Data Augmentation of Sar Sensor Image via Information Maximizing Generative Adversarial Net
ieee international conference on electronic information and communication technology(2021)
Abstract
In practical applications, the SAR image dataset is always insufficient and lacks sample diversity. Generative adversarial nets(GANs) have been widely used to address the problem of insufficient data. However, owing to no restriction on input noise, the generated images are highly random and lack diversity. The paper proposes a data augmentation method based on the information maximizing GAN to generate samples purposefully. The proposed method is realized by using information-theoretic to extend the GAN, maximizing the mutual information between the observations and a fixed subset of noise variables. Finally, it finds out a highly semantic and meaningful hidden representation information in the SAR image and thus increases the sample diversity. The experiment results based on the MSTAD data illustrate the proposed method can effectively improve the robustness and accuracy of the classifier especially for the limited training dataset.
MoreTranslated text
Key words
data augmentation,Generative Adversarial Nets,SAR target recognition,convolutional neural networks
AI Read Science
Must-Reading Tree
Example
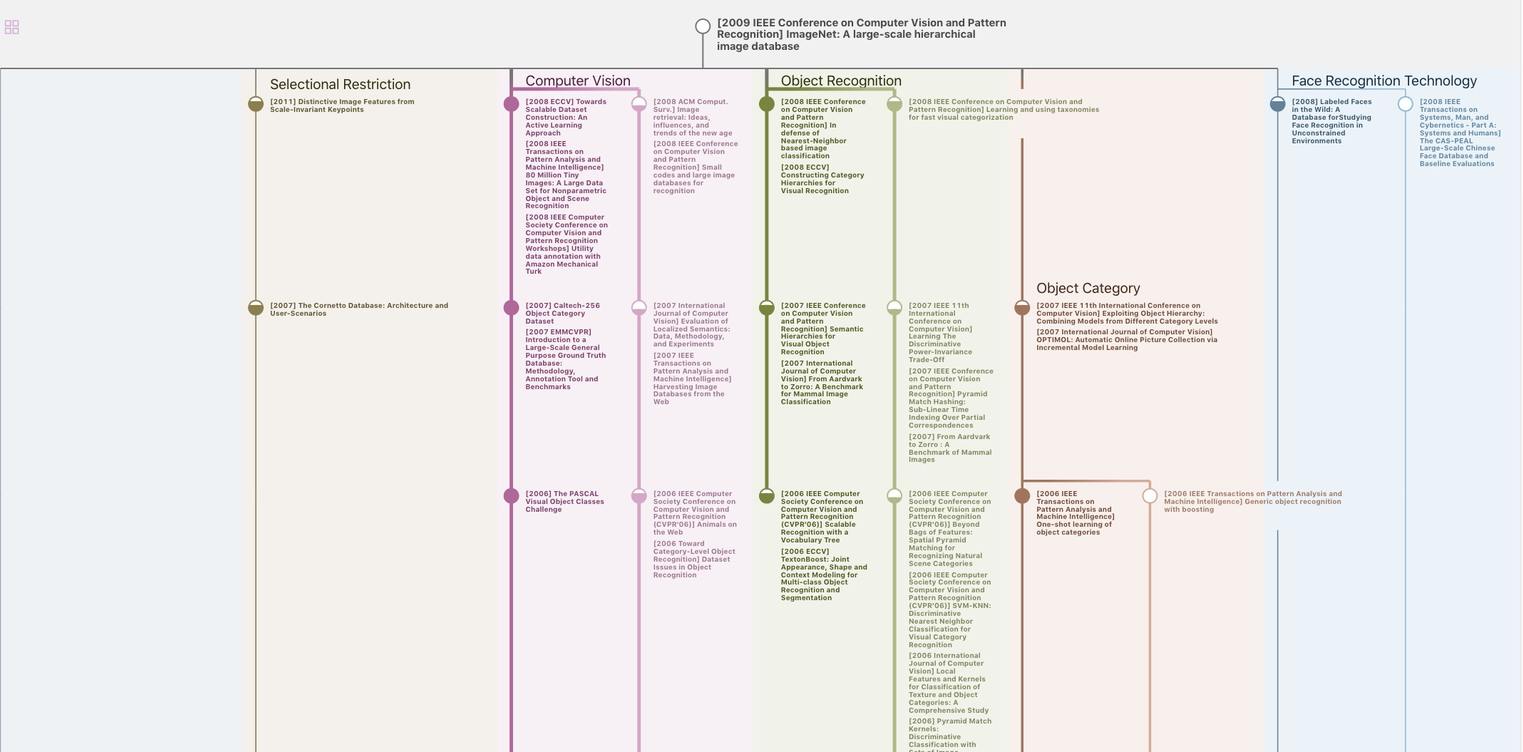
Generate MRT to find the research sequence of this paper
Chat Paper
Summary is being generated by the instructions you defined