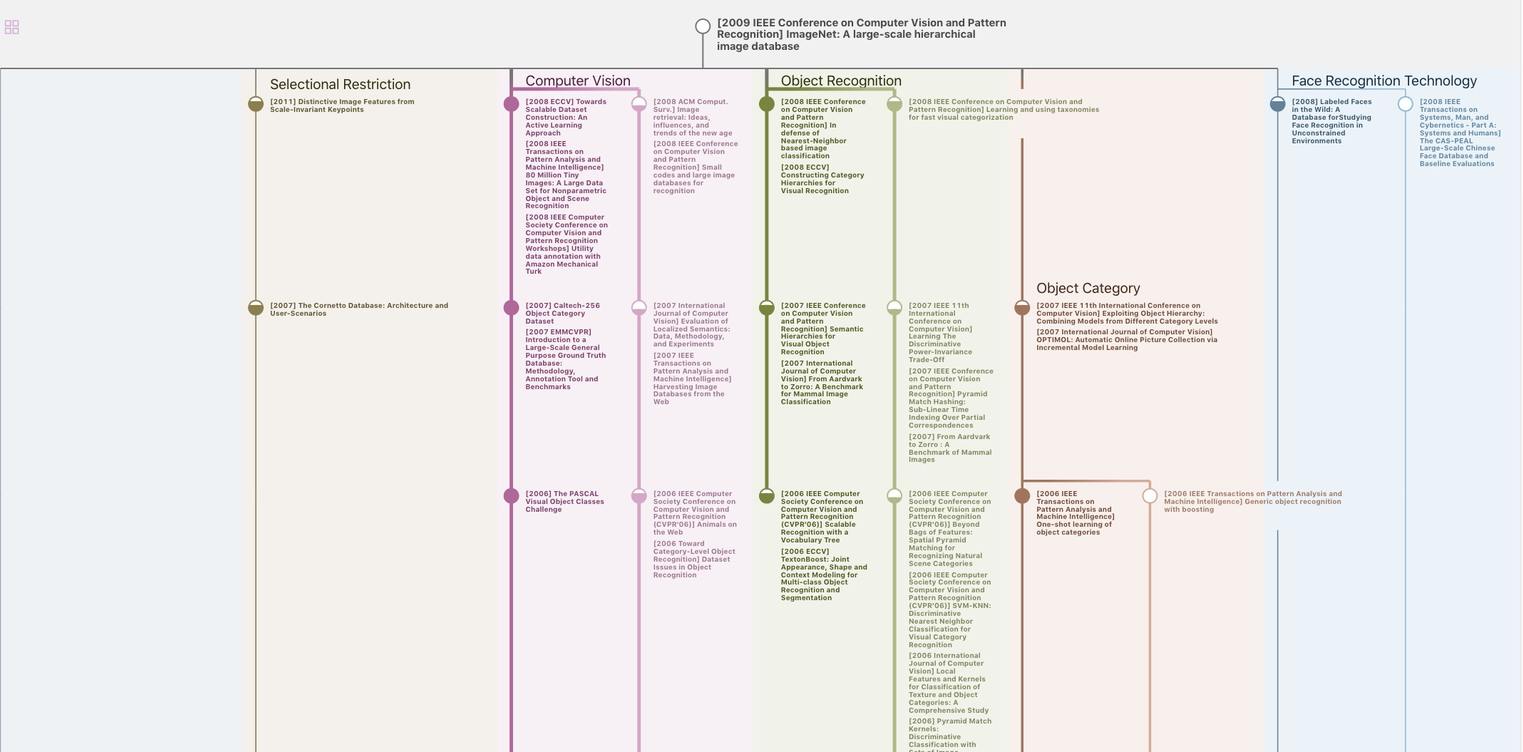
生成溯源树,研究论文发展脉络
Chat Paper
正在生成论文摘要